Is Your Anomaly Detector Ready for Change? Adapting AIOps Solutions to the Real World
International Conference on AI Engineering - Software Engineering for AI(2023)
Abstract
Anomaly detection techniques are essential in automating the monitoring of IT
systems and operations. These techniques imply that machine learning algorithms
are trained on operational data corresponding to a specific period of time and
that they are continuously evaluated on newly emerging data. Operational data
is constantly changing over time, which affects the performance of deployed
anomaly detection models. Therefore, continuous model maintenance is required
to preserve the performance of anomaly detectors over time. In this work, we
analyze two different anomaly detection model maintenance techniques in terms
of the model update frequency, namely blind model retraining and informed model
retraining. We further investigate the effects of updating the model by
retraining it on all the available data (full-history approach) and only the
newest data (sliding window approach). Moreover, we investigate whether a data
change monitoring tool is capable of determining when the anomaly detection
model needs to be updated through retraining.
MoreTranslated text
Key words
anomaly detection,AIOps,model monitoring,model maintenance,concept drift detection
AI Read Science
Must-Reading Tree
Example
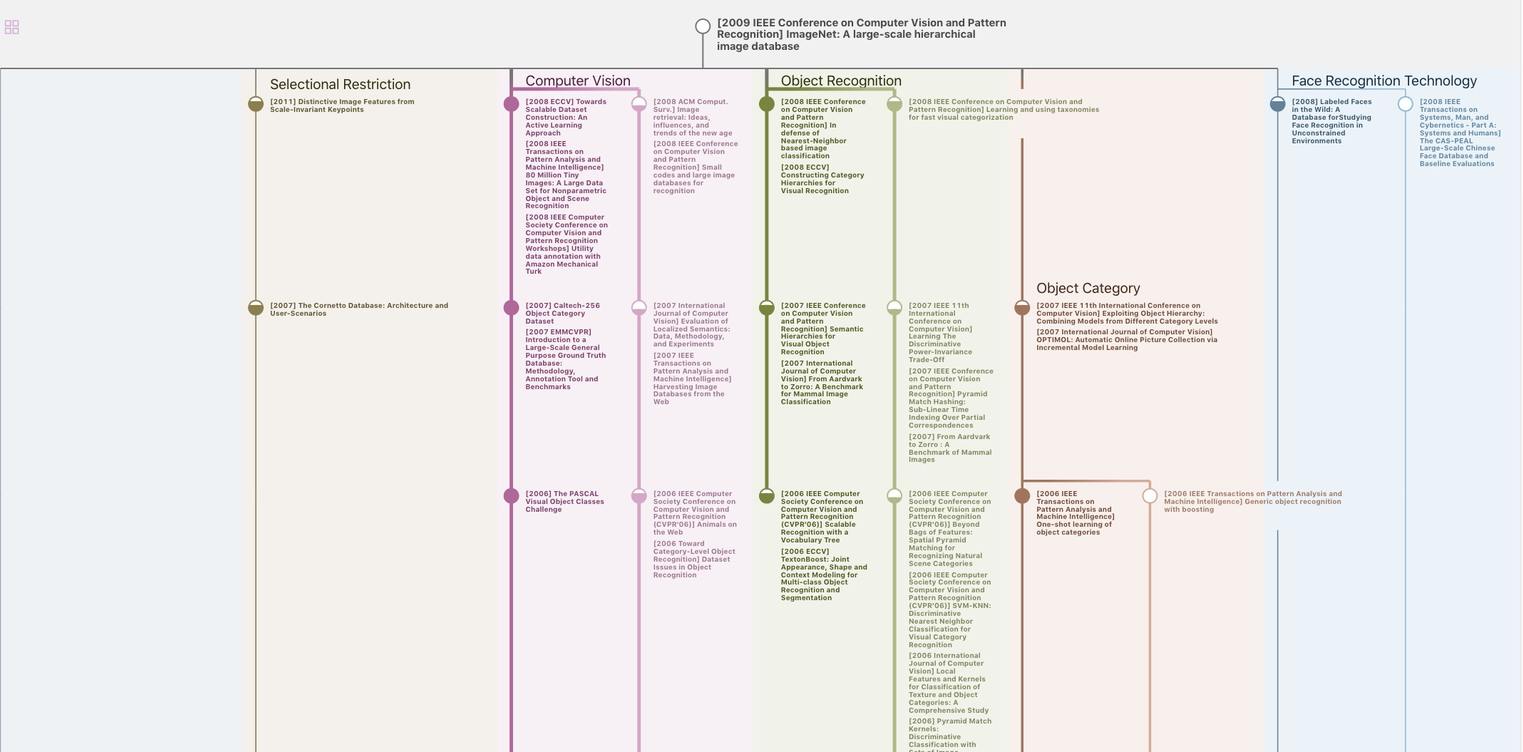
Generate MRT to find the research sequence of this paper
Chat Paper
Summary is being generated by the instructions you defined