Exploring the Application of Large-Scale Pre-Trained Models on Adverse Weather Removal.
IEEE transactions on image processing : a publication of the IEEE Signal Processing Society(2024)
摘要
Image restoration under adverse weather conditions (e.g., rain, snow, and haze) is a fundamental computer vision problem that has important implications for various downstream applications. Distinct from early methods that are specially designed for specific types of weather, recent works tend to simultaneously remove various adverse weather effects based on either spatial feature representation learning or semantic information embedding. Inspired by various successful applications incorporating large-scale pre-trained models (e.g., CLIP), in this paper, we explore their potential benefits for leveraging large-scale pre-trained models in this task based on both spatial feature representation learning and semantic information embedding aspects: 1) spatial feature representation learning, we design a Spatially Adaptive Residual (SAR) encoder to adaptively extract degraded areas. To facilitate training of this model, we propose a Soft Residual Distillation (CLIP-SRD) strategy to transfer spatial knowledge from CLIP between clean and adverse weather images; 2) semantic information embedding, we propose a CLIP Weather Prior (CWP) embedding module to enable the network to adaptively respond to different weather conditions. This module integrates the sample-specific weather priors extracted by the CLIP image encoder with the distribution-specific information (as learned by a set of parameters) and embeds these elements using a cross-attention mechanism. Extensive experiments demonstrate that our proposed method can achieve state-of-the-art performance under various and severe adverse weather conditions. The code will be made available.
更多查看译文
关键词
Adverse Weather Removal,Image Restoration,Multi-modal Pre-trained Model
AI 理解论文
溯源树
样例
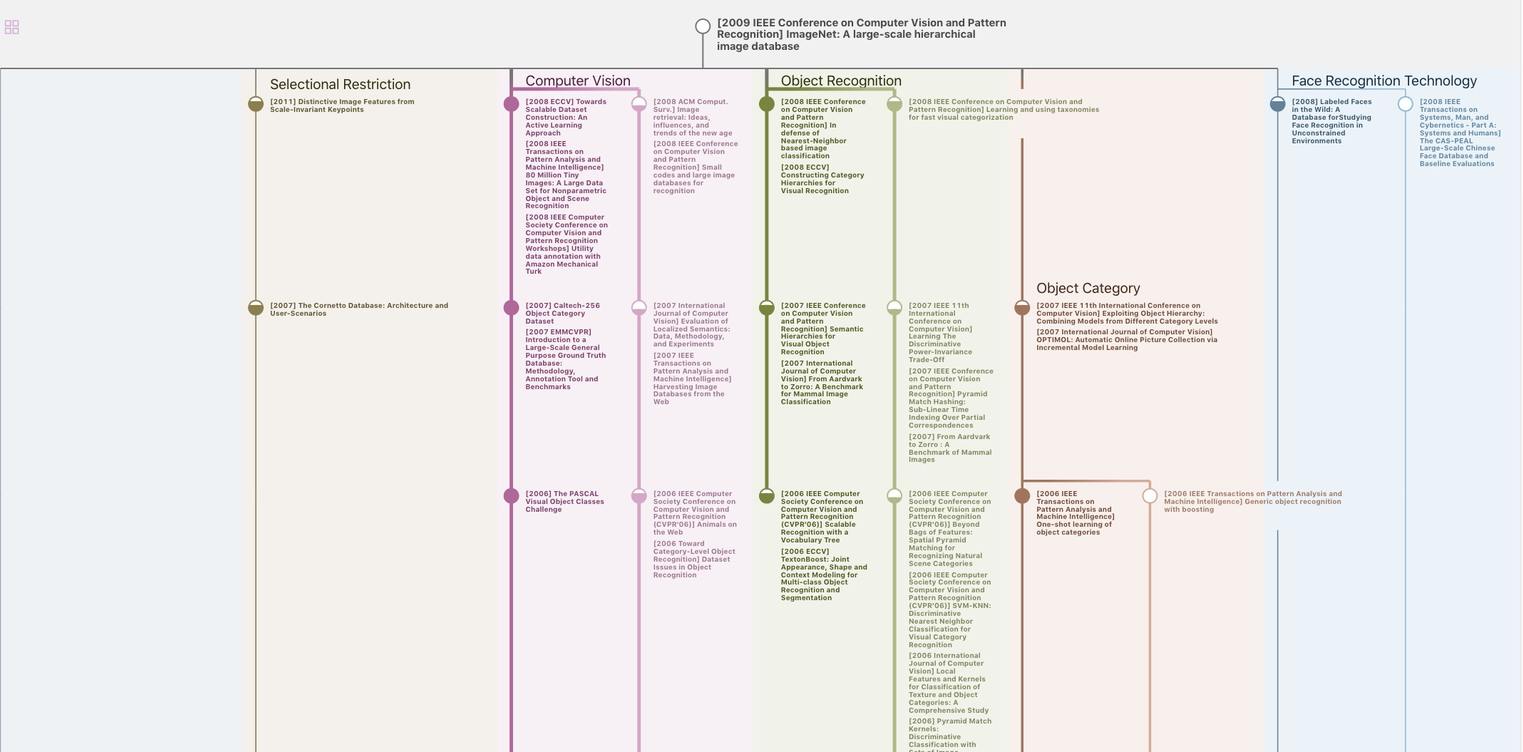
生成溯源树,研究论文发展脉络
Chat Paper
正在生成论文摘要