How Does Fine-Tuning Impact Out-of-Distribution Detection for Vision-Language Models?
International Journal of Computer Vision(2024)
Abstract
Recent large vision-language models such as CLIP have shown remarkable out-of-distribution (OOD) detection and generalization performance. However, their zero-shot in-distribution (ID) accuracy is often limited for downstream datasets. Recent CLIP-based fine-tuning methods such as prompt learning have demonstrated significant improvements in ID classification and OOD generalization where OOD labels are available. Nonetheless, it remains unclear whether the model is reliable to semantic shifts without OOD labels. In this paper, we aim to bridge the gap and present a comprehensive study to understand how fine-tuning impact OOD detection for few-shot downstream tasks. By framing OOD detection as multi-modal concept matching, we establish a connection between fine-tuning methods and various OOD scores. Our results suggest that a proper choice of OOD scores is essential for CLIP-based fine-tuning. In particular, the maximum concept matching (MCM) score provides a promising solution consistently. We also show that prompt learning demonstrates the state-of-the-art OOD detection performance over the zero-shot counterpart.
MoreTranslated text
Key words
CLIP,OOD detection,Fine-tuning,Multi-modality,Vision-language models,Prompt learning,Few-shot learning,Adaptor
AI Read Science
Must-Reading Tree
Example
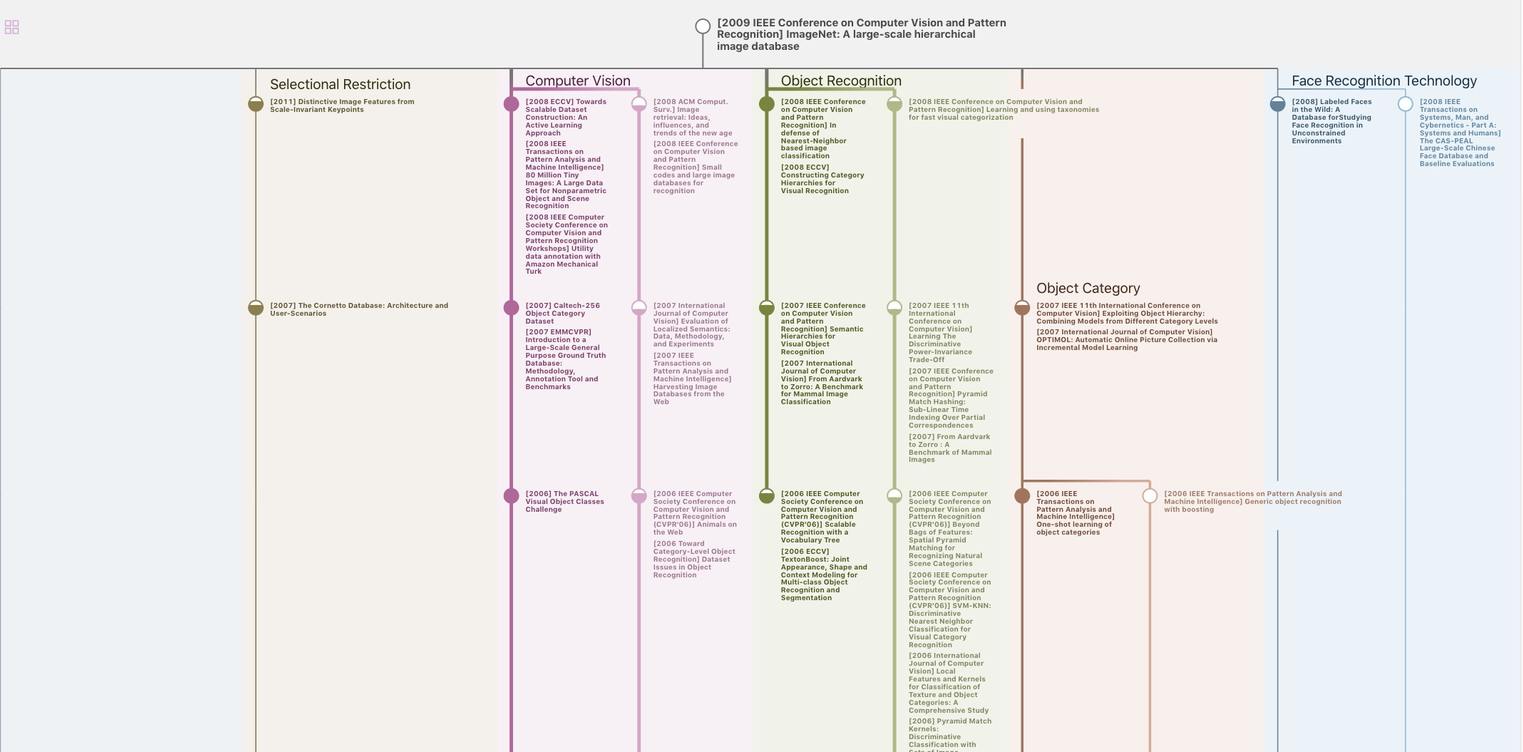
Generate MRT to find the research sequence of this paper
Chat Paper
Summary is being generated by the instructions you defined