Predicting Dynamic Properties of Heap Allocations using Neural Networks Trained on Static Code An Intellectual Abstract
PROCEEDINGS OF THE 2023 ACM SIGPLAN INTERNATIONAL SYMPOSIUM ON MEMORY MANAGEMENT, ISMM 2023(2023)
摘要
Memory allocators and runtime systems can leverage dynamic properties of heap allocations - such as object lifetimes, hotness or access correlations - to improve performance and resource consumption. A significant amount of work has focused on approaches that collect this information in performance profiles and then use it in new memory allocator or runtime designs, both offline (e.g., in ahead-of-time compilers) and online (e.g., in JIT compilers). This is a special instance of profile-guided optimization. This approach introduces significant challenges: 1) The profiling oftentimes introduces substantial overheads, which are prohibitive in many production scenarios, 2) Creating a representative profiling run adds significant engineering complexity and reduces deployment velocity, and 3) Profiles gathered ahead of time or during the warm-up phase of a server are often not representative of all workload behavior and may miss important corner cases. In this paper, we investigate a fundamentally different approach. Instead of deriving heap allocation properties from profiles, we explore the ability of neural network models to predict them from the statically available code. As an intellectual abstract, we do not offer a conclusive answer but describe the trade-off space of this approach, investigate promising directions, motivate these directions with data analysis and experiments, and highlight challenges that future work needs to overcome.
更多查看译文
关键词
Machine Learning,Profile-guided Optimization,Lifetime Prediction,Memory Management
AI 理解论文
溯源树
样例
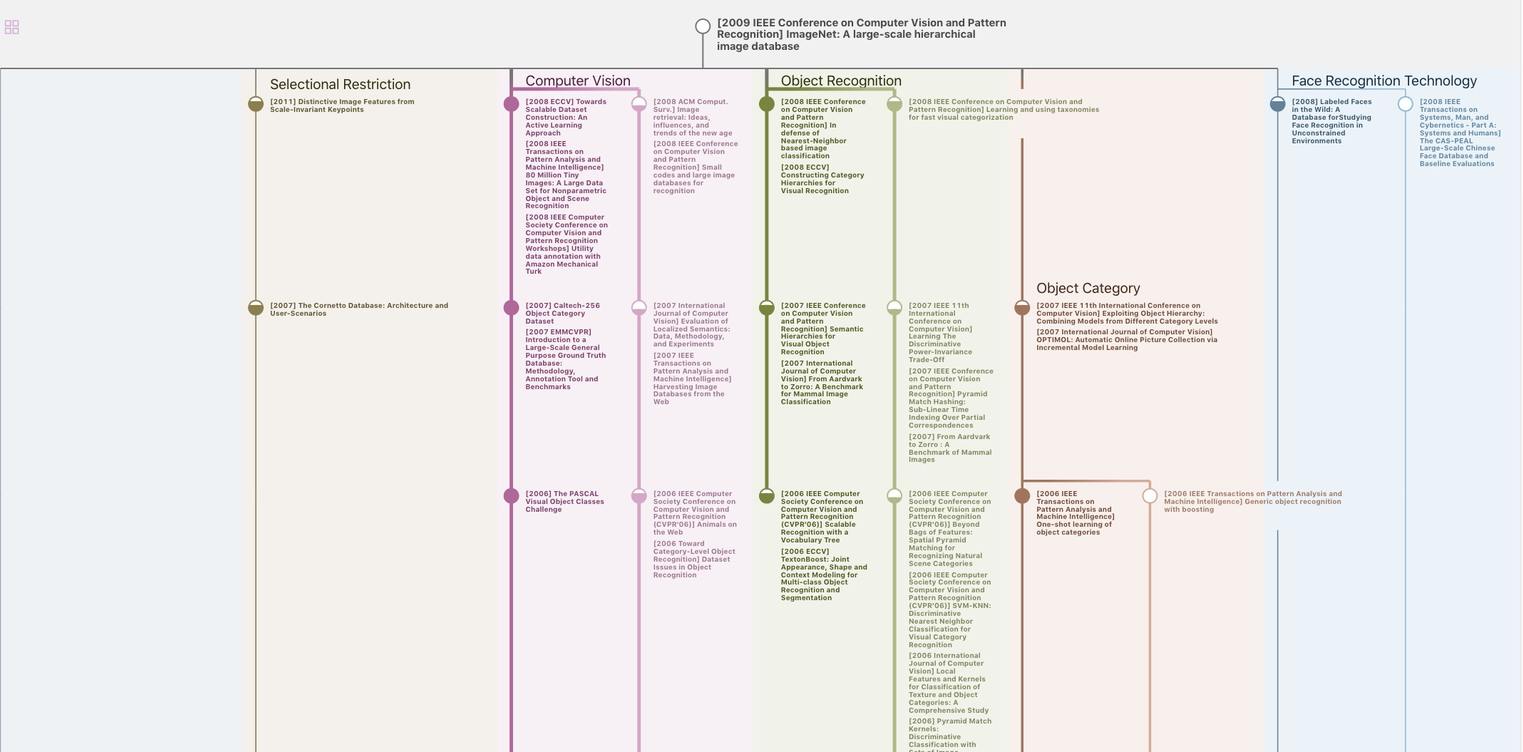
生成溯源树,研究论文发展脉络
Chat Paper
正在生成论文摘要