Computing Optimal Equilibria and Mechanisms via Learning in Zero-Sum Extensive-Form Games
arXiv (Cornell University)(2023)
摘要
We introduce a new approach for computing optimal equilibria via learning in games. It applies to extensive-form settings with any number of players, including mechanism design, information design, and solution concepts such as correlated, communication, and certification equilibria. We observe that optimal equilibria are minimax equilibrium strategies of a player in an extensive-form zero-sum game. This reformulation allows to apply techniques for learning in zero-sum games, yielding the first learning dynamics that converge to optimal equilibria, not only in empirical averages, but also in iterates. We demonstrate the practical scalability and flexibility of our approach by attaining state-of-the-art performance in benchmark tabular games, and by computing an optimal mechanism for a sequential auction design problem using deep reinforcement learning.
更多查看译文
关键词
learning,zero-sum,extensive-form
AI 理解论文
溯源树
样例
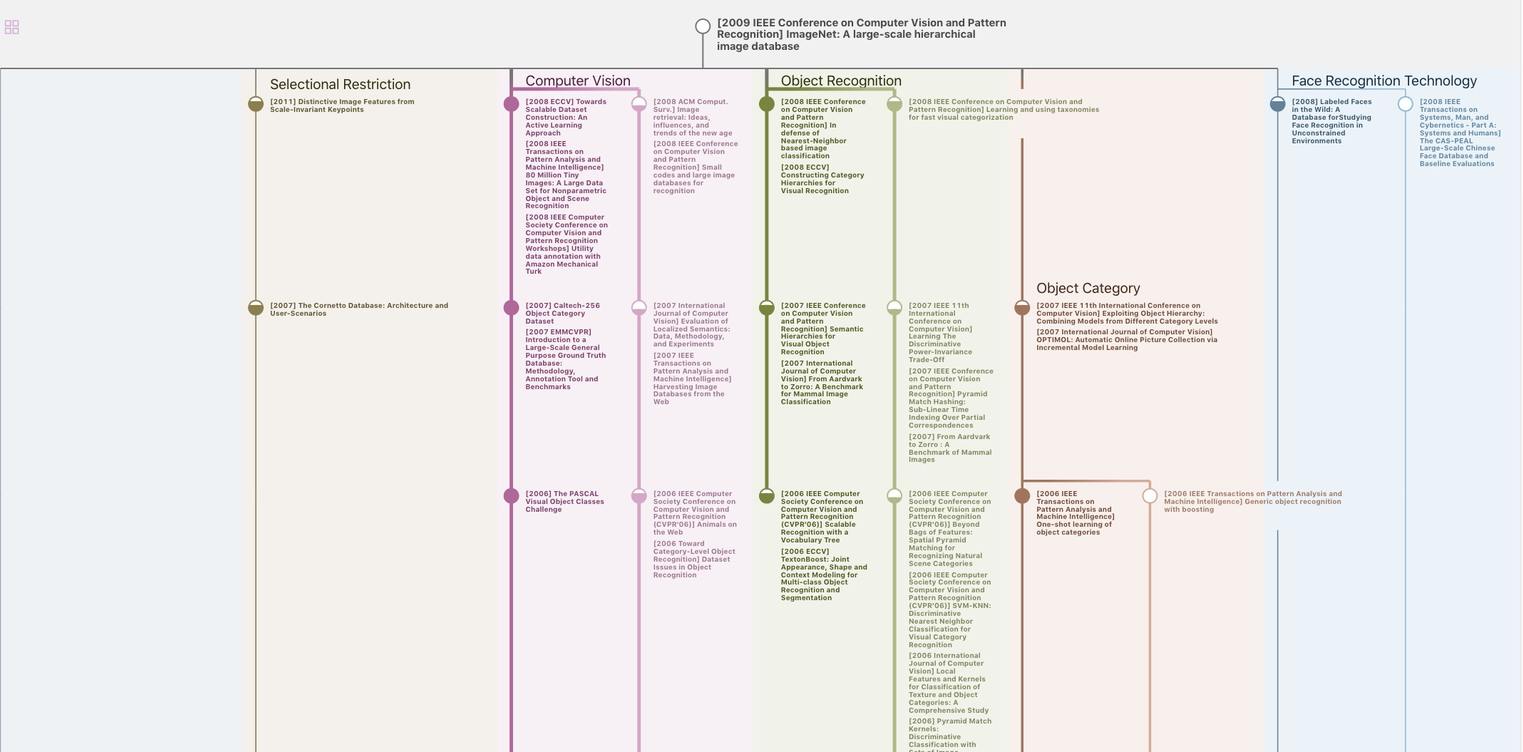
生成溯源树,研究论文发展脉络
Chat Paper
正在生成论文摘要