A Learning-Based Method for Efficient Large-Scale Sensitivity Analysis and Tuning of Single Column Atmosphere Model (SCAM)
crossref(2022)
Abstract
. The Single Column Atmospheric Model (SCAM) is an essential tool for analyzing and improving the physics schemes of CAM. Although it already largely reduces the compute cost from a complete CAM, the exponentially-growing parameter space makes a combined analysis or tuning of multiple parameters difficult. In this paper, we propose a hybrid framework that combines parallel execution and a learning-based surrogate model, to support large-scale sensitivity analysis (SA) and tuning of combinations of multiple parameters. We start with a workflow (with modifications to the original SCAM) 5 to support the execution and assembly of a large number of sampling, sensitivity analysis, and tuning tasks. By reusing the 3,840 instances with the variation of 11 parameters, we train a neural network (NN) based surrogate model that achieves both accuracy and efficiency (with the computational cost reduced by several orders of magnitude). The improved balance between cost and accuracy enables us to integrate NN-based grid search into the traditional optimization methods to achieve better optimization results with fewer compute cycles. Using such a hybrid framework, we explore the joint sensitivity of 10 multi-parameter combinations to multiple cases using a set of three parameters, identify the most sensitive three-parameter combination out of eleven, and perform a tuning process that reduces the error of precipitation by 5% to 15% in different cases
MoreTranslated text
AI Read Science
Must-Reading Tree
Example
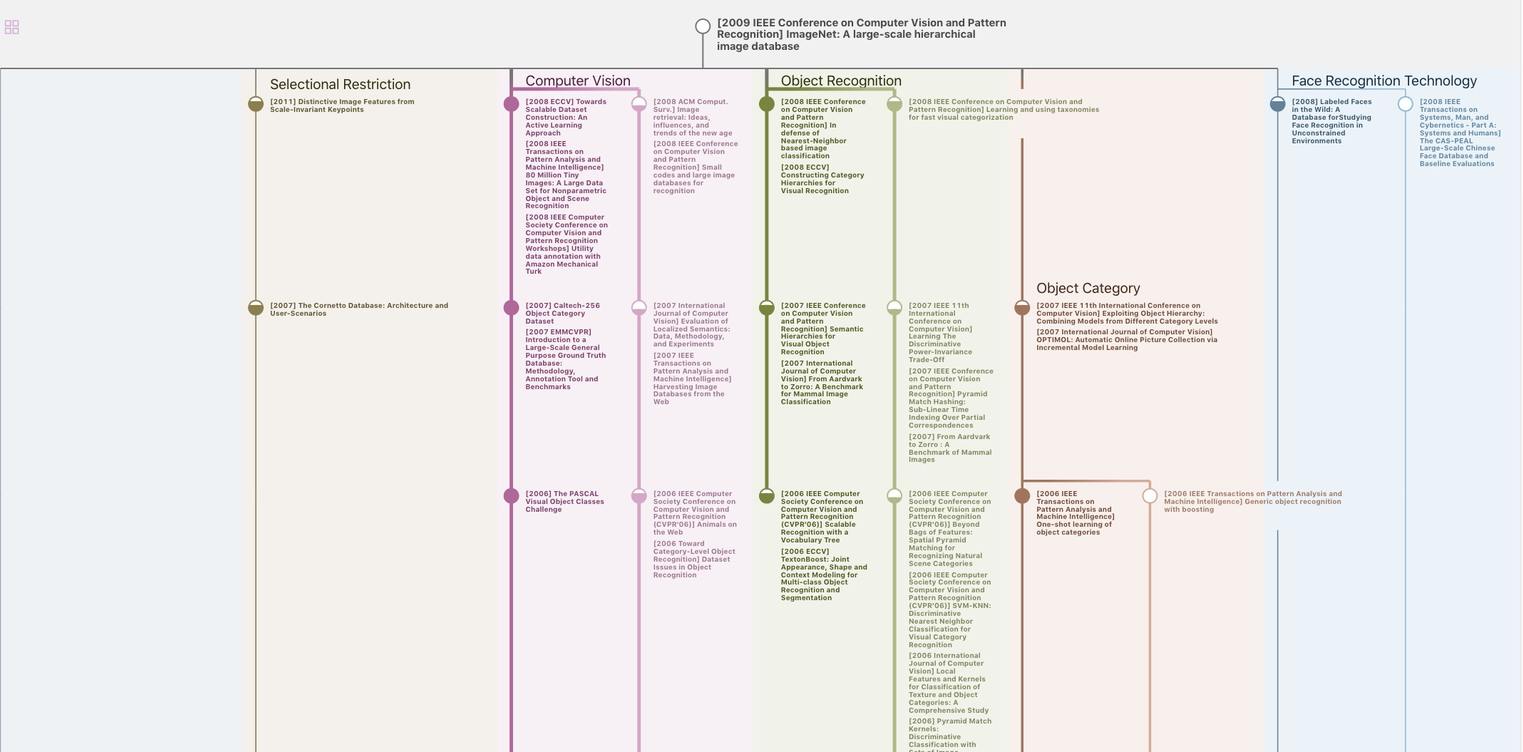
Generate MRT to find the research sequence of this paper
Chat Paper
Summary is being generated by the instructions you defined