Neural Stream Functions
2023 IEEE 16th Pacific Visualization Symposium (PacificVis)(2023)
Abstract
We present a neural network approach to compute stream functions, which are scalar functions with gradients orthogonal to a given vector field. As a result, isosurfaces of the stream function extract stream surfaces, which can be visualized to analyze flow features. Our approach takes a vector field as input and trains an implicit neural representation to learn a stream function for that vector field. The network learns to map input coordinates to a stream function value by minimizing the inner product of the gradient of the neural network’s output and the vector field. Since stream function solutions may not be unique, we give optional constraints for the network to learn particular stream functions of interest. Specifically, we introduce regularizing loss functions that can optionally be used to generate stream function solutions whose stream surfaces follow the flow field’s curvature, or that can learn a stream function that includes a stream surface passing through a seeding rake. We also discuss considerations for properly visualizing the trained implicit network and extracting artifact-free surfaces. We compare our results with other implicit solutions and present qualitative and quantitative results for several synthetic and simulated vector fields.
MoreTranslated text
Key words
Flow Visualization,Implicit Neural Representations,Stream Surface Extraction
AI Read Science
Must-Reading Tree
Example
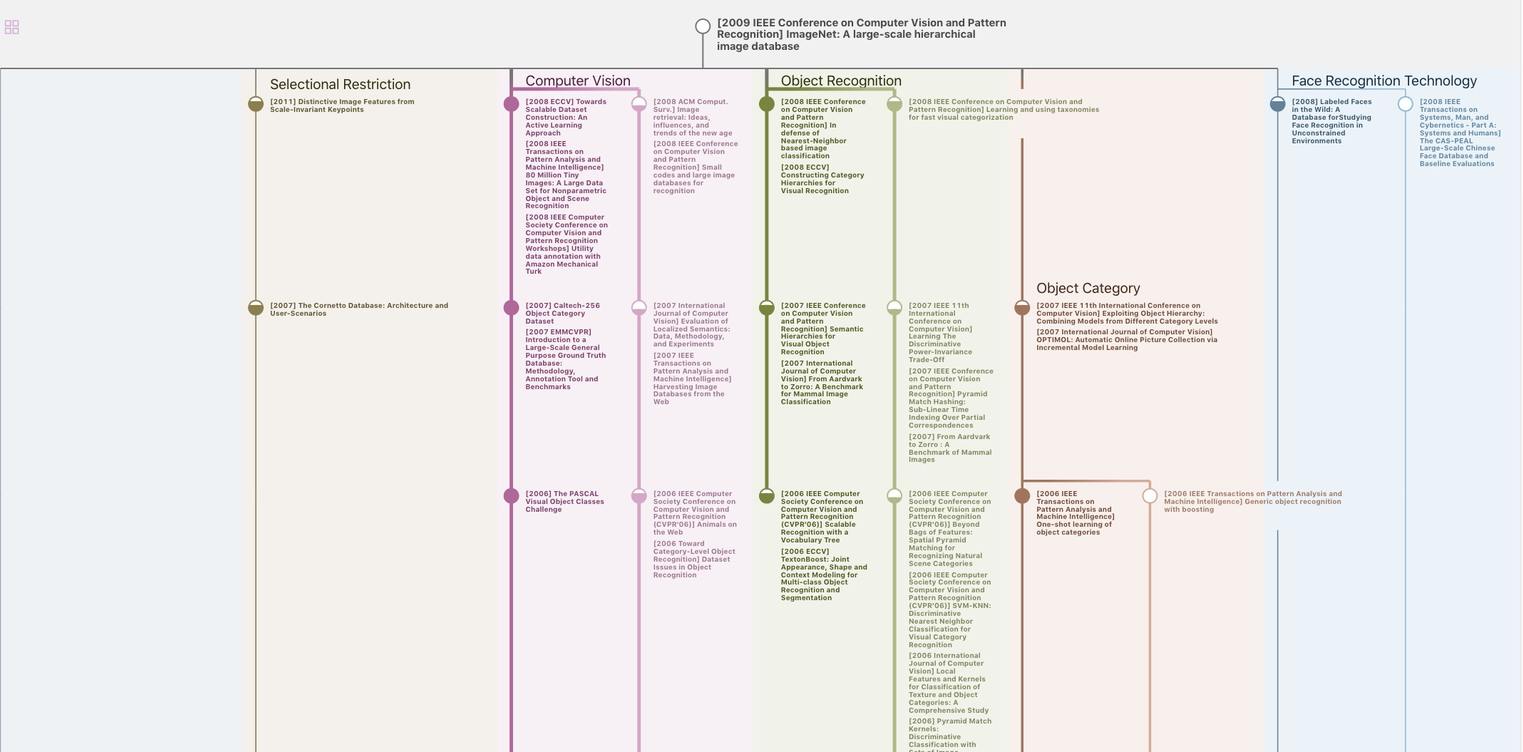
Generate MRT to find the research sequence of this paper
Chat Paper
Summary is being generated by the instructions you defined