Privacy- and Utility-Preserving NLP with Anonymized Data: A case study of Pseudonymization
CoRR(2023)
摘要
This work investigates the effectiveness of different pseudonymization techniques, ranging from rule-based substitutions to using pre-trained Large Language Models (LLMs), on a variety of datasets and models used for two widely used NLP tasks: text classification and summarization. Our work provides crucial insights into the gaps between original and anonymized data (focusing on the pseudonymization technique) and model quality and fosters future research into higher-quality anonymization techniques to better balance the trade-offs between data protection and utility preservation. We make our code, pseudonymized datasets, and downstream models publicly available
更多查看译文
关键词
anonymized data,pseudonymization,nlp,utility-preserving
AI 理解论文
溯源树
样例
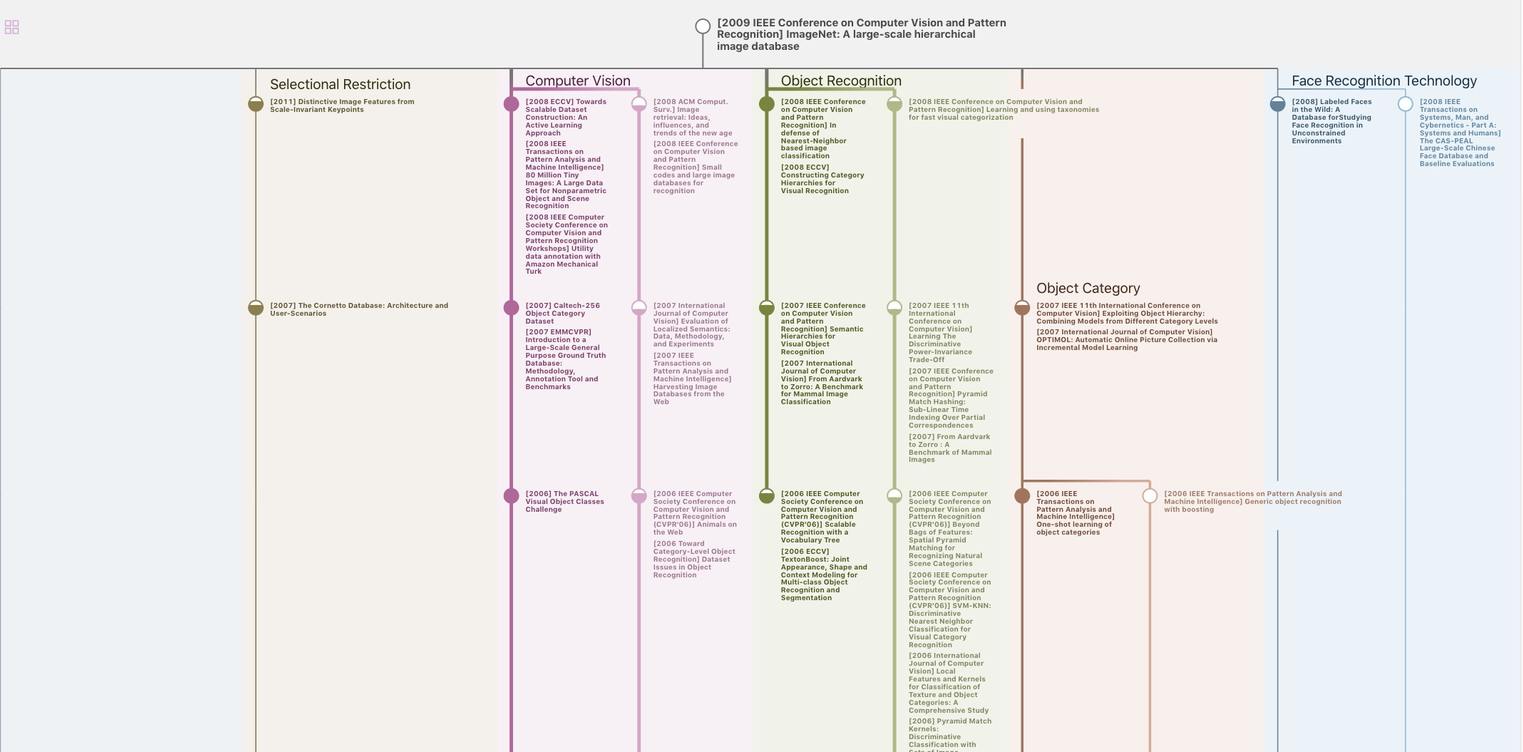
生成溯源树,研究论文发展脉络
Chat Paper
正在生成论文摘要