Explaining Reinforcement Learning with Shapley Values.
ICML 2023(2023)
摘要
For reinforcement learning systems to be widely adopted, their users must understand and trust them. We present a theoretical analysis of explaining reinforcement learning using Shapley values, following a principled approach from game theory for identifying the contribution of individual players to the outcome of a cooperative game. We call this general framework Shapley Values for Explaining Reinforcement Learning (SVERL). Our analysis exposes the limitations of earlier uses of Shapley values in reinforcement learning. We then develop an approach that uses Shapley values to explain agent performance. In a variety of domains, SVERL produces meaningful explanations that match and supplement human intuition.
更多查看译文
关键词
reinforcement learning,values
AI 理解论文
溯源树
样例
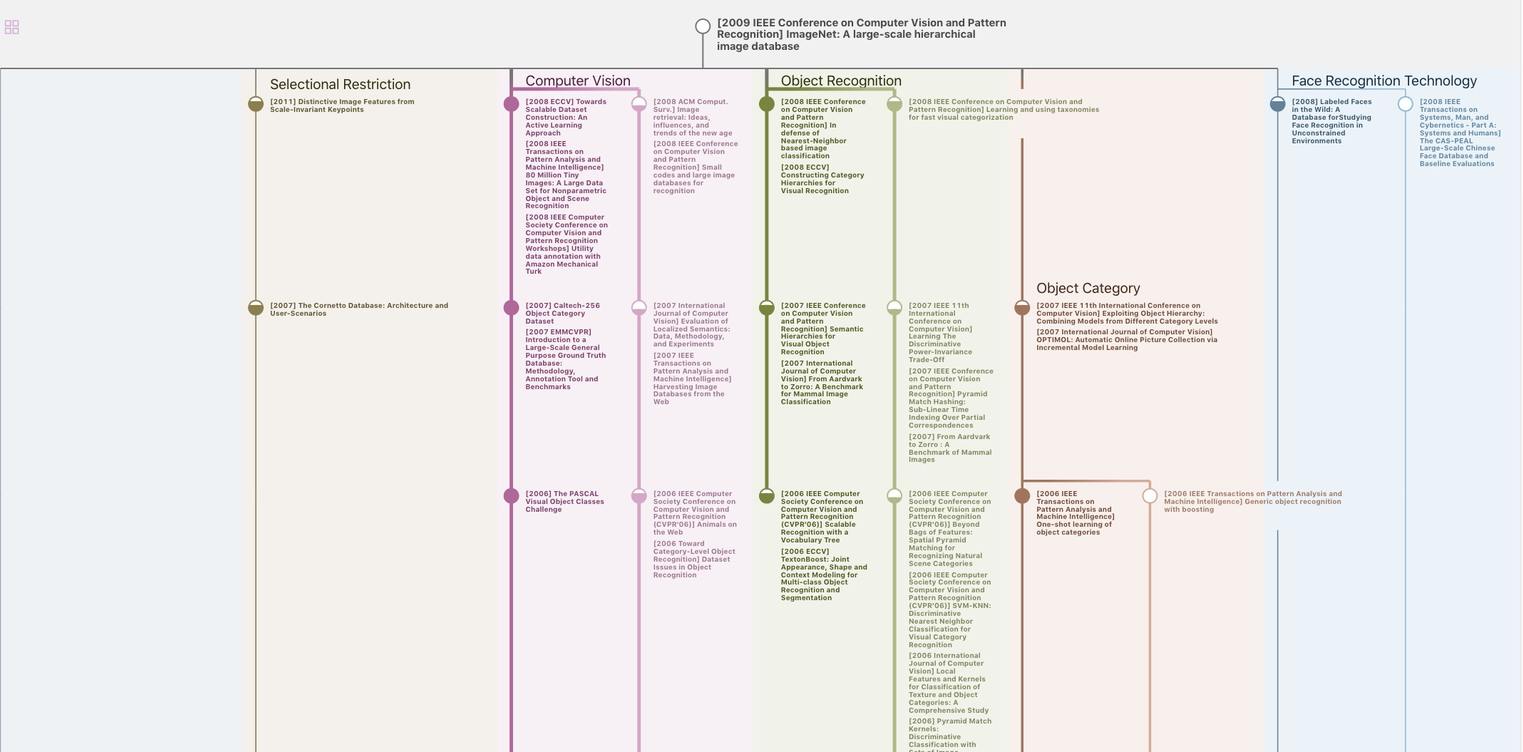
生成溯源树,研究论文发展脉络
Chat Paper
正在生成论文摘要