Evading Machine-Learning-Based Android Malware Detector for IoT Devices
IEEE Systems Journal(2023)
摘要
Securing the Android gadgets from malware activities is a major concern in Internet of Things, and the drastic rise in adversarial attacks makes this problem more challenging. Existing Android adversarial techniques focus on the feature vector space manipulations through complex deep learning techniques, and the operational integrity is also not experimented. This work implements two evasion attack scenarios on the feature vector of Android apks and creates functionality preserved Android malware. The malware samples are injected with features that are absent in malicious samples, but found in legitimate applications. The sample thus formed will be identical in functionality, besides having statistical dissimilarity. One of the attack scenarios implemented is through feature similarity using Euclidean distance (ED), which measures between malware and benign samples. The other version of attack is by generating variants through particle swarm optimization (PSO). We find that our evasion algorithms are not highly biased nor complex, making them easier to train and understand compared to generative adversarial neural networks. The experiments were carried out on real-world Android applications from AndroZoo and AMD. We could successfully achieve the highest performance of 100% evasion rate with the PSO algorithm and 89.6% evasion rate with the ED algorithm with the lowest computational complexity compared to machine learning or deep learning mechanisms.
更多查看译文
关键词
Adversarial machine learning (ML),Android,evasion attack,malware detection
AI 理解论文
溯源树
样例
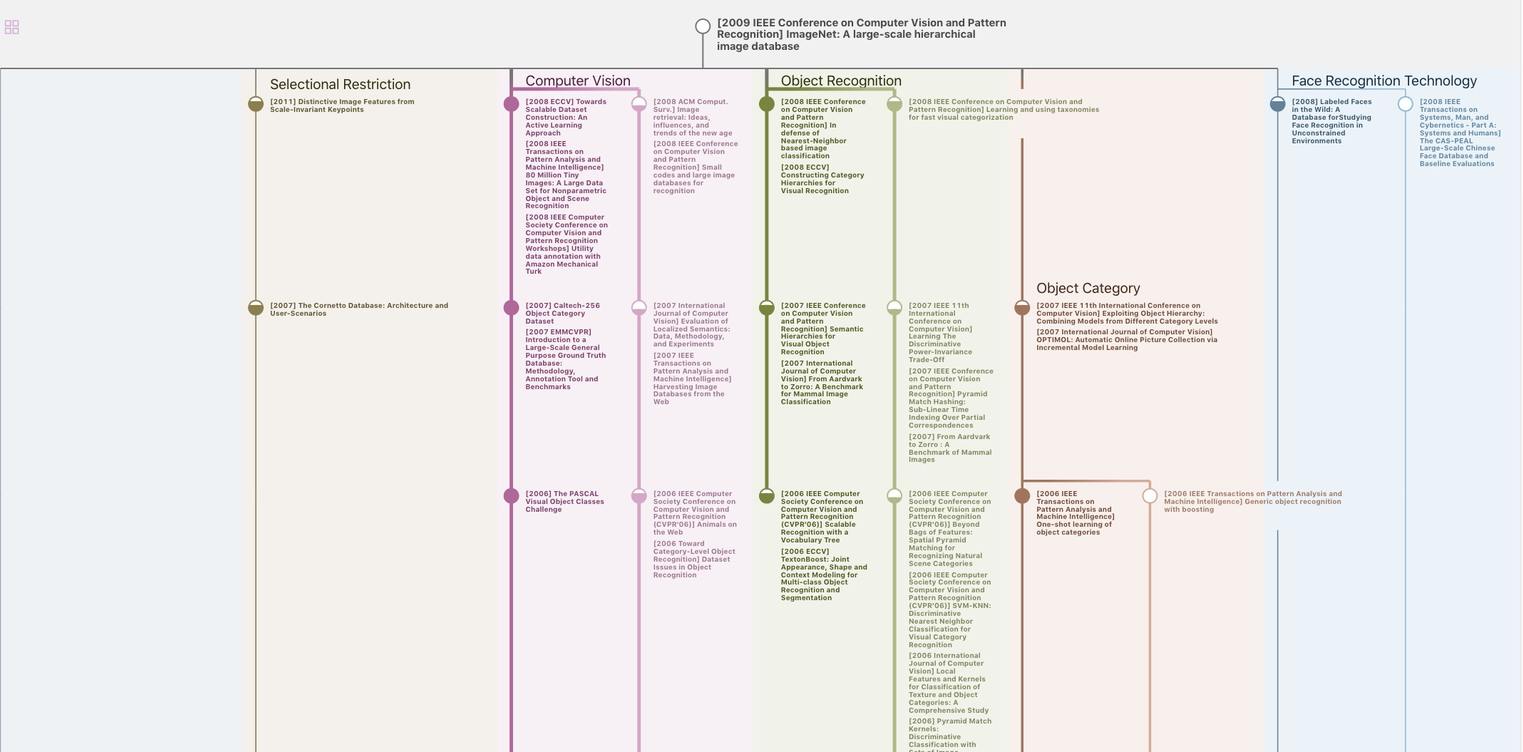
生成溯源树,研究论文发展脉络
Chat Paper
正在生成论文摘要