Better Generalization with Semantic IDs: A case study in Ranking for Recommendations
CoRR(2023)
摘要
Training good representations for items is critical in recommender models. Typically, an item is assigned a unique randomly generated ID, and is commonly represented by learning an embedding corresponding to the value of the random ID. Although widely used, this approach have limitations when the number of items are large and items are power-law distributed -- typical characteristics of real-world recommendation systems. This leads to the item cold-start problem, where the model is unable to make reliable inferences for tail and previously unseen items. Removing these ID features and their learned embeddings altogether to combat cold-start issue severely degrades the recommendation quality. Content-based item embeddings are more reliable, but they are expensive to store and use, particularly for users' past item interaction sequence. In this paper, we use Semantic IDs, a compact discrete item representations learned from content embeddings using RQ-VAE that captures hierarchy of concepts in items. We showcase how we use them as a replacement of item IDs in a resource-constrained ranking model used in an industrial-scale video sharing platform. Moreover, we show how Semantic IDs improves the generalization ability of our system, without sacrificing top-level metrics.
更多查看译文
关键词
semantic ids,ranking,recommendations
AI 理解论文
溯源树
样例
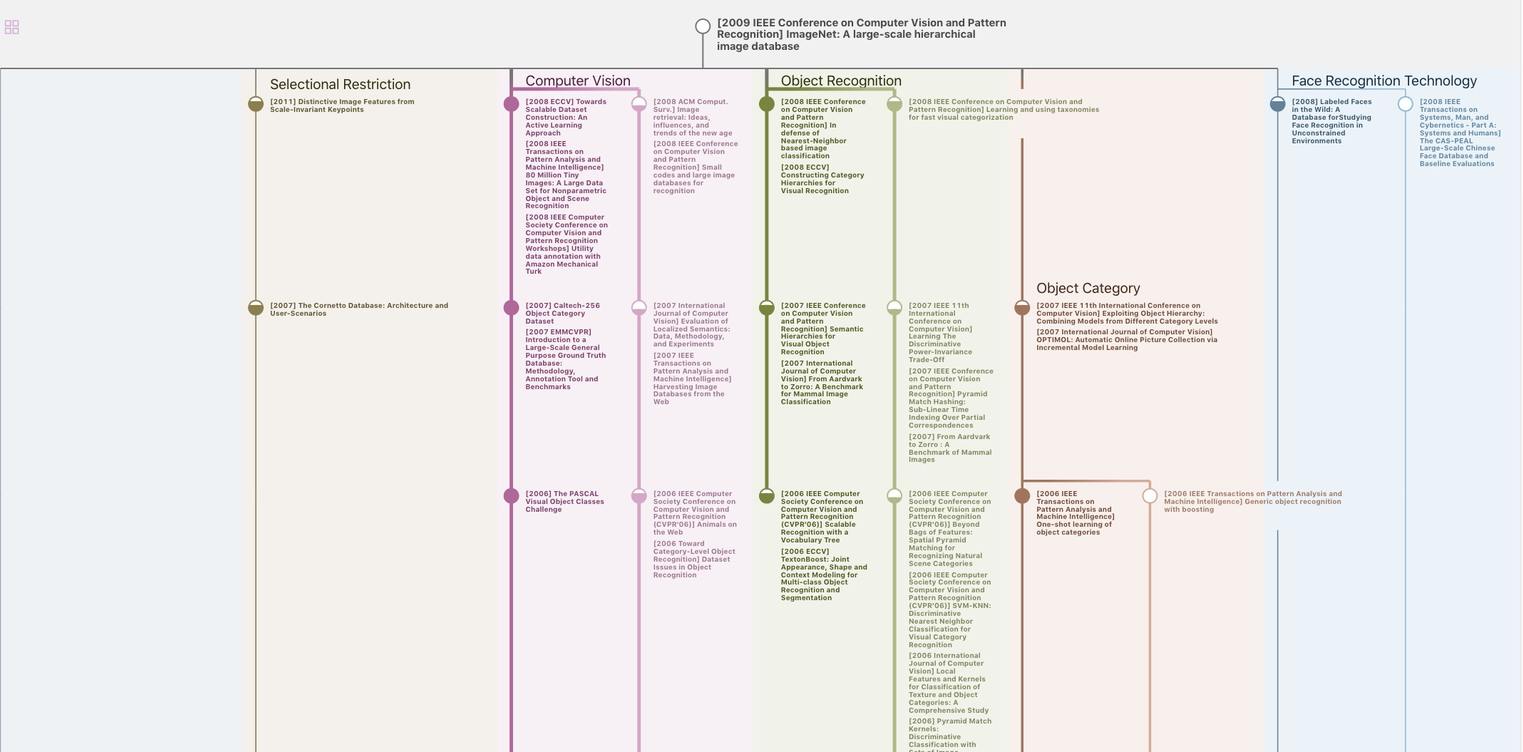
生成溯源树,研究论文发展脉络
Chat Paper
正在生成论文摘要