CUED at ProbSum 2023: Hierarchical Ensemble of Summarization Models
conf_acl(2023)
摘要
In this paper, we consider the challenge of summarizing patients' medical progress notes in a limited data setting. For the Problem List Summarization (shared task 1A) at the BioNLP Workshop 2023, we demonstrate that Clinical-T5 fine-tuned to 765 medical clinic notes outperforms other extractive, abstractive and zero-shot baselines, yielding reasonable baseline systems for medical note summarization. Further, we introduce Hierarchical Ensemble of Summarization Models (HESM), consisting of token-level ensembles of diverse fine-tuned Clinical-T5 models, followed by Minimum Bayes Risk (MBR) decoding. Our HESM approach lead to a considerable summarization performance boost, and when evaluated on held-out challenge data achieved a ROUGE-L of 32.77, which was the best-performing system at the top of the shared task leaderboard.
更多查看译文
关键词
hierarchical ensemble,probsum,models
AI 理解论文
溯源树
样例
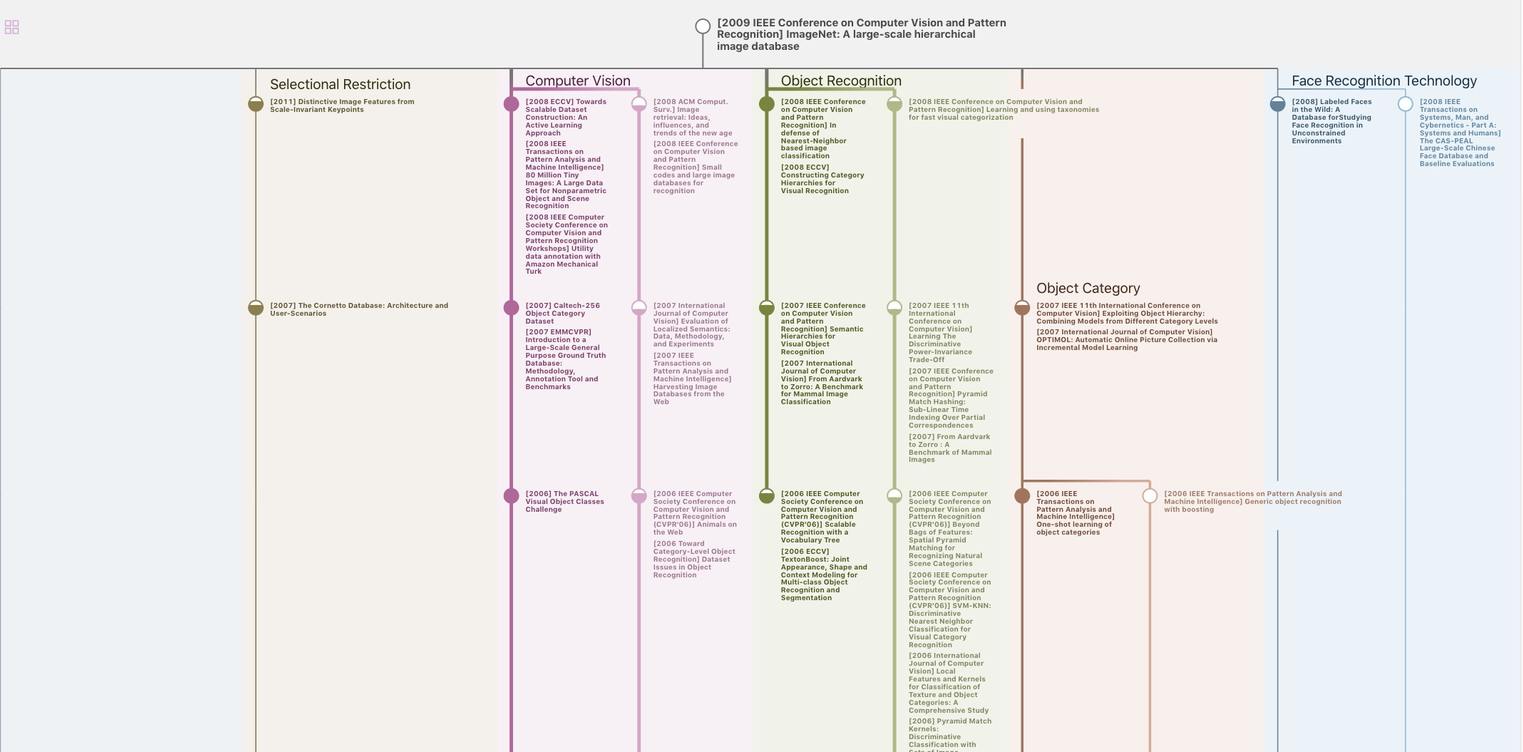
生成溯源树,研究论文发展脉络
Chat Paper
正在生成论文摘要