Self-Paced Absolute Learning Progress as a Regularized Approach to Curriculum Learning
CoRR(2023)
摘要
The usability of Reinforcement Learning is restricted by the large computation times it requires. Curriculum Reinforcement Learning speeds up learning by defining a helpful order in which an agent encounters tasks, i.e. from simple to hard. Curricula based on Absolute Learning Progress (ALP) have proven successful in different environments, but waste computation on repeating already learned behaviour in new tasks. We solve this problem by introducing a new regularization method based on Self-Paced (Deep) Learning, called Self-Paced Absolute Learning Progress (SPALP). We evaluate our method in three different environments. Our method achieves performance comparable to original ALP in all cases, and reaches it quicker than ALP in two of them. We illustrate possibilities to further improve the efficiency and performance of SPALP.
更多查看译文
关键词
curriculum,learning,progress,self-paced
AI 理解论文
溯源树
样例
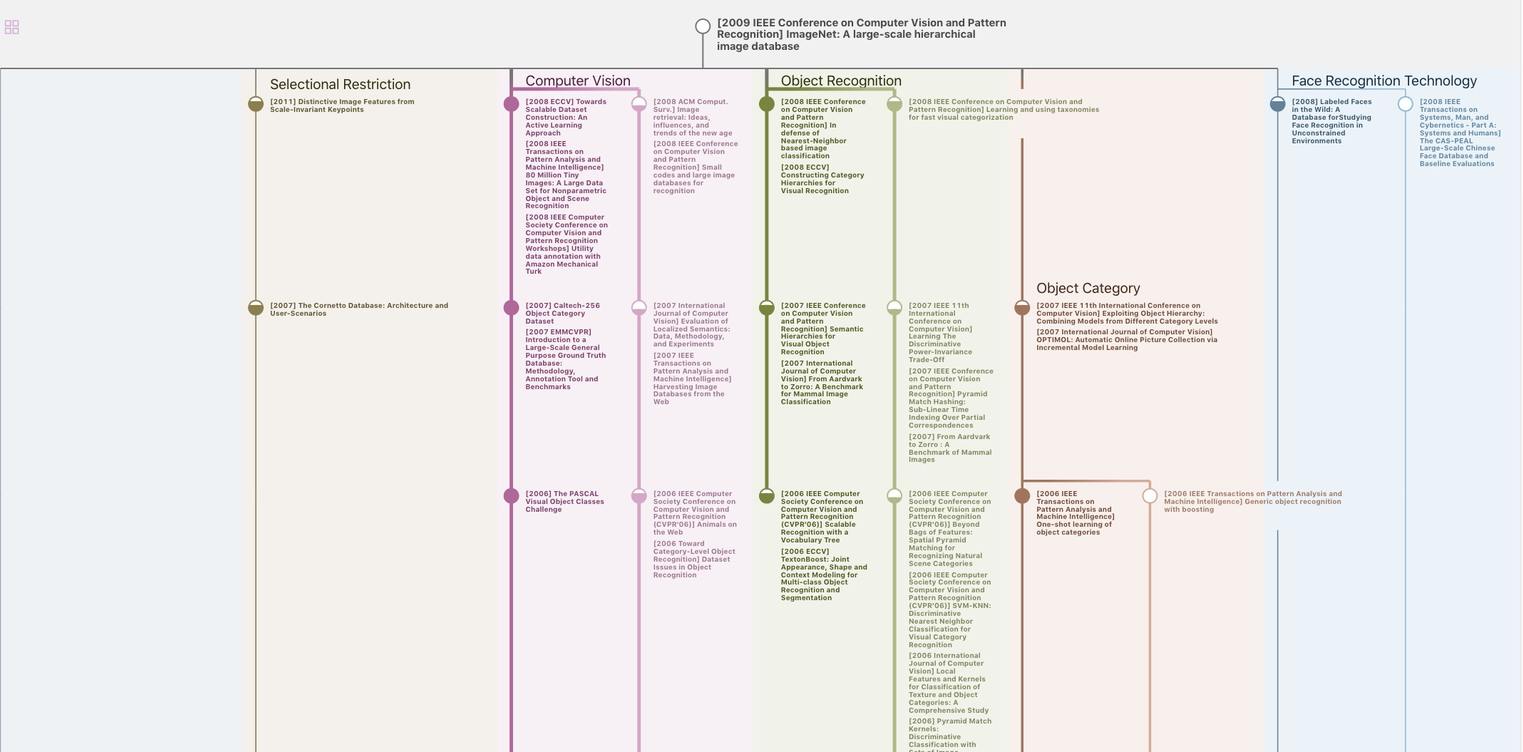
生成溯源树,研究论文发展脉络
Chat Paper
正在生成论文摘要