Label-wise Deep Semantic-Alignment Hashing for Cross-Modal Retrieval
ICMR '23: Proceedings of the 2023 ACM International Conference on Multimedia Retrieval(2023)
摘要
Hashing plays an important role in the content-based retrieval of multimedia data. Existing methods focus on designing various joint-optimization strategies to preserve the similarity relationships between different modalities and improve the performance of cross-modal retrieval. However, when the intrinsic information of any modality is significantly insufficient compared with others, the final hash space will collapse to the trivial space due to the joint training. Compared with inter-modal semantic alignment, directly aligning the semantics of each modality with the label-wise semantics can obtain higher-quality common semantic spaces, and only a pair-wise alignment between each space can obtain a unified representation. Following this idea, we design a general hash generation framework for uni-modal embedding and directly align the hashing code from different modalities using a pair-wise loss, further improving the retrieval performance within the less-semantic modality. Within this framework, we introduce two optimizations to penalize dissimilar items. First, with a Gaussian distribution to describe the hashing semantic distribution, JS-divergence is introduced to keep the consistency of label-wise semantics and hashing similarity. Then the attention mechanism is used for hard-sample re-weighting to learn fine-grained distribution alignment further. We conduct extensive experiments on three public datasets to validate the enhancements of our work.
更多查看译文
关键词
Cross-Modal Retrieval, Cross-Modal Hashing, Label-Wise Semantic Alignment
AI 理解论文
溯源树
样例
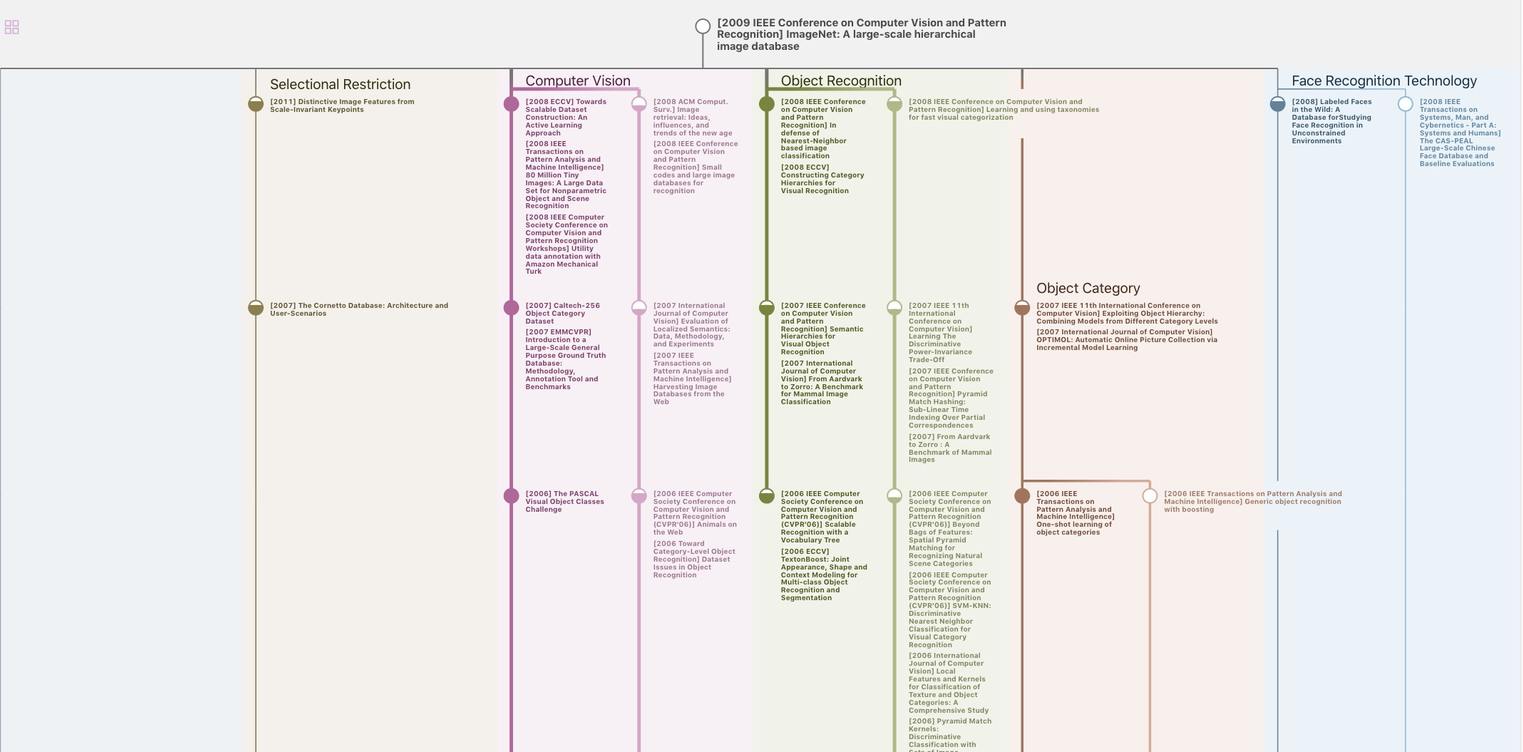
生成溯源树,研究论文发展脉络
Chat Paper
正在生成论文摘要