Unsupervised speech enhancement with deep dynamical generative speech and noise models
Conference of the International Speech Communication Association(2023)
Abstract
This work builds on a previous work on unsupervised speech enhancement using a dynamical variational autoencoder (DVAE) as the clean speech model and non-negative matrix factorization (NMF) as the noise model. We propose to replace the NMF noise model with a deep dynamical generative model (DDGM) depending either on the DVAE latent variables, or on the noisy observations, or on both. This DDGM can be trained in three configurations: noise-agnostic, noise-dependent and noise adaptation after noise-dependent training. Experimental results show that the proposed method achieves competitive performance compared to state-of-the-art unsupervised speech enhancement methods, while the noise-dependent training configuration yields a much more time-efficient inference process.
MoreTranslated text
AI Read Science
Must-Reading Tree
Example
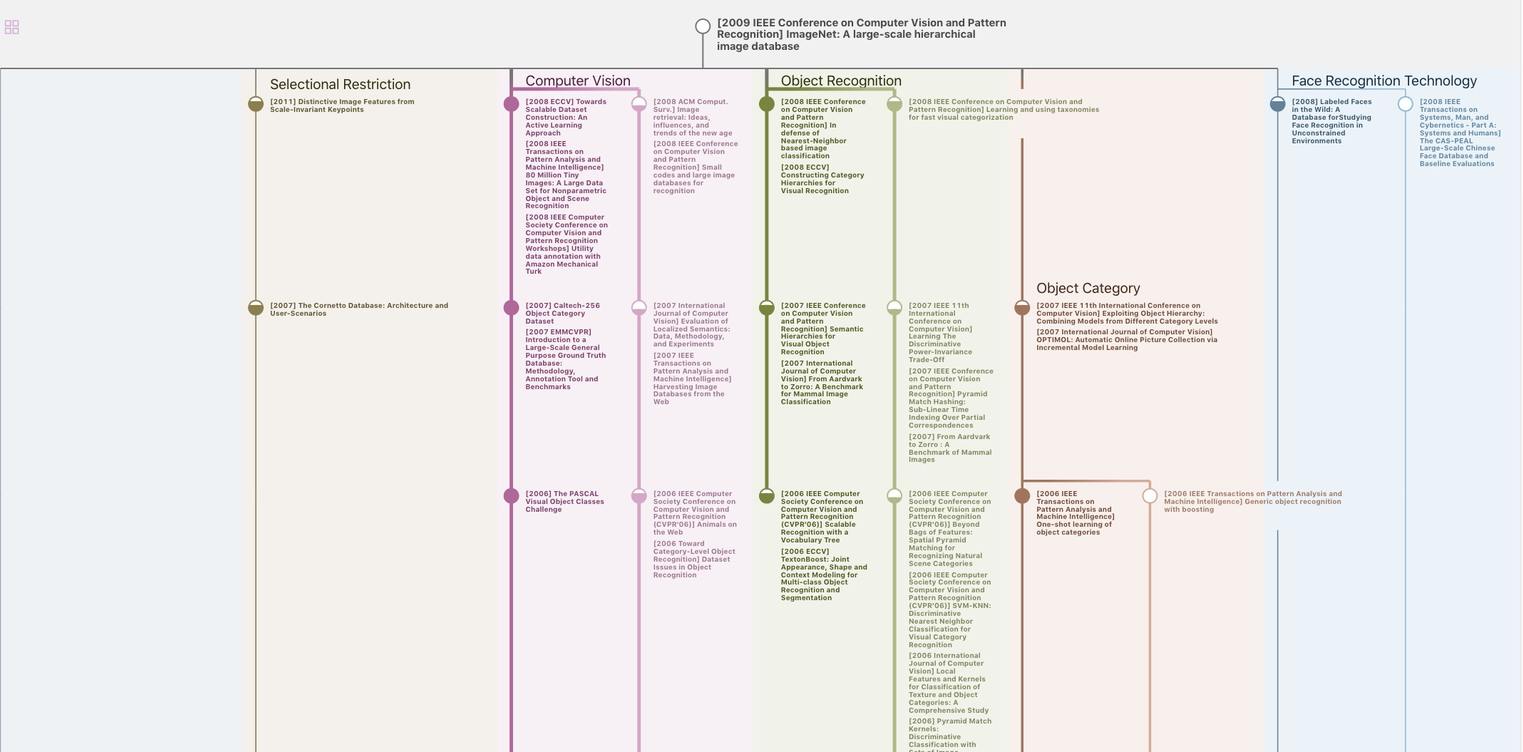
Generate MRT to find the research sequence of this paper
Chat Paper
Summary is being generated by the instructions you defined