Continually Learning Out-of-Distribution Spatiotemporal Data for Robust Energy Forecasting
MACHINE LEARNING AND KNOWLEDGE DISCOVERY IN DATABASES: APPLIED DATA SCIENCE AND DEMO TRACK, ECML PKDD 2023, PT VII(2023)
摘要
Forecasting building energy usage is essential for promoting sustainability and reducing waste, as it enables building managers to adjust energy use to improve energy efficiency and reduce costs. This importance is magnified during anomalous periods, such as the COVID-19 pandemic, which have disrupted occupancy patterns and made accurate forecasting more challenging. Forecasting energy usage during anomalous periods is difficult due to changes in occupancy patterns and energy usage behavior. One of the primary reasons for this is the shift in distribution of occupancy patterns, with many people working or learning from home. This has created a need for new forecasting methods that can adapt to changing occupancy patterns. Online learning has emerged as a promising solution to this challenge, as it enables building managers to adapt to changes in occupancy patterns and adjust energy usage accordingly. With online learning, models can be updated incrementally with each new data point, allowing them to learn and adapt in real-time. Continual learning methods offer a powerful solution to address the challenge of catastrophic forgetting in online learning, allowing energy forecasting models to retain valuable insights while accommodating new data and improving generalization in out-of-distribution scenarios. Another solution is to use human mobility data as a proxy for occupancy, leveraging the prevalence of mobile devices to track movement patterns and infer occupancy levels. Human mobility data can be useful in this context as it provides a way to monitor occupancy patterns without relying on traditional sensors or manual data collection methods. We have conducted extensive experiments using data from four buildings to test the efficacy of these approaches. However, deploying these methods in the real world presents several challenges.
更多查看译文
关键词
Forecasting,Energy,Continual Learning,COVID
AI 理解论文
溯源树
样例
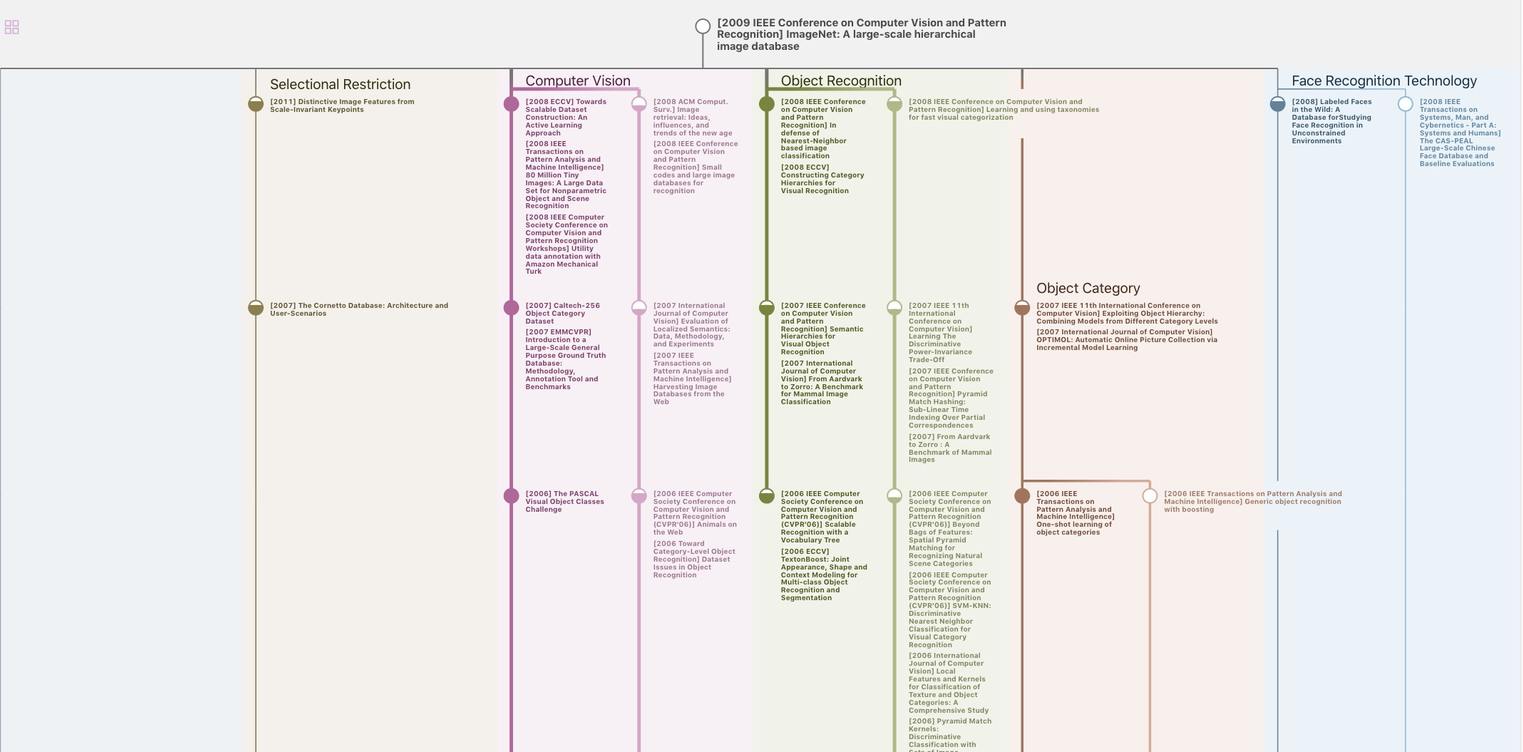
生成溯源树,研究论文发展脉络
Chat Paper
正在生成论文摘要