On a Novel Content Edge Caching Approach based on Multi-Agent Federated Reinforcement Learning in Internet of Vehicles
2023 32nd Wireless and Optical Communications Conference (WOCC)(2023)
摘要
Driven by the emerging requirements of Internet of Vehicles (IoV), future vehicles are expected to have the ability to provide not only the autonomous driving services, but also the multimedia services for working and entertainment. The edge caching service enabled by the Road Side Units (RSUs) can complement the limited environment perceiving and computing ability of future vehicles to gather, pre-process, and cache the contents of driving assistance, work, and entertainments. In this paper, we use federated learning to learn the popularity variation tendency considering user preference in different districts and their concerns for privacy-preserving. We further split the possible contents into blocks and use completely cooperative multi-agent reinforcement learning based on Deep Q network to make a more flexible and accurate caching decision considering the various emergency levels and delay requirements of different contents. Numerical results demonstrate that the proposed method outperforms traditional caching strategies.
更多查看译文
关键词
Edge caching,federated learning,multi-agent reinforcement learning,Deep Q Network
AI 理解论文
溯源树
样例
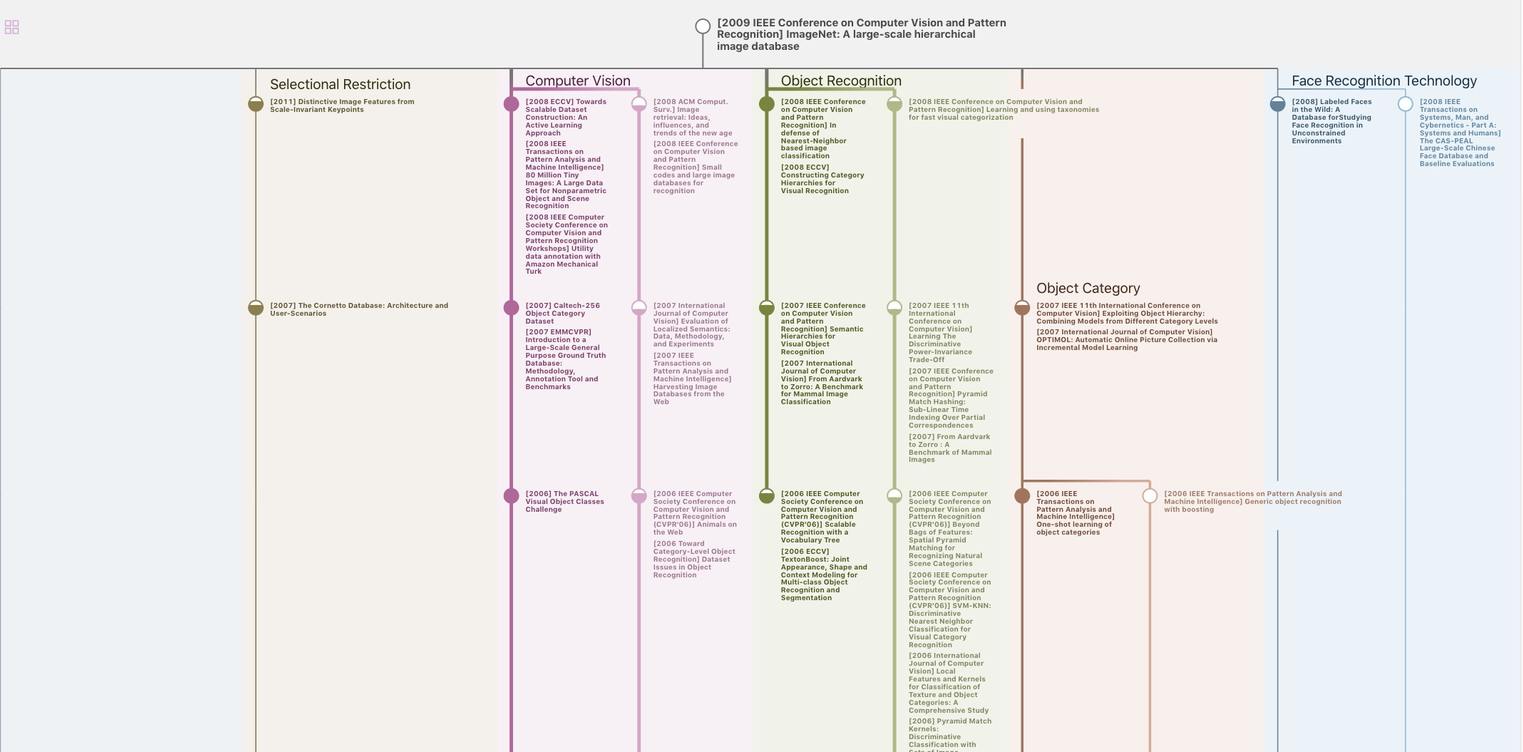
生成溯源树,研究论文发展脉络
Chat Paper
正在生成论文摘要