Graph Contrastive Learning on Complementary Embedding for Recommendation
ICMR '23: Proceedings of the 2023 ACM International Conference on Multimedia Retrieval(2023)
摘要
Previous works build interest learning via mining deeply on interactions. However, the interactions come incomplete and insufficient to support interest modeling, even bringing severe bias into recommendations. To address the interaction sparsity and the consequent bias challenges, we propose a graph contrastive learning on complementary embedding (GCCE), which introduces negative interests to assist positive interests of interactions for interest modeling. To embed interest, we design a perturbed graph convolution by preventing embedding distribution from bias. Since negative samples are not available in the general scenario of implicit feedback, we elaborate a complementary embedding generation to depict users’ negative interests. Finally, we develop a new contrastive task to contrastively learn from the positive and negative interests to promote recommendation. We validate the effectiveness of GCCE on two real datasets, where it outperforms the state-of-the-art models for recommendation.
更多查看译文
关键词
Recommender system, contrastive learning, graph neural network, user interest
AI 理解论文
溯源树
样例
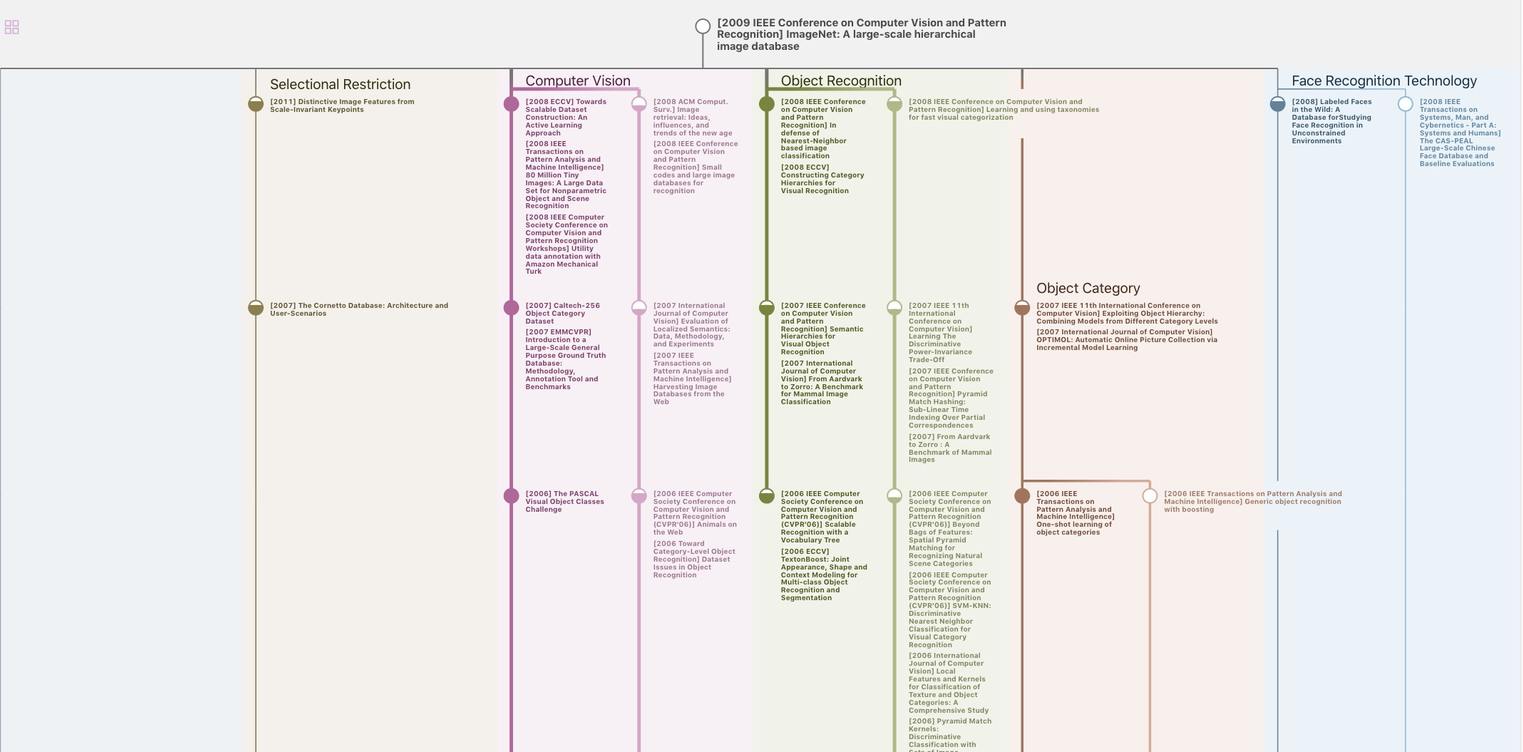
生成溯源树,研究论文发展脉络
Chat Paper
正在生成论文摘要