SIGMA-DF: Single-Side Guided Meta-Learning for Deepfake Detection
ICMR '23: Proceedings of the 2023 ACM International Conference on Multimedia Retrieval(2023)
摘要
The current challenge of Deepfake detection is the cross-domain performance on unseen Deepfake data. Instead of extracting forgery artifacts that are robust to the cross-domain scenarios as most previous works, we propose a novel method named Single-sIde Guided Meta-leArning framework for DeepFake detection (SIGMA-DF) which simulates the cross-domain scenarios during training by synthesizing virtual testing domain through meta-learning. In addition, SIGMA-DF integrates the meta-learning algorithm with a new ensemble meta-learning framework, which separately trains multiple meta-learners in the meta-train phase to aggregate multiple domain shifts in each iteration. Hence multiple cross-domain scenarios are simulated, better leveraging the domain knowledge. In addition, considering the contribution of hard samples in single-side distribution optimization, a novel weighted single-side loss function is proposed to only narrow the intra-class distance between real faces and enlarge the inter-class distance for both real and fake faces in embedding space with the awareness of sample weights. Extensive experiments are conducted on several standard Deepfake detection datasets to demonstrate that the proposed SIGMA-DF achieves state-of-the-art performance. In particular, in the cross-domain evaluation from FF++ to Celeb-DF and DFDC, our SIGMA-DF outperforms the baselines by 4.4% and 4.5% in terms of AUC, respectively.
更多查看译文
关键词
Deepfake Detection, Multimedia Forensics, Meta Learning, Domain Generalization
AI 理解论文
溯源树
样例
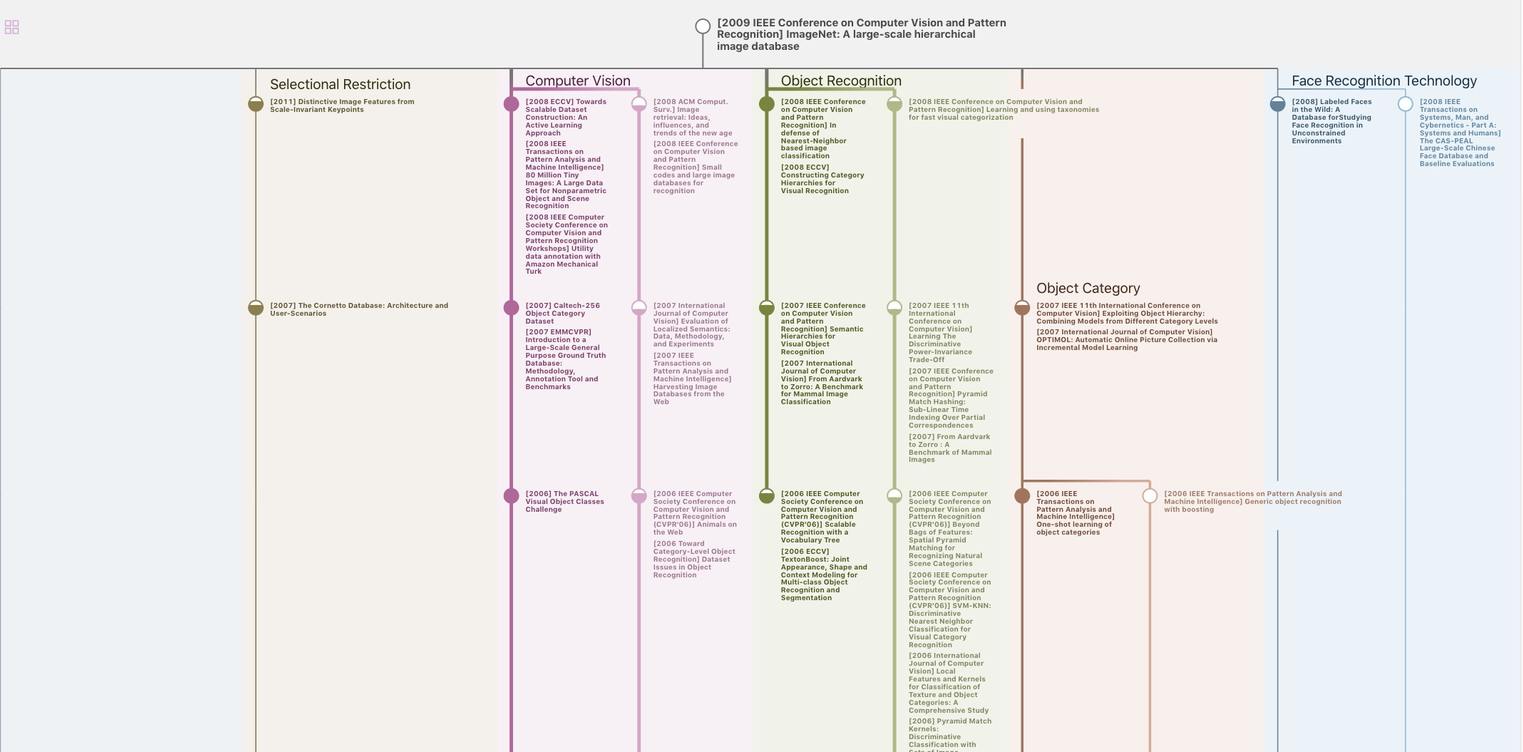
生成溯源树,研究论文发展脉络
Chat Paper
正在生成论文摘要