Recursive Sparse Bayesian Learning
2022 China Automation Congress (CAC)(2022)
摘要
While sparse Bayesian learning (SBL) has attracted a great amount of attention in a wide range of areas, its practical utility is limited due to the high computational cost. To address this issue, we propose a recursive SBL algorithm in this paper, which reduces the computational complexity per iteration from $\mathcal{O}\left( {{n^3}} \right)$ to $\mathcal{O}\left( {{n^2}} \right)$. First, based on the Kalman filter, we derive the posterior distribution of model parameters recursively by dealing with the input-output data in turn. More specifically, the posterior distribution of model parameters of the previous iteration will be regarded as its prior distribution of the current iteration and then combined with the data likelihood to derive its posterior distribution of the current iteration, resulting in a Kalman filter like updating. Second, for unknown hyperparameters that were introduced for imposing the sparsity-promoting prior on model parameters, we also develop a computationally efficient method to estimate their values. Lastly, we demonstrate the effectiveness of our method on several numerical experiments. Compared with other methods, our method has a competitive performance with a very low computational cost.
更多查看译文
关键词
Sparse Bayesian learning,Kalman filter,Recursive algorithm
AI 理解论文
溯源树
样例
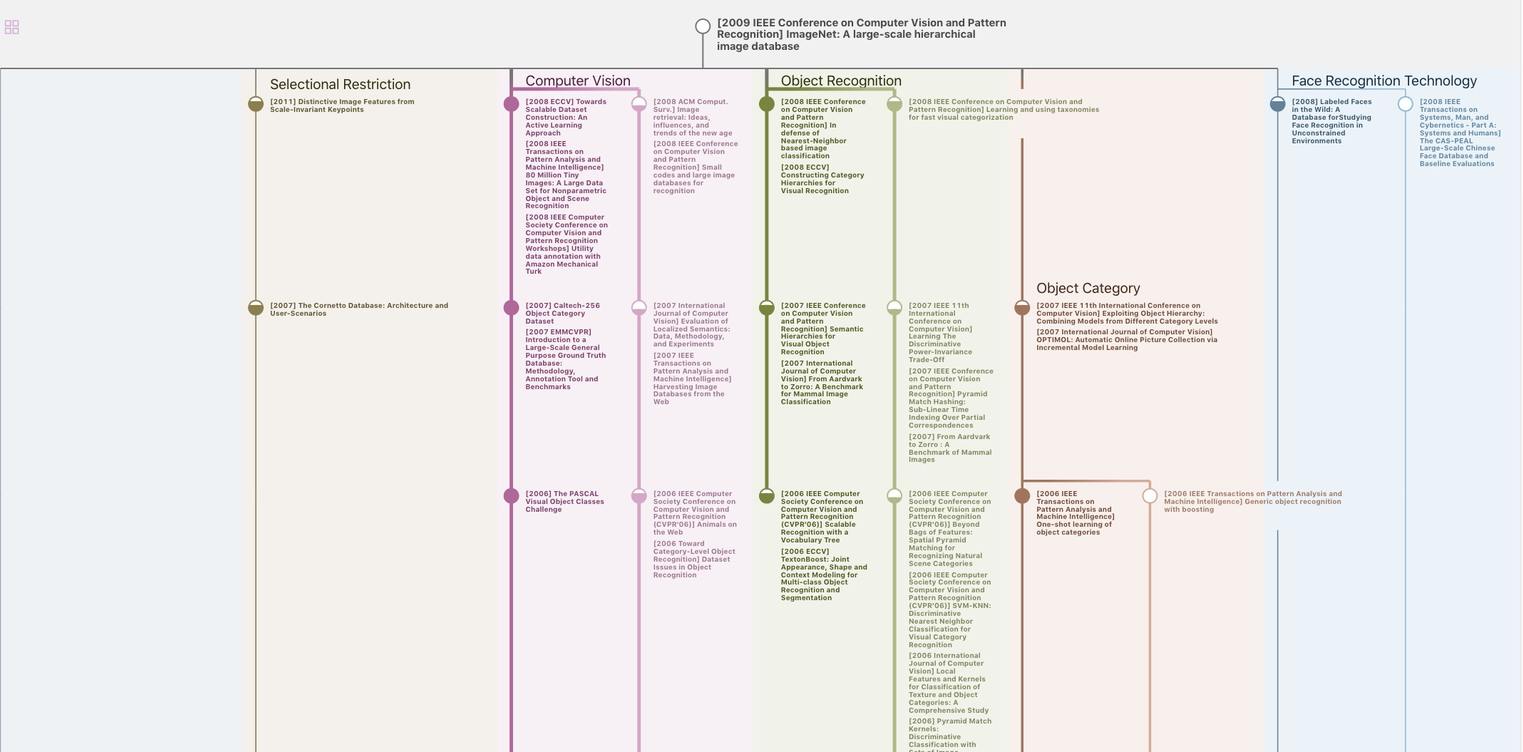
生成溯源树,研究论文发展脉络
Chat Paper
正在生成论文摘要