Deep Learning-Enabled Zero-Touch Device Identification: Mitigating the Impact of Channel Variability Through MIMO Diversity
IEEE Communications Magazine(2023)
摘要
Deep learning-enabled device fingerprinting has proven efficient in enabling automated identification and authentication of transmitting devices. It does so by leveraging the transmitters' unique features that are inherent to hardware impairments caused during manufacturing to extract device-specific signatures that can be exploited to uniquely distinguish and separate between (identical) devices. Though shown to achieve promising performances, hardware fingerprinting approaches are known to suffer greatly when the training data and the testing data are generated under different channels conditions that often change when time and/or location changes. To the best of our knowledge, this work is the first to use MIMO diversity to mitigate the impact of channel variability and provide a channel-resilient device identification over flat fading channels. Specifically, we show that MIMO can increase the device classification accuracy by up to about $50\%$ when model training and testing are done over the same channel and by up to about $70\%$ when training and testing are done over different fading channels.
更多查看译文
关键词
channel variability,device,learning-enabled,zero-touch
AI 理解论文
溯源树
样例
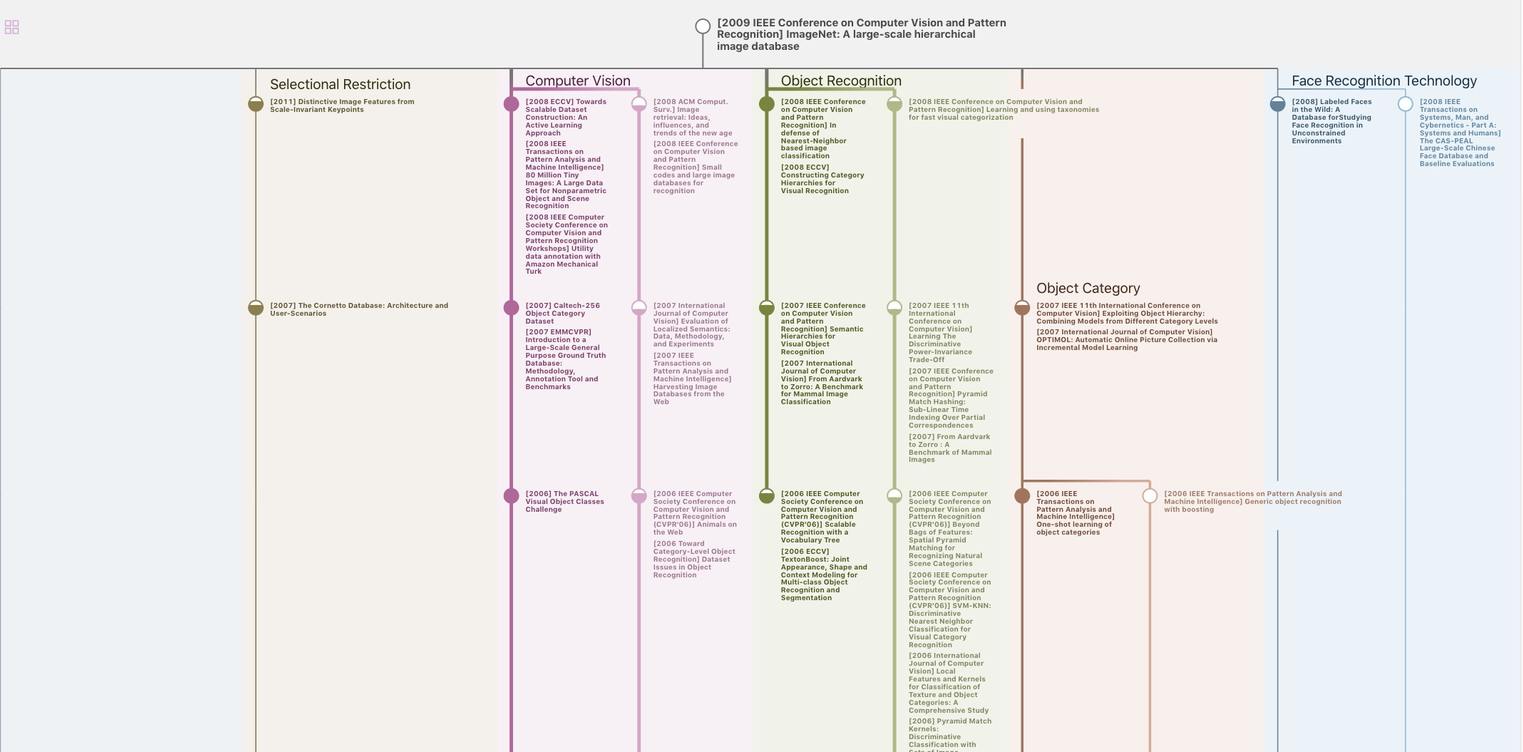
生成溯源树,研究论文发展脉络
Chat Paper
正在生成论文摘要