Dynamic Spatio-temporal Access Queries using Semi-Supervised Regression
2023 IEEE 39th International Conference on Data Engineering Workshops (ICDEW)(2023)
摘要
Understanding the cost of accessing services in a transit network, and how this varies spatially and temporally is vital for transport agencies to make effective decisions. However, to understand this at the city-scale typically demands the computation of a very large number of shortest path queries, which is computationally infeasible in a practical setting. In this work we define the notion of an access query, an analytical query which returns the aggregate access costs to a set of points of interest within a given time interval. To solve the computational bottleneck, we develop a solution that uses semi-supervised machine learning to efficiently compute these aggregate access costs using a gravity-model. The solution dynamically generates a descriptive representation of the connectivity between origins and destinations in a multi-modal network, and dynamically labels a small subset of the overall trips which are used to form a target vector for the learning algorithm. We also consider the fair distribution of access across spatio-temporal dimensions. The solution can reduce processing times by up to 97%, while maintaining high levels of accuracy; the predicted journey times to services are accurate to within 3.3 minutes, and a high level of correlation (85%) to the ground truth is achieved.
更多查看译文
关键词
Urban Analytics, Accessibility, Semi-Supervised Learning, Spatiotemporal Data
AI 理解论文
溯源树
样例
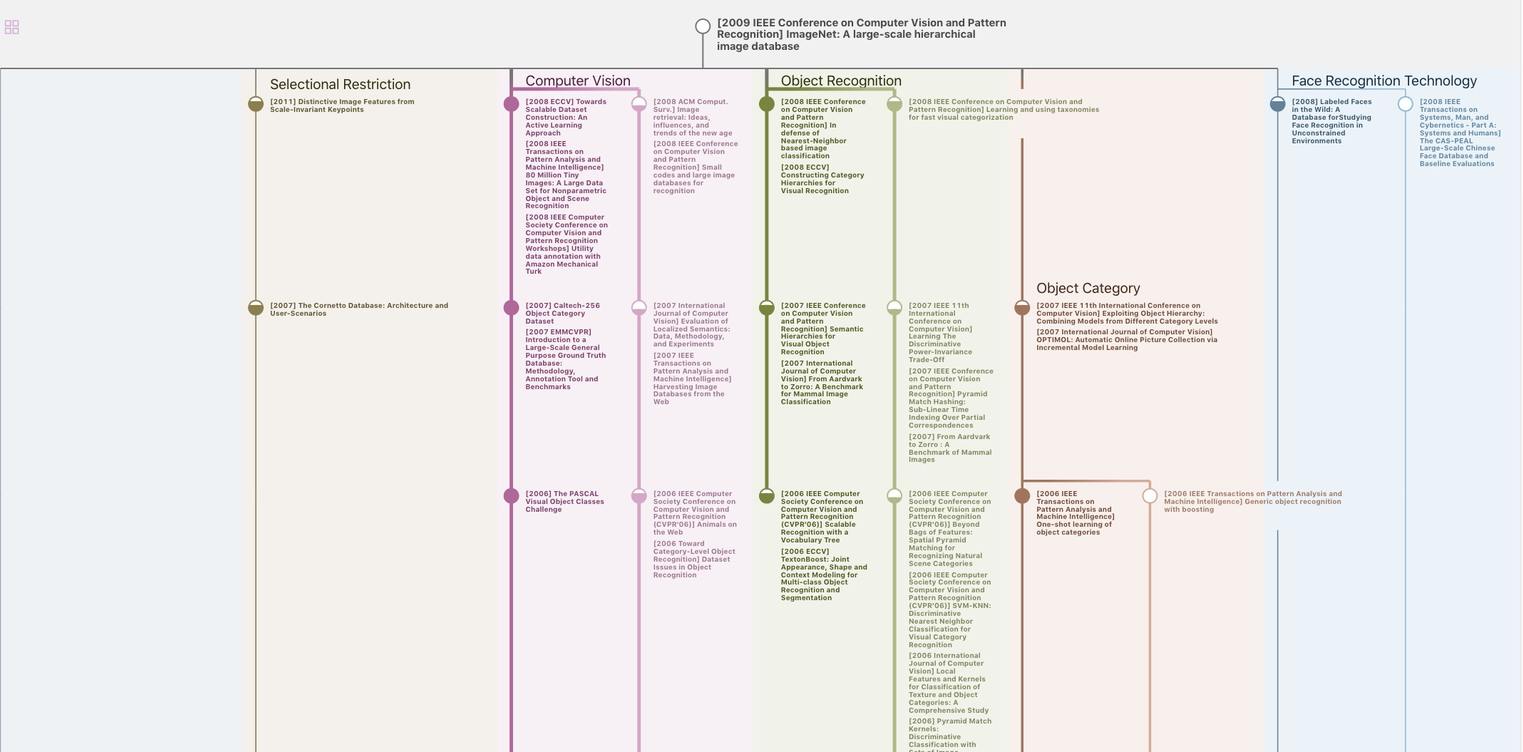
生成溯源树,研究论文发展脉络
Chat Paper
正在生成论文摘要