Deep Learning for the Detection of Emotion in Human Speech: The Impact of Audio Sample Duration and English versus Italian Languages
2023 32nd Wireless and Optical Communications Conference (WOCC)(2023)
摘要
Identification of emotion types is important in the diagnosis and treatment of certain mental illnesses. This study uses audio data and deep learning methods such as convolutional neural networks (CNN) and long short-term memory (LSTM) to classify the emotion of human speech. We use the IEMOCAP and DEMoS datasets, consisting of English and Italian audio speech data in our experiments to classify speech into one of up to four emotions: angry, happy, neutral, and sad. The classification performance results demonstrate the effectiveness of the deep learning methods and our experiments yield between 62 and 92 percent classification accuracies. We specifically investigate the impact of the audio sample duration on the classification accuracy. In addition, we examine and compare the classification accuracy for English versus Italian languages.
更多查看译文
关键词
emotion recognition,deep learning,spectrogram,convolutional neural network (CNN),long short-term memory (LSTM)
AI 理解论文
溯源树
样例
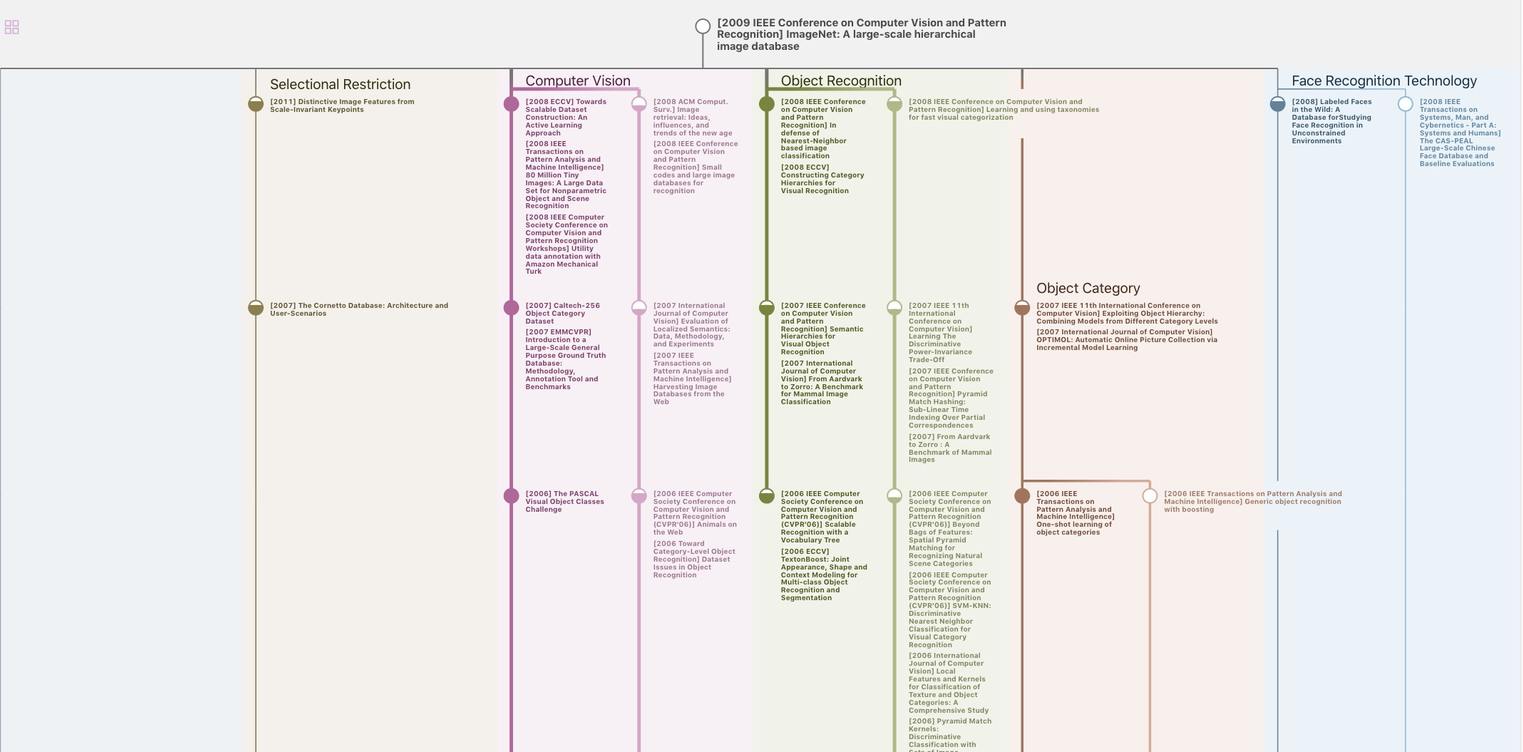
生成溯源树,研究论文发展脉络
Chat Paper
正在生成论文摘要