Deep Stacked Autoencoder-Based Long-Term Spectrum Prediction Using Real-World Data
IEEE Transactions on Cognitive Communications and Networking(2023)
Abstract
Spectrum prediction is challenging due to its multi-dimension, complex inherent dependency, and heterogeneity among the spectrum data. In this paper, we first propose a stacked autoencoder (SAE) and bi-directional long short-term memory (Bi-LSTM) based spectrum prediction method (SAEL-SP). Specifically, a SAE is designed to extract the hidden features (semantic coding) of spectrum data in an unsupervised manner. Then, the output of SAE is connected to a predictor (Bi-LSTM), which is used for long-term prediction by learning hidden features. The main advantage of SAEL-SP is that the underlying features of spectrum data can be retained automatically, layer by layer, rather than designing them manually. To further improve the prediction accuracy of SAEL-SP and achieve a wider bandwidth prediction, we propose a SAE-based spectrum prediction method using temporal-spectral-spatial features of data (SAE-TSS). Different from SAEL-SP, the input of SAE-TSS is the image format. SAE-TSS achieves higher prediction accuracy than SAEL-SP using the features extracted from time, frequency, and space dimensions. We use a real-world spectrum dataset to validate the effectiveness of two prediction frameworks. Experiment results show that both SAEL-SP and SAE-TSS outperform existing spectrum prediction approaches.
MoreTranslated text
Key words
Predictive models,Feature extraction,Hidden Markov models,Time-frequency analysis,Frequency measurement,Autoregressive processes,Data models,Spectrum prediction,temporal-spectral-spatial correlations,deep learning,stacked autoencoder
AI Read Science
Must-Reading Tree
Example
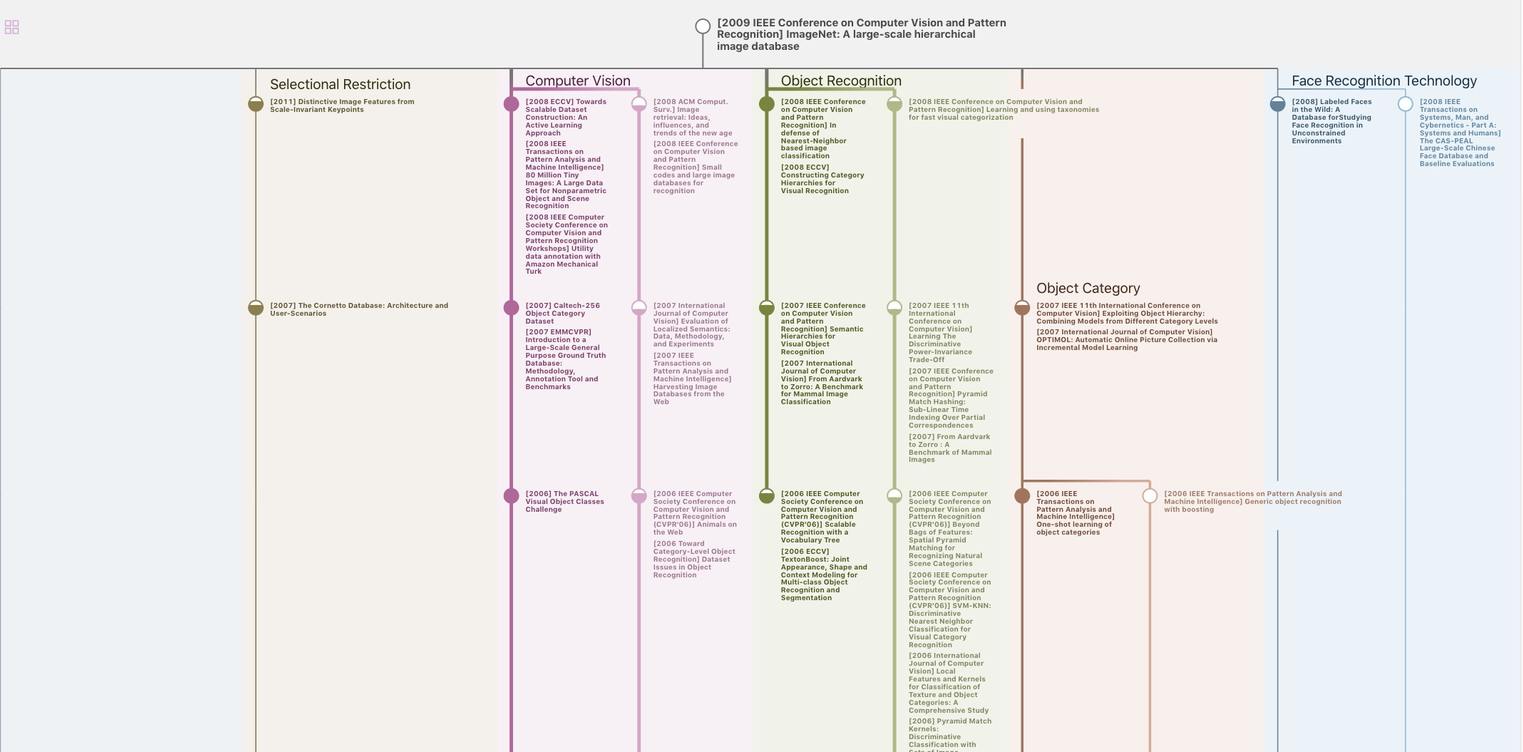
Generate MRT to find the research sequence of this paper
Chat Paper
Summary is being generated by the instructions you defined