Optimal Scheduling of Integrated Energy System Based on Multi-agent Reinforcement Learning
2022 China Automation Congress (CAC)(2022)
摘要
The different interests pursued by each entity and the complex coupling relationship between multiple energy sources pose many challenges to the optimal operation of multi-park integrated energy systems(IESs). To this end, this paper proposes a data-driven based multi-agent proximal policy optimization (MAPPO) algorithm for the optimal scheduling of multi-park IESs. Firstly, the real-time optimal scheduling problem of multi-park IESs considering energy trading and carbon market trading is modeled as a Markov decision process (MDP). Secondly, the joint evaluation of MAPPO centralized training decentralized execution(CTDE) framework and global value function is used to achieve distributed autonomous learning for each agent to improve the quality of scheduling policies and privacy security of each park. The simulation results show that the proposed method has a faster convergence speed and can reduce the operating cost of the system under the influence of random fluctuations of new energy output and load.
更多查看译文
关键词
multi-park integrated energy systems,optimal scheduling,reinforcement learning,multi-agent proximal policy optimization
AI 理解论文
溯源树
样例
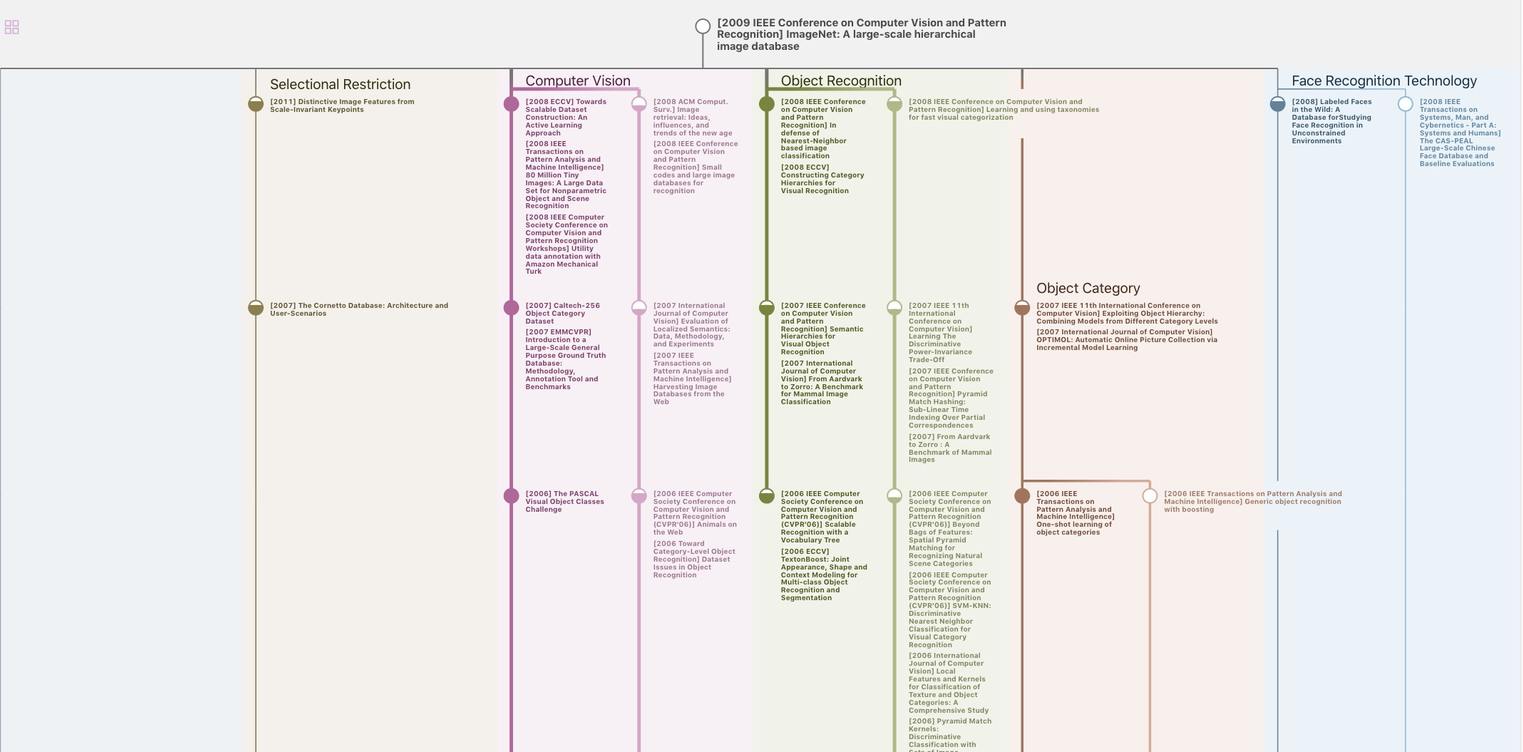
生成溯源树,研究论文发展脉络
Chat Paper
正在生成论文摘要