SMILE: A Cost-Effective System for Serving Massive Pretrained Language Models in The Cloud.
SIGMOD/PODS '23: Companion of the 2023 International Conference on Management of Data(2023)
摘要
Deep learning models, particularly pre-trained language models (PLMs), have become increasingly important for a variety of applications that require text/language processing. However, these models are resource-intensive and often require costly hardware such as dedicated GPU servers. In response to this issue, we present SMILE, a novel prototype system for efficient deployment and management of such models in the cloud. Our goal is to build a cloud platform from which tenants can easily derive their own custom models, and rent PLM processors to run inference services on these models at reduced costs. To facilitate this, we present a co-design of cost-effective storage and computation scheme for managing massive customized PLMs with constrained hardware resources via effective resource sharing and multiplexing. Our system consists of four core components: vPLM creator, vPLM storage appliance, vPLM trainer, and vPLM processor, which allow tenants to easily create, store, train, and use their customized PLM in the cloud without the need for dedicated hardware or maintenance. In particular, vPLM processors are virtualized from a physical machine, and are designed to have a multi-tenant nature, enabling efficient utilization of resources by precomputing the intermediate representation of PLMs and using adapters to provide customization instead of training the entire model. This allows tenants to host their PLMs in the cloud at minor costs. In our demonstration, we show that over 10,000 models can be hosted on one single machine without compromising the inference speed and accuracy. Overall, our system provides a convenient and cost-effective solution for tenants to host and manage PLMs in the cloud for their customized tasks.
更多查看译文
AI 理解论文
溯源树
样例
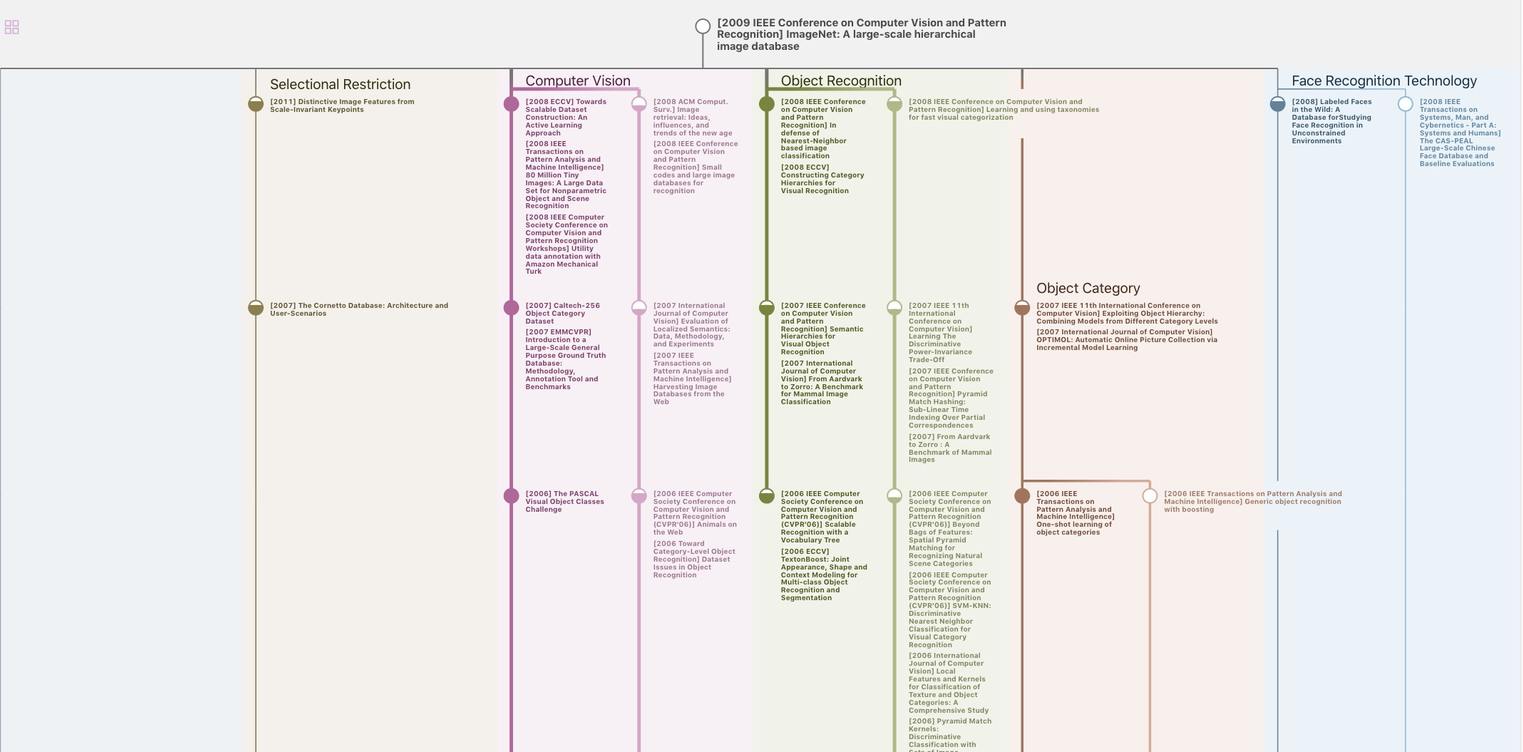
生成溯源树,研究论文发展脉络
Chat Paper
正在生成论文摘要