Mechanical Fault Diagnosis With Noisy Multisource Signals via Unified Pinball Loss Intuitionistic Fuzzy Support Tensor Machine
IEEE TRANSACTIONS ON INDUSTRIAL INFORMATICS(2024)
摘要
In this article, a challenging and significant intelligent fault diagnosis task is investigated, in which multisource sensor signals with intense noise and outlier disturbances are jointly analyzed. Such a scenario has hardly been considered in industrial research. To this end, we develop a novel tensor-based nonlinear classifier called a unified pinball loss intuitionistic fuzzy support tensor machine, which can successfully solve the above tasks and improve the performance of fault diagnosis in practical applications. First, the noisy multisource signals are converted into time-frequency images and reconstructed into tensor samples to mine the time-domain, frequency-domain features, and coupled structure information in the spatial domain. Next, we design two nonlinear forms of nonmembership functions in the tensor space and obtain an intuitionistic fuzzy score for each training sample to enhance the robustness of the model. Subsequently, a pinball loss function is introduced to better handle noise sensitivity and resampling instability problems. Note that, we employ the tensor robust principal component analysis method to accurately recover the low-rank tensors corrupted by sparse noise from the original tensors. Finally, two numerical examples are presented to verify the feasibility and validity of the proposed method.
更多查看译文
关键词
Tensors,Time-frequency analysis,Feature extraction,Fault diagnosis,Image reconstruction,Training,Support vector machines,intuitionistic fuzzy support tensor machine (STM),noisy multisource signals,pinball loss,tensor robust principal component analysis (TRPCA)
AI 理解论文
溯源树
样例
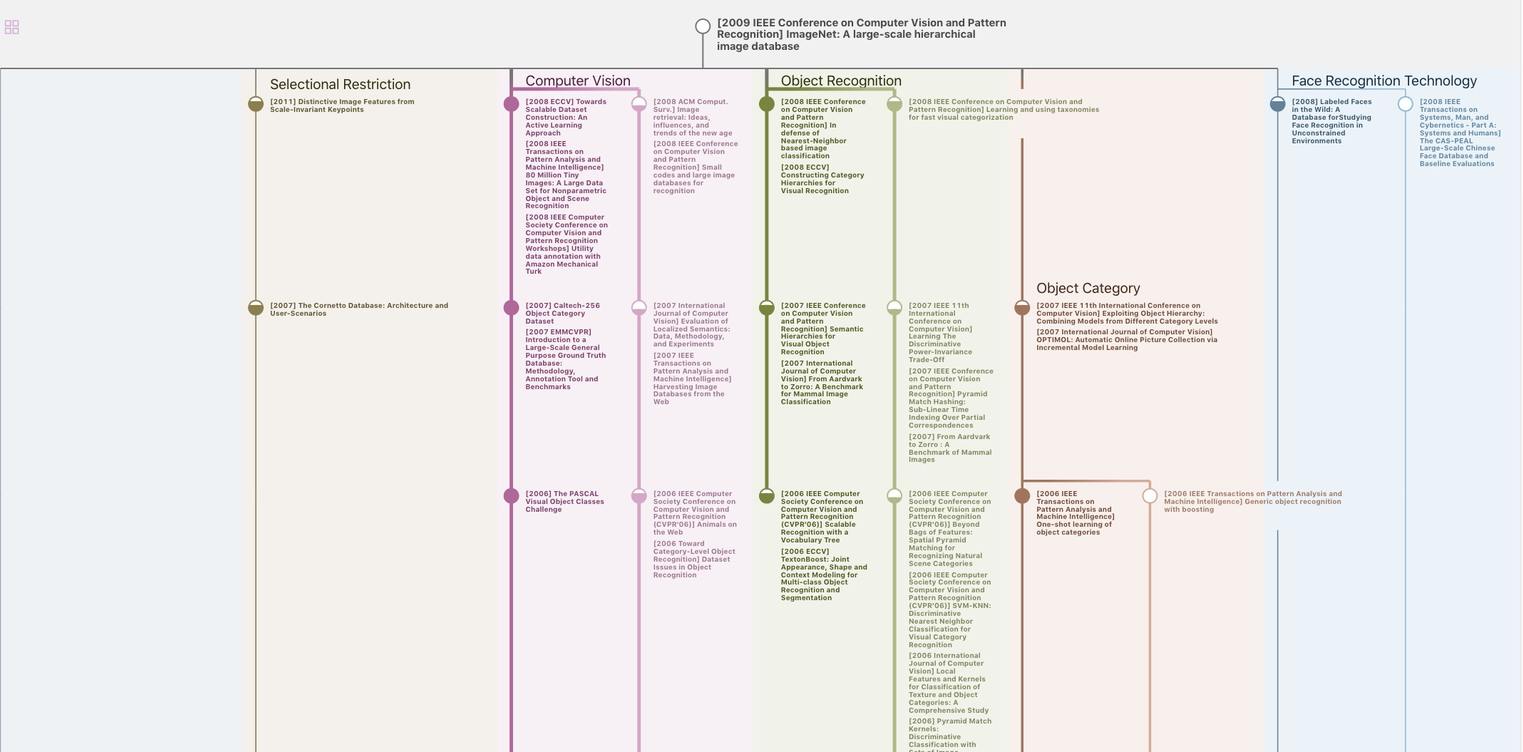
生成溯源树,研究论文发展脉络
Chat Paper
正在生成论文摘要