Pseudo-Label Guided Sparse Deep Belief Network Learning Method for Fault Diagnosis of Radar Critical Components
IEEE Transactions on Instrumentation and Measurement(2023)
摘要
Effective fault diagnosis of critical components is essential to ensure the safe and reliable operation of the entire system. This article deals with the fault diagnosis of transmitter/receiver (T/R) module, which is a critical component in the phased array radar system, by proposing a novel deep belief network (DBN) learning method. A sparse DBN based on Gaussian function is first constructed to automatically learn the relationship between monitoring data and component health conditions. With the trained sparse DBN, the pseudo-labels are produced for unlabeled samples, while the information entropy is employed to calculate the confidence levels reflecting their certainty to reduce the effect of pseudo-label noise. The pseudo-labeled samples with high confidence levels are added to the training set to retrain the network. Optimal model configuration parameters are obtained through a chaos game optimization (CGO) algorithm. The effectiveness of the proposed method is verified on a real-world dataset from a certain type of phased array radar. The experiments show that the mean identification rate of this method can reach 96.33%, which not only exceeds some DBN-based modeling methods, but also exceeds other intelligent methods.
更多查看译文
关键词
Chaos game optimization (CGO),deep belief network (DBN),fault diagnosis,pseudo-labels,transmitter/receiver (T/R) module
AI 理解论文
溯源树
样例
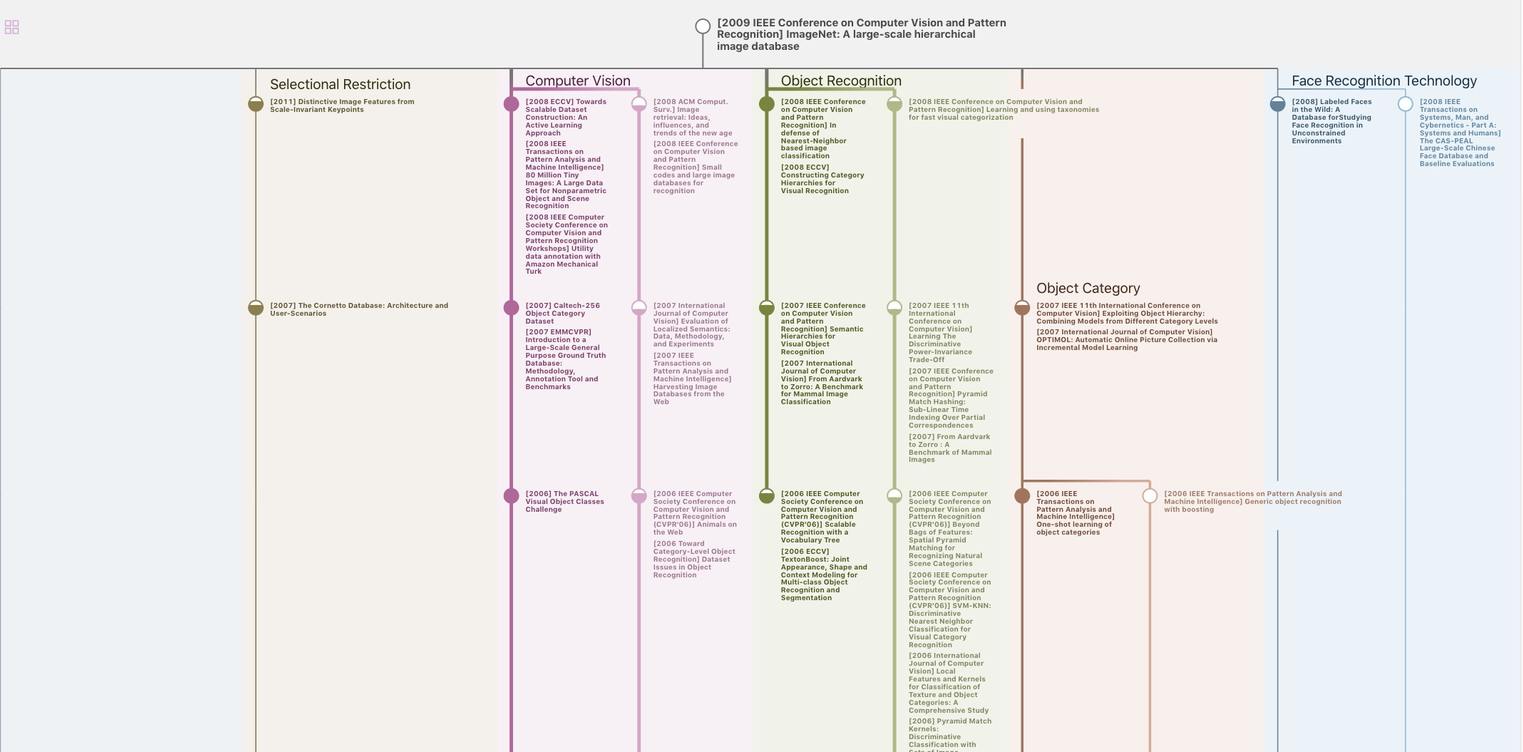
生成溯源树,研究论文发展脉络
Chat Paper
正在生成论文摘要