A Relaxed Optimization Approach for Adversarial Attacks against Neural Machine Translation Models
CoRR(2023)
摘要
In this paper, we propose an optimization-based adversarial attack against Neural Machine Translation (NMT) models. First, we propose an optimization problem to generate adversarial examples that are semantically similar to the original sentences but destroy the translation generated by the target NMT model. This optimization problem is discrete, and we propose a continuous relaxation to solve it. With this relaxation, we find a probability distribution for each token in the adversarial example, and then we can generate multiple adversarial examples by sampling from these distributions. Experimental results show that our attack significantly degrades the translation quality of multiple NMT models while maintaining the semantic similarity between the original and adversarial sentences. Furthermore, our attack outperforms the baselines in terms of success rate, similarity preservation, effect on translation quality, and token error rate. Finally, we propose a black-box extension of our attack by sampling from an optimized probability distribution for a reference model whose gradients are accessible.
更多查看译文
关键词
adversarial attacks,relaxed optimization approach,translation
AI 理解论文
溯源树
样例
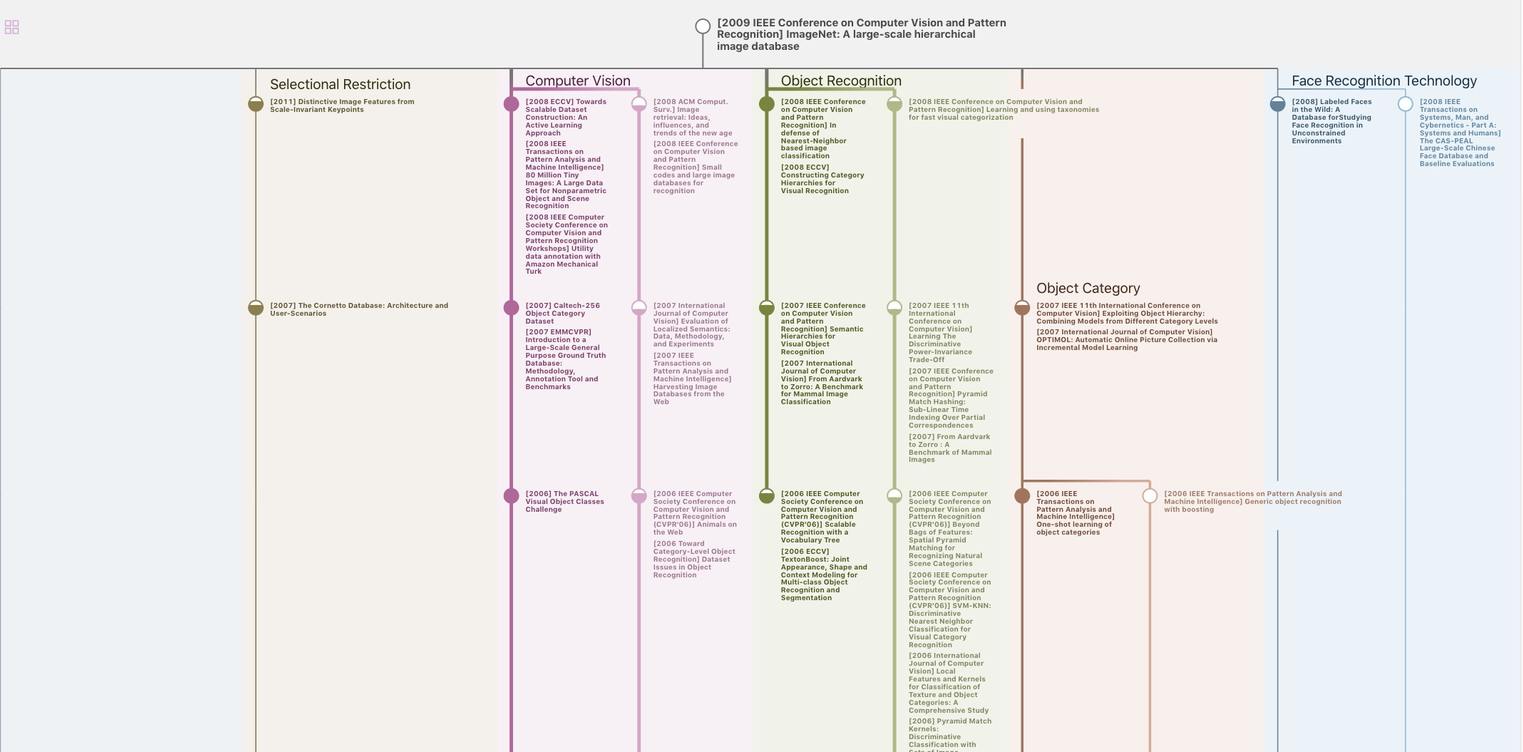
生成溯源树,研究论文发展脉络
Chat Paper
正在生成论文摘要