3D molecule generation by denoising voxel grids
NeurIPS(2023)
摘要
We propose a new score-based approach to generate 3D molecules represented as atomic densities on regular grids. First, we train a denoising neural network that learns to map from a smooth distribution of noisy molecules to the distribution of real molecules. Then, we follow the neural empirical Bayes framework [Saremi and Hyvarinen, 2019] and generate molecules in two steps: (i) sample noisy density grids from a smooth distribution via underdamped Langevin Markov chain Monte Carlo, and (ii) recover the ``clean'' molecule by denoising the noisy grid with a single step. Our method, VoxMol, generates molecules in a fundamentally different way than the current state of the art (i.e., diffusion models applied to atom point clouds). It differs in terms of the data representation, the noise model, the network architecture and the generative modeling algorithm. VoxMol achieves comparable results to state of the art on unconditional 3D molecule generation while being simpler to train and faster to generate molecules.
更多查看译文
关键词
3d molecule generation
AI 理解论文
溯源树
样例
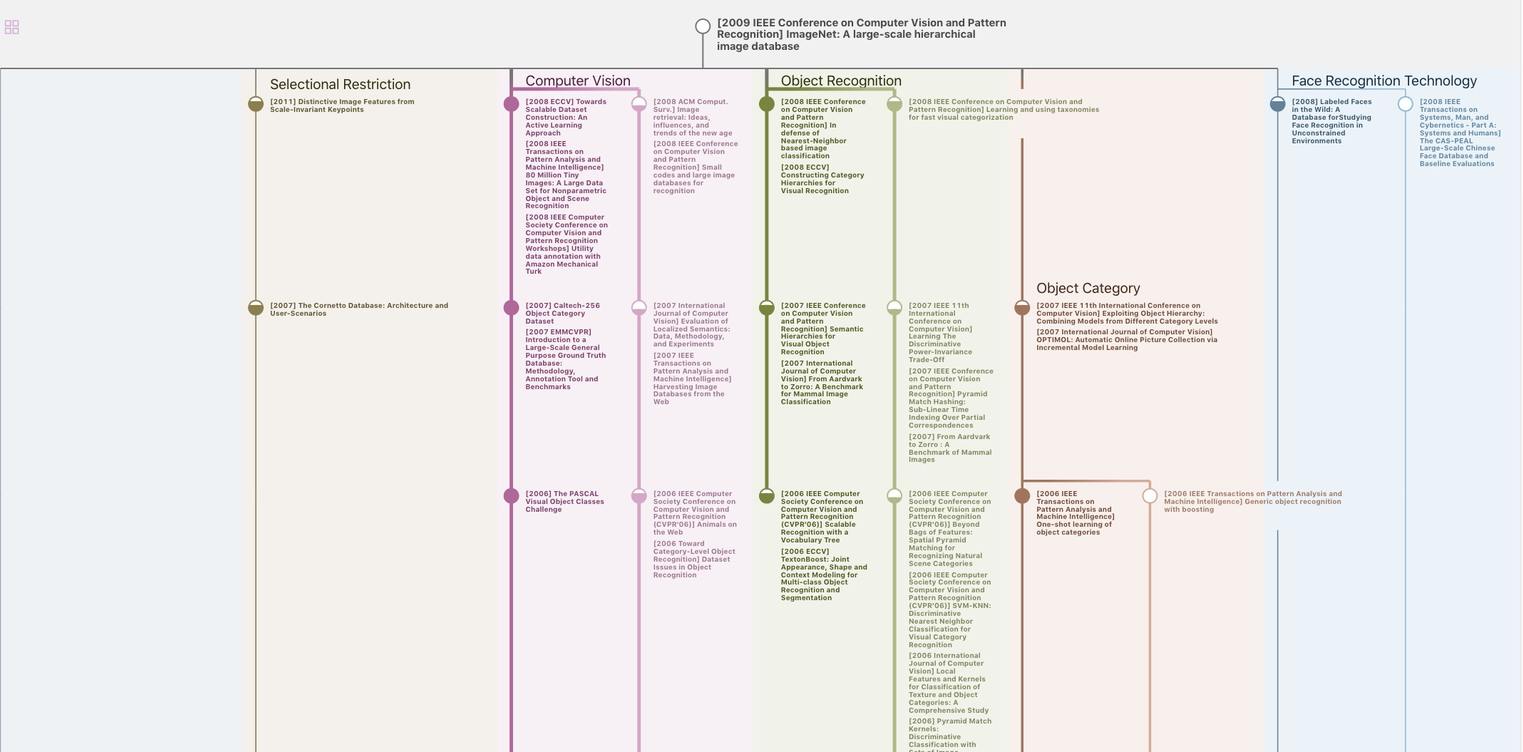
生成溯源树,研究论文发展脉络
Chat Paper
正在生成论文摘要