How robust accuracy suffers from certified training with convex relaxations
CoRR(2023)
摘要
Adversarial attacks pose significant threats to deploying state-of-the-art classifiers in safety-critical applications. Two classes of methods have emerged to address this issue: empirical defences and certified defences. Although certified defences come with robustness guarantees, empirical defences such as adversarial training enjoy much higher popularity among practitioners. In this paper, we systematically compare the standard and robust error of these two robust training paradigms across multiple computer vision tasks. We show that in most tasks and for both $\mathscr{l}_\infty$-ball and $\mathscr{l}_2$-ball threat models, certified training with convex relaxations suffers from worse standard and robust error than adversarial training. We further explore how the error gap between certified and adversarial training depends on the threat model and the data distribution. In particular, besides the perturbation budget, we identify as important factors the shape of the perturbation set and the implicit margin of the data distribution. We support our arguments with extensive ablations on both synthetic and image datasets.
更多查看译文
关键词
robust accuracy,relaxations,training
AI 理解论文
溯源树
样例
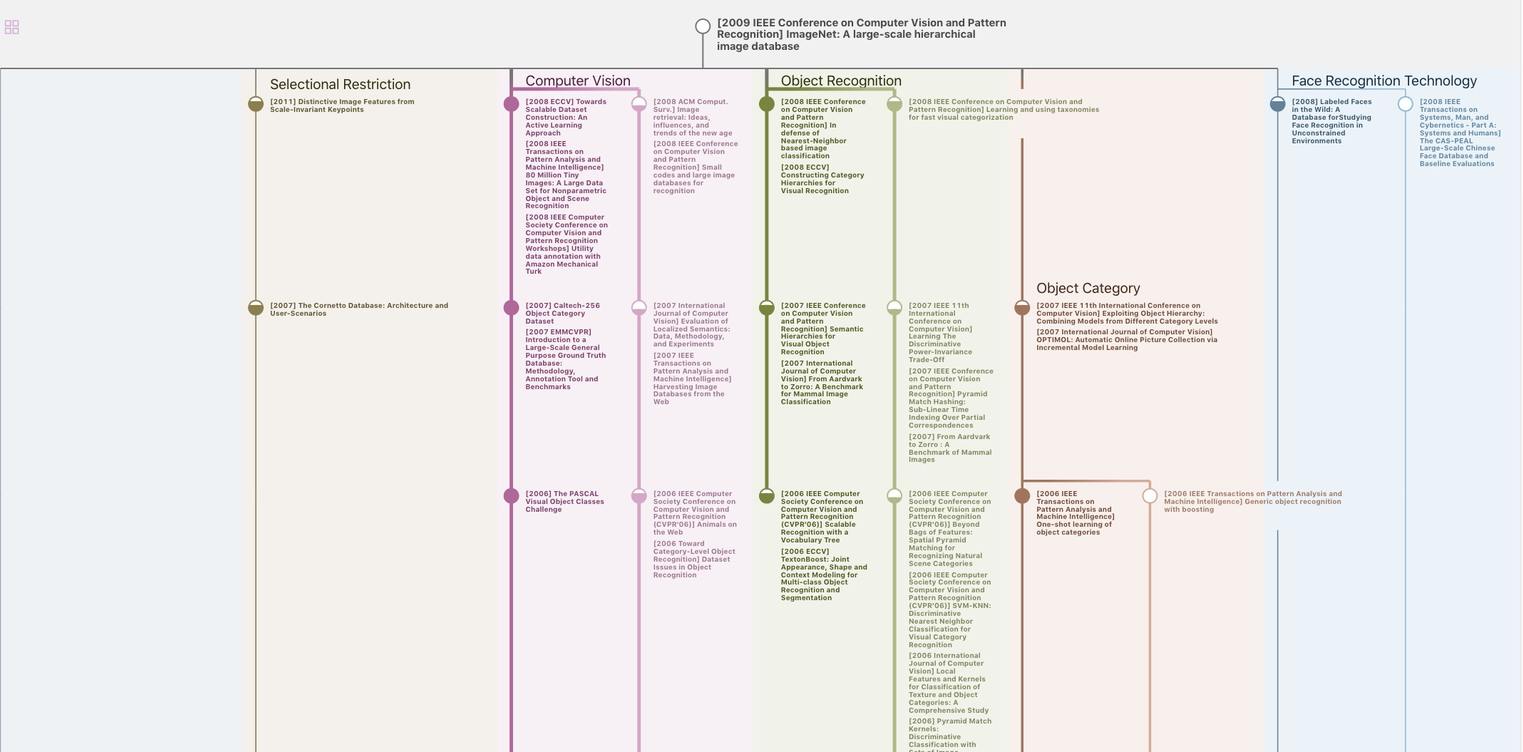
生成溯源树,研究论文发展脉络
Chat Paper
正在生成论文摘要