In-memory neural network accelerator based on phase change memory (PCM) with one-selector/one-resistor (1S1R) structure operated in the subthreshold regime
2023 IEEE International Memory Workshop (IMW)(2023)
摘要
In-memory computing (IMC) shows a disruptive potential for accelerating artificial intelligence (AI) in both inference and training tasks. Scalable IMC, however, requires novel memory technologies with extremely low current. Here we demonstrate ultra-low current matrix-vector multiplication (MVM) in a crosspoint array of phase change memory (PCM) and ovonic threshold switch (OTS) with one-selector/one-resistor (181R) structure operated in the subthreshold regime. Thanks to highly-uniform sub-$\mu$A currents, the 181R PCM crosspoint array rejects parasitic IR drop across wires, enabling excellent scaling compared to other memory devices. Our simulation of a fullyconnected neural network (FCNN) with ternary weights indicates an accuracy of 98% for MNIST classification with an array size of 512x512, which strongly supports subthreshold-operated 181R crosspoint arrays for neural network inference accelerators.
更多查看译文
关键词
Deep learning,in-memory computing,neural network accelerator,phase change memory,selector device
AI 理解论文
溯源树
样例
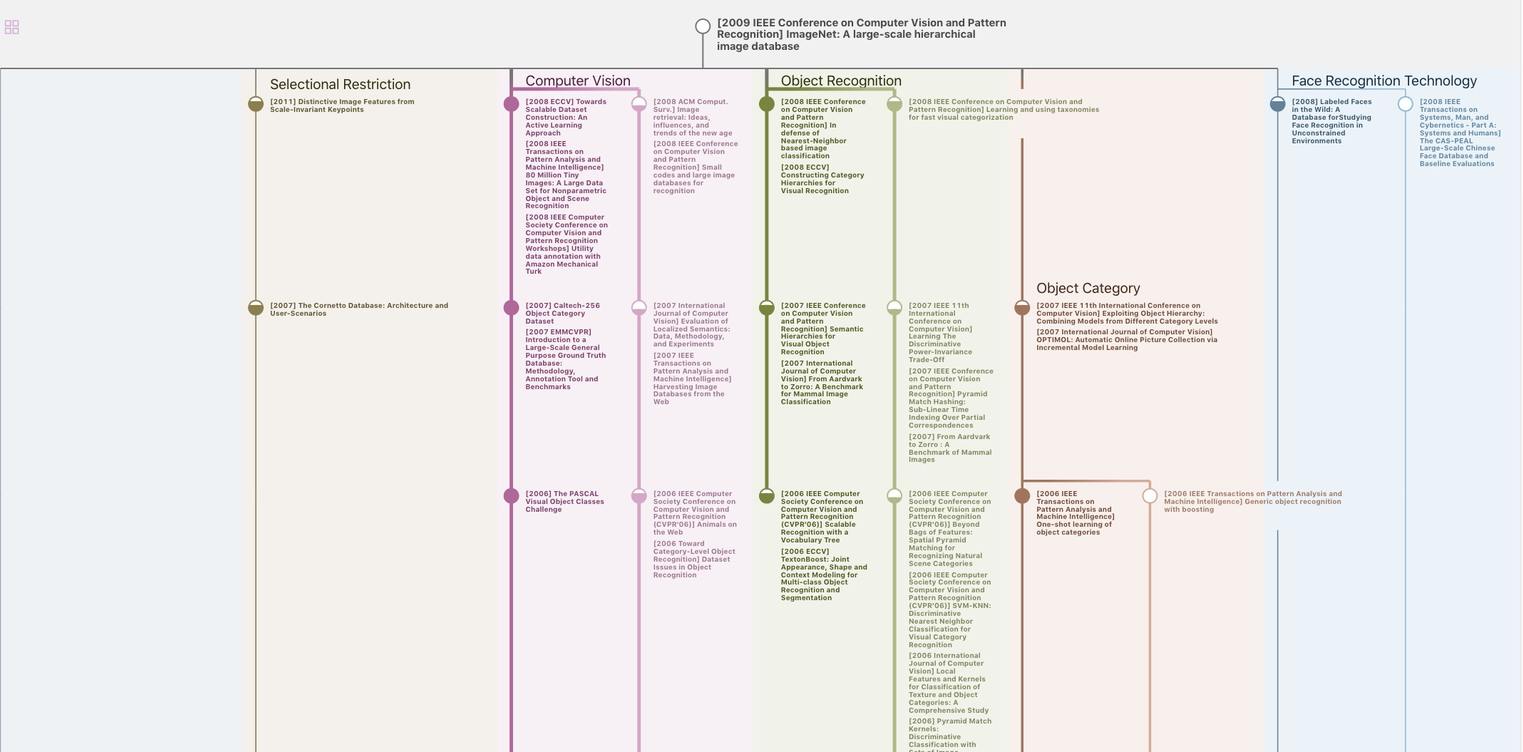
生成溯源树,研究论文发展脉络
Chat Paper
正在生成论文摘要