Accuracy of fully automated, AI-generated models compared with validated clinical model to predict post-operative glomerular filtration rate after renal surgery.
Journal of Clinical Oncology(2023)
摘要
693 Background: The American Urologic Association (AUA) recommends estimation of the postoperative glomerular filtration rate (GFR) in patients with a renal mass to help decide between partial nephrectomy (PN) or radical nephrectomy (RN). If postoperative GFR<45 mL/min/1.73m2, a PN should be prioritized. Most existing methods to predict postoperative GFR are rarely implemented in the clinical setting due to complexity. Previously validated models based on clinical equations or kidney volumes from hand-segmented or semi-automated segmentations are quite accurate but have seen limited uptake in clinical practice. We hypothesize that we could develop an artificial intelligence (AI)-GFR prediction that would be calculated automatically on a preoperative computed tomography (CT) scan and predict a postoperative GFR as accurately as a validated clinical model. Methods: 300 patients undergoing PN or RN for renal tumor from the 2021Kidney and Kidney Tumor Segmentation Challenge(KiTS21) were analyzed. We excluded 7 patients having bilateral tumors. Preoperative GFR was the closest recorded value preoperatively and postoperative GFR≥90 days postoperatively. Split-renal-function (SRF) was determined in a fully automated way from preoperative imaging and our previously developed deep learning segmentation model. We programmed the algorithm to estimate postoperative GFR as 1.24×preoperative GFR×contralateral SRF for RN; and as 89% of the preoperative GFR for PN. We compared AI-predicted GFR to a validated clinical model (GFR=35+preoperative GFR(x0.65)-18(if radical nephrectomy)-age(x0.25)+3(if tumor size >7 cm)-2 (if diabetes)). We compared the AI and clinical model estimations of GFR to the measured postoperative GFR using correlation coefficients (R) and compared the ability of AI models to predict a postoperative GFR<45 using logistic regression and AUCs. Results: In 293 patients, the median age was 60 years ((IQR) 51-68), 40.6% were female, and 62.1% had PN. The median tumor size was 4.2 (2.6-6.1), and 91.8% of the tumors were malignant, of which 35.1% were high-grade, 25.6% were high-stage, and 21.8% had necrosis. The median R.E.N.A.L. nephrometry score was 8 (7-9). When comparing measured postoperative GFR, the correlation coefficients were 0.75 and 0.77 for the AI model and clinical models, respectively. For the prediction of a postoperative GFR< 45 ml/min/1.73m2, the AI and clinical models performed similarly (AUC of 0.89 and 0.9, respectively). Conclusions: Our study demonstrates the feasibility of a fully automated prediction of postoperative GFR based on CT imaging and baseline GFR with comparable predictive accuracy to existing validated clinical prediction models. These AI-generated predictions can be implemented for decision-making, with no clinical details, clinician time, or measurements needed.
更多查看译文
关键词
renal surgery,clinical models,ai-generated,post-operative
AI 理解论文
溯源树
样例
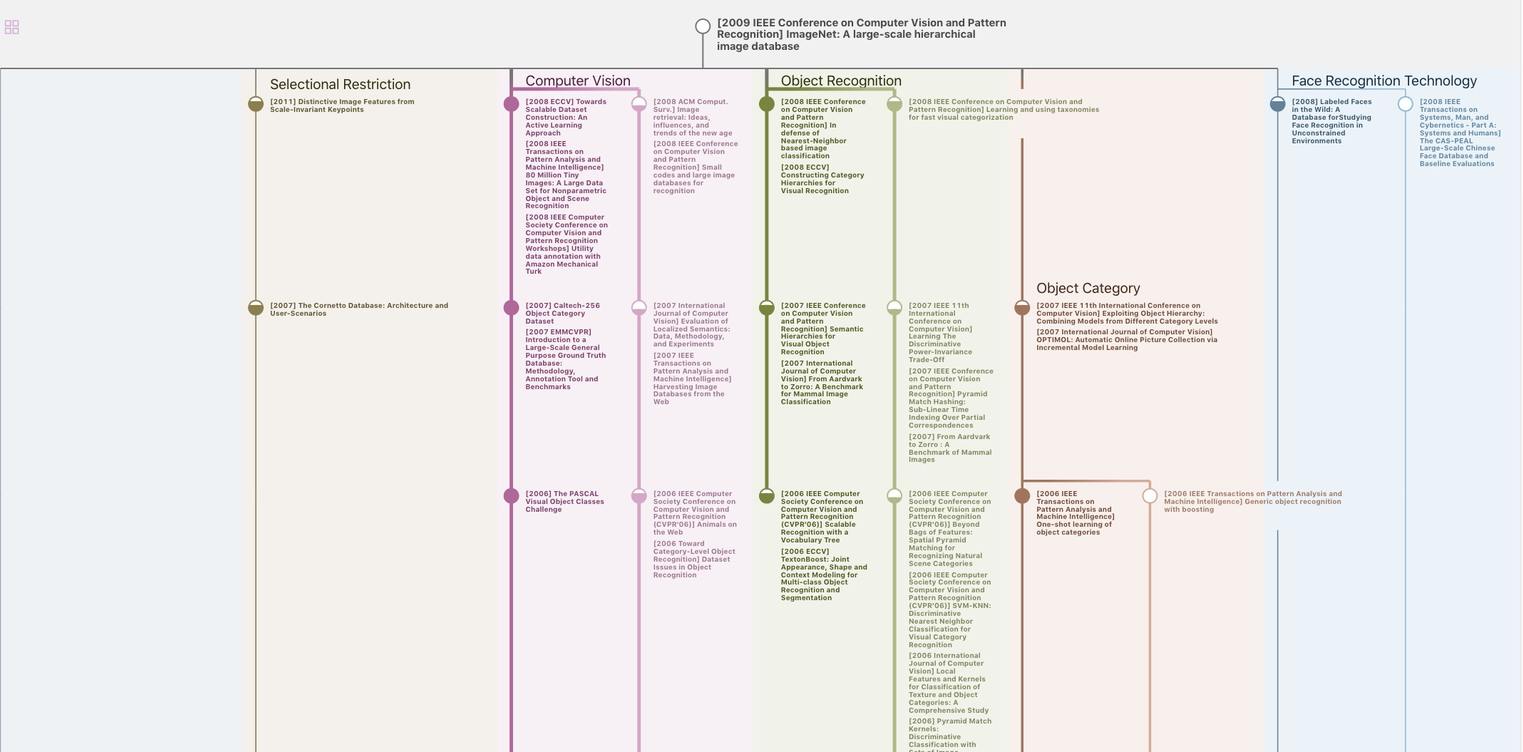
生成溯源树,研究论文发展脉络
Chat Paper
正在生成论文摘要