Defect detection for PV Modules based on the improved YOLOv5s
2022 China Automation Congress (CAC)(2022)
Abstract
Cracks and fragments are common defects of photovoltaic (PV) modules, both of which can lead to severe degradation of PV power generation. Traditional fault diagnosis methods are difficult to accurately locate the defect regions in PV modules. In this paper, a developed YOLOv5s is proposed to achieve cracks and fragments detection of PV modules in the electroluminescent (EL) images. More Specific, a defect image dataset is constructed based on image preprocessing and data augmentation on 402 EL images. And a novel cross stage partial operation unit named C3_cbam and a novel spatial pyramidal pooling operation unit named SPP_eca are proposed respectively by combining with visual attention mechanism. Among which, C3_cbam is presented to develop C3_1 unit in YOLOv5s to help the network focus on critical regions in the image by incorporating spatial and channel information. SPP_eca is the improvement of SPP unit by enhancing channel attention to reduce the aliasing effects. The experiment results illustrate that the proposed algorithm achieves 0.923 mAP on the constructed defect image dataset, which has an accuracy increment of 3.3% compared with the original YOLOv5s algorithm. The algorithm also performs well compared to other state-of-art defect detection algorithms.
MoreTranslated text
Key words
defect detection,PV modules,deep neural network
AI Read Science
Must-Reading Tree
Example
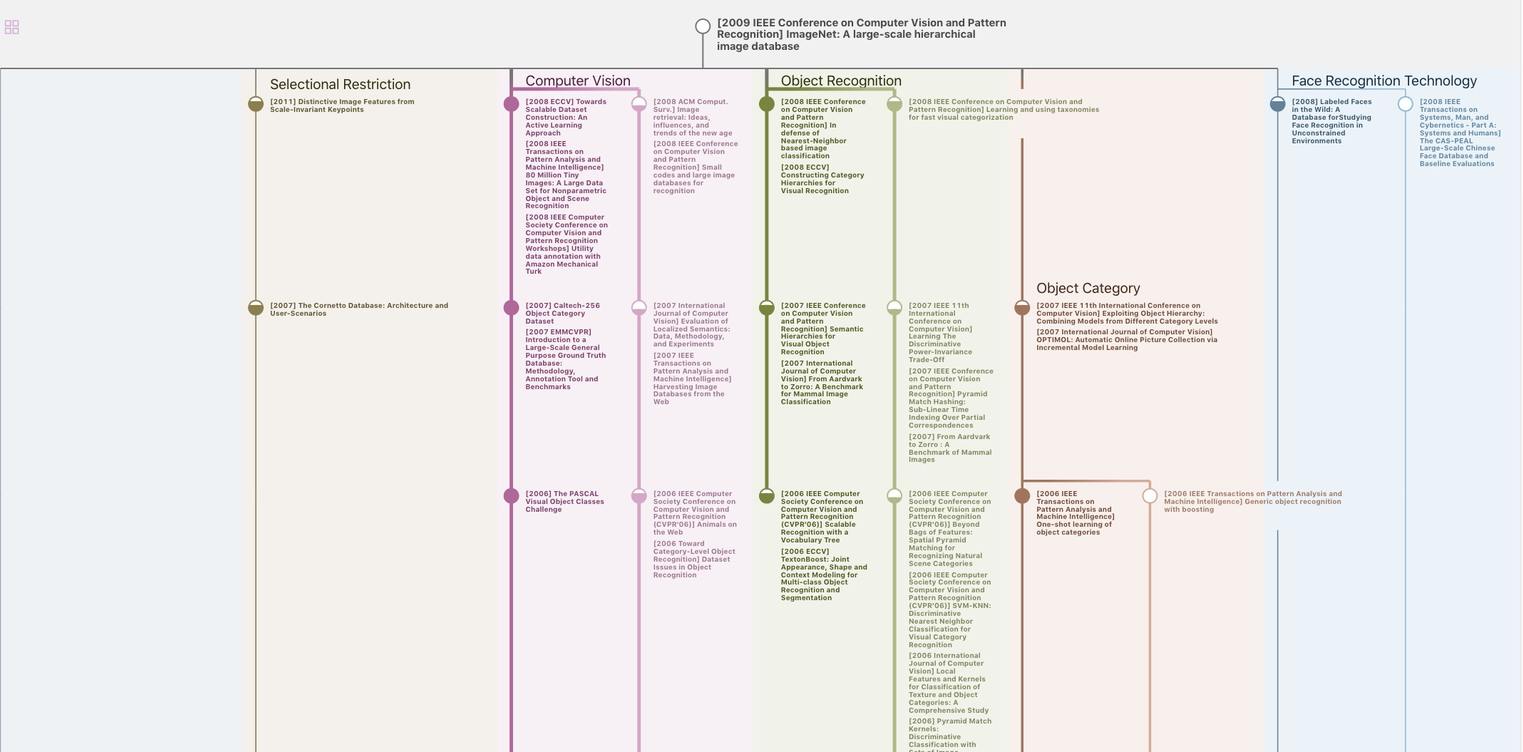
Generate MRT to find the research sequence of this paper
Chat Paper
Summary is being generated by the instructions you defined