Can ChatGPT Enable ITS? The Case of Mixed Traffic Control via Reinforcement Learning
CoRR(2023)
摘要
The surge in Reinforcement Learning (RL) applications in Intelligent Transportation Systems (ITS) has contributed to its growth as well as highlighted key challenges. However, defining objectives of RL agents in traffic control and management tasks, as well as aligning policies with these goals through an effective formulation of Markov Decision Process (MDP), can be challenging and often require domain experts in both RL and ITS. Recent advancements in Large Language Models (LLMs) such as GPT-4 highlight their broad general knowledge, reasoning capabilities, and commonsense priors across various domains. In this work, we conduct a large-scale user study involving 70 participants to investigate whether novices can leverage ChatGPT to solve complex mixed traffic control problems. Three environments are tested, including ring road, bottleneck, and intersection. We find ChatGPT has mixed results. For intersection and bottleneck, ChatGPT increases number of successful policies by 150% and 136% compared to solely beginner capabilities, with some of them even outperforming experts. However, ChatGPT does not provide consistent improvements across all scenarios.
更多查看译文
关键词
Intelligent Transportation Systems,State Space,Control Problem,Domain Experts,Reward Function,Markov Decision Process,Successful Policy,Traffic Control,Traffic Management,Number Of Policies,Broad Knowledge,Traffic Environment,Traffic Problem,Study Group,Autonomous Vehicles,Traffic Flow,Observation Space,Expert Statement,Reinforcement Learning Problem,Proximal Policy Optimization,Reinforcement Learning Task
AI 理解论文
溯源树
样例
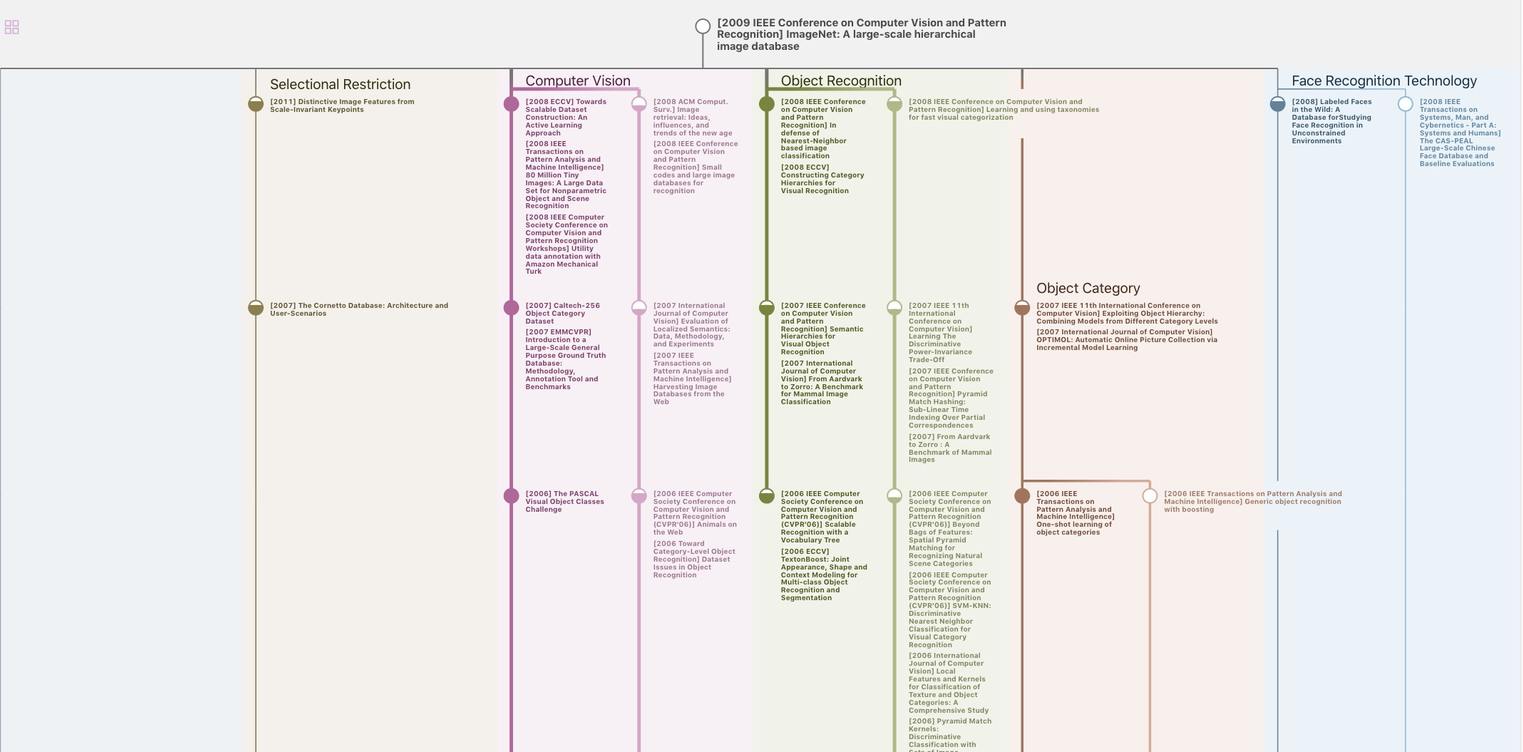
生成溯源树,研究论文发展脉络
Chat Paper
正在生成论文摘要