Semiparametric posterior corrections
arxiv(2023)
摘要
We present a new approach to semiparametric inference using corrected posterior distributions. The method allows us to leverage the adaptivity, regularization and predictive power of nonparametric Bayesian procedures to estimate low-dimensional functionals of interest without being restricted by the holistic Bayesian formalism. Starting from a conventional nonparametric posterior, we target the functional of interest by transforming the entire distribution with a Bayesian bootstrap correction. We provide conditions for the resulting $\textit{one-step posterior}$ to possess calibrated frequentist properties and specialize the results for several canonical examples: the integrated squared density, the mean of a missing-at-random outcome, and the average causal treatment effect on the treated. The procedure is computationally attractive, requiring only a simple, efficient post-processing step that can be attached onto any arbitrary posterior sampling algorithm. Using the ACIC 2016 causal data analysis competition, we illustrate that our approach can outperform the existing state-of-the-art through the propagation of Bayesian uncertainty.
更多查看译文
关键词
semiparametric posterior corrections
AI 理解论文
溯源树
样例
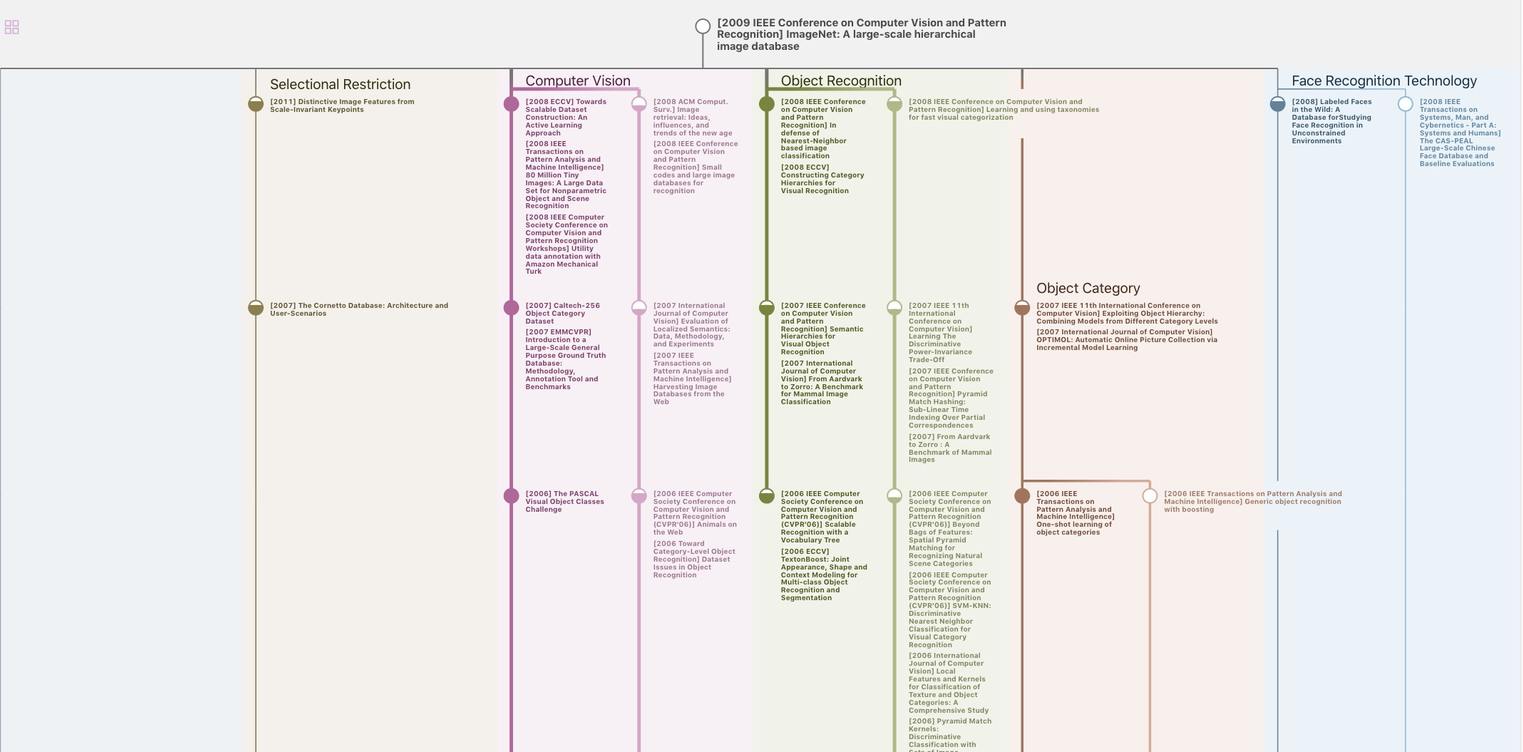
生成溯源树,研究论文发展脉络
Chat Paper
正在生成论文摘要