Few-shot gearbox fault diagnosed based on meta-learning and time convolution network
2022 China Automation Congress (CAC)(2022)
摘要
In order to resolve the problem that the traditional fault diagnosis methods can not accurately diagnose under complex working conditions and few fault data, a fault diagnosis method based on meta-learning and time convolution network (TCN) is proposed in this paper. Firstly, Ensemble Empirical Mode Decomposition-Independent Component Analysis (EEMDICA) is used to denoise the original signals under different working conditions, and the acquired signals are randomly sampled to form meta tasks according to the meta-learning protocol. Through the TCN model training, the priori knowledge is obtained by optimizing the initialization parameters. The knowledge is used to improve accuracy in unforeseen condition tasks. Finally, simulation experiments are carried out on the gearbox dataset. The results indicate that the method has advantages in solving the fault classification problem of few shot gearbox under complex working conditions.
更多查看译文
关键词
Few-shot,Meta-learning,Gearbox fault diagnosis,Complex working conditions
AI 理解论文
溯源树
样例
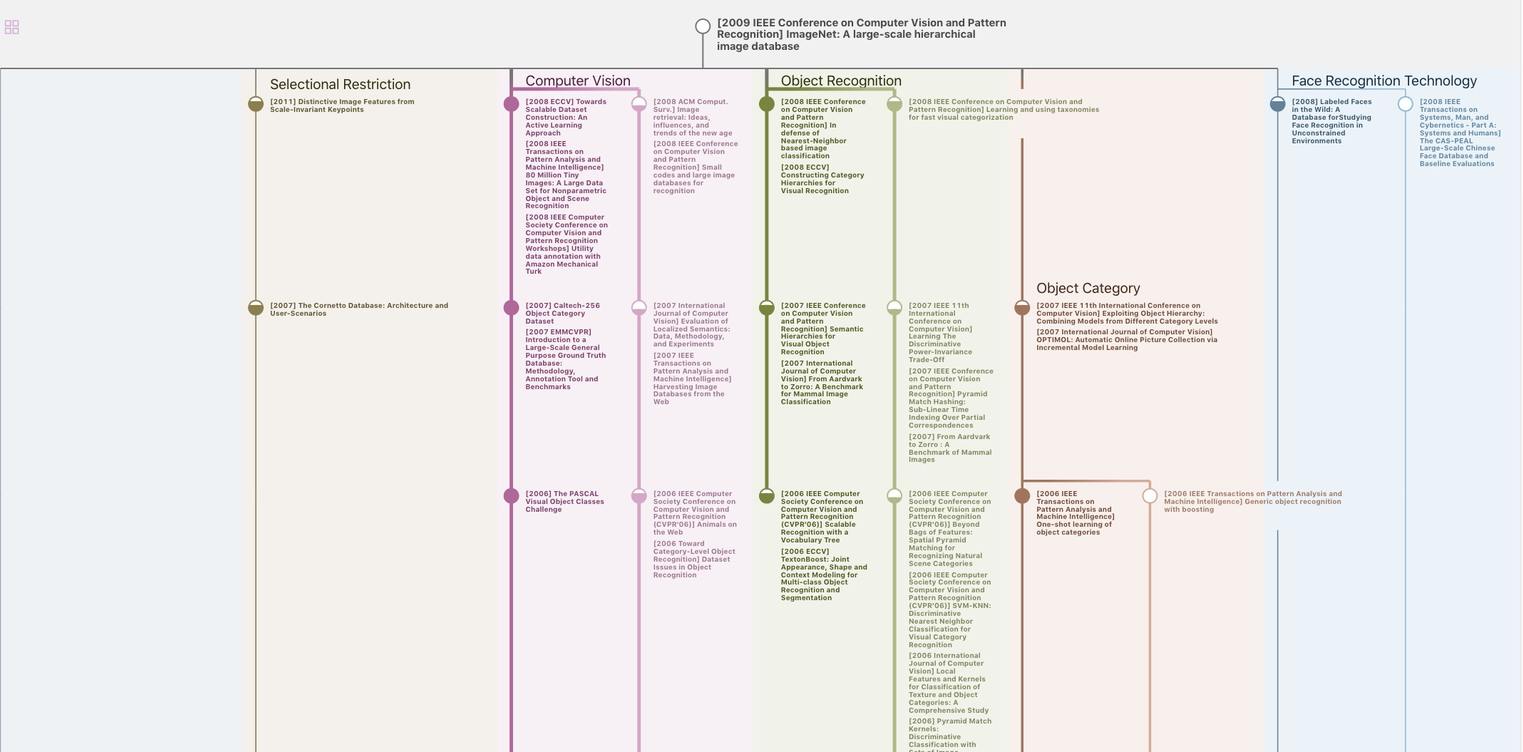
生成溯源树,研究论文发展脉络
Chat Paper
正在生成论文摘要