UOD: Universal One-Shot Detection of Anatomical Landmarks
MEDICAL IMAGE COMPUTING AND COMPUTER ASSISTED INTERVENTION, MICCAI 2023, PT I(2023)
Abstract
One-shot medical landmark detection gains much attention and achieves great success for its label-efficient training process. However, existing one-shot learning methods are highly specialized in a single domain and suffer domain preference heavily in the situation of multi-domain unlabeled data. Moreover, one-shot learning is not robust that it faces performance drop when annotating a sub-optimal image. To tackle these issues, we resort to developing a domain-adaptive one-shot landmark detection framework for handling multi-domain medical images, named Universal One-shot Detection (UOD). UOD consists of two stages and two corresponding universal models which are designed as combinations of domain-specific modules and domain-shared modules. In the first stage, a domain-adaptive convolution model is self-supervised learned to generate pseudo landmark labels. In the second stage, we design a domain-adaptive transformer to eliminate domain preference and build the global context for multi-domain data. Even though only one annotated sample from each domain is available for training, the domain-shared modules help UOD aggregate all one-shot samples to detect more robust and accurate landmarks. We investigated both qualitatively and quantitatively the proposed UOD on three widely-used public X-ray datasets in different anatomical domains (i.e., head, hand, chest) and obtained state-of-the-art performances in each domain. The code is at https://github.com/heqin-zhu/UOD_universal_oneshot_detection.
MoreTranslated text
Key words
One-shot learning,Domain-adaptive model,Anatomical landmark detection,Transformer network
AI Read Science
Must-Reading Tree
Example
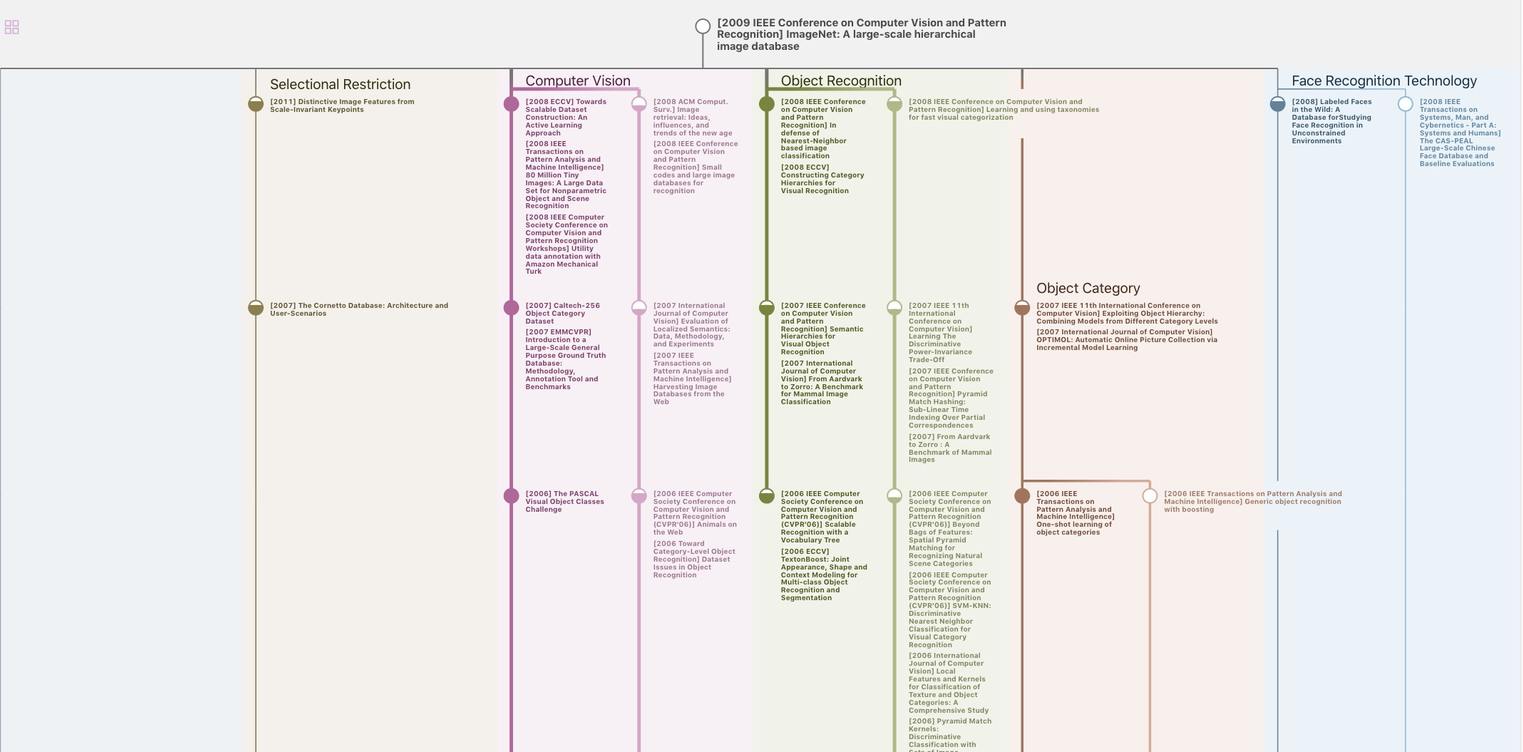
Generate MRT to find the research sequence of this paper
Chat Paper
Summary is being generated by the instructions you defined