Efficient and Equivariant Graph Networks for Predicting Quantum Hamiltonian
CoRR(2023)
摘要
We consider the prediction of the Hamiltonian matrix, which finds use in quantum chemistry and condensed matter physics. Efficiency and equivariance are two important, but conflicting factors. In this work, we propose a SE(3)-equivariant network, named QHNet, that achieves efficiency and equivariance. Our key advance lies at the innovative design of QHNet architecture, which not only obeys the underlying symmetries, but also enables the reduction of number of tensor products by 92\%. In addition, QHNet prevents the exponential growth of channel dimension when more atom types are involved. We perform experiments on MD17 datasets, including four molecular systems. Experimental results show that our QHNet can achieve comparable performance to the state of the art methods at a significantly faster speed. Besides, our QHNet consumes 50\% less memory due to its streamlined architecture. Our code is publicly available as part of the AIRS library (\url{https://github.com/divelab/AIRS}).
更多查看译文
关键词
equivariant graph networks,quantum
AI 理解论文
溯源树
样例
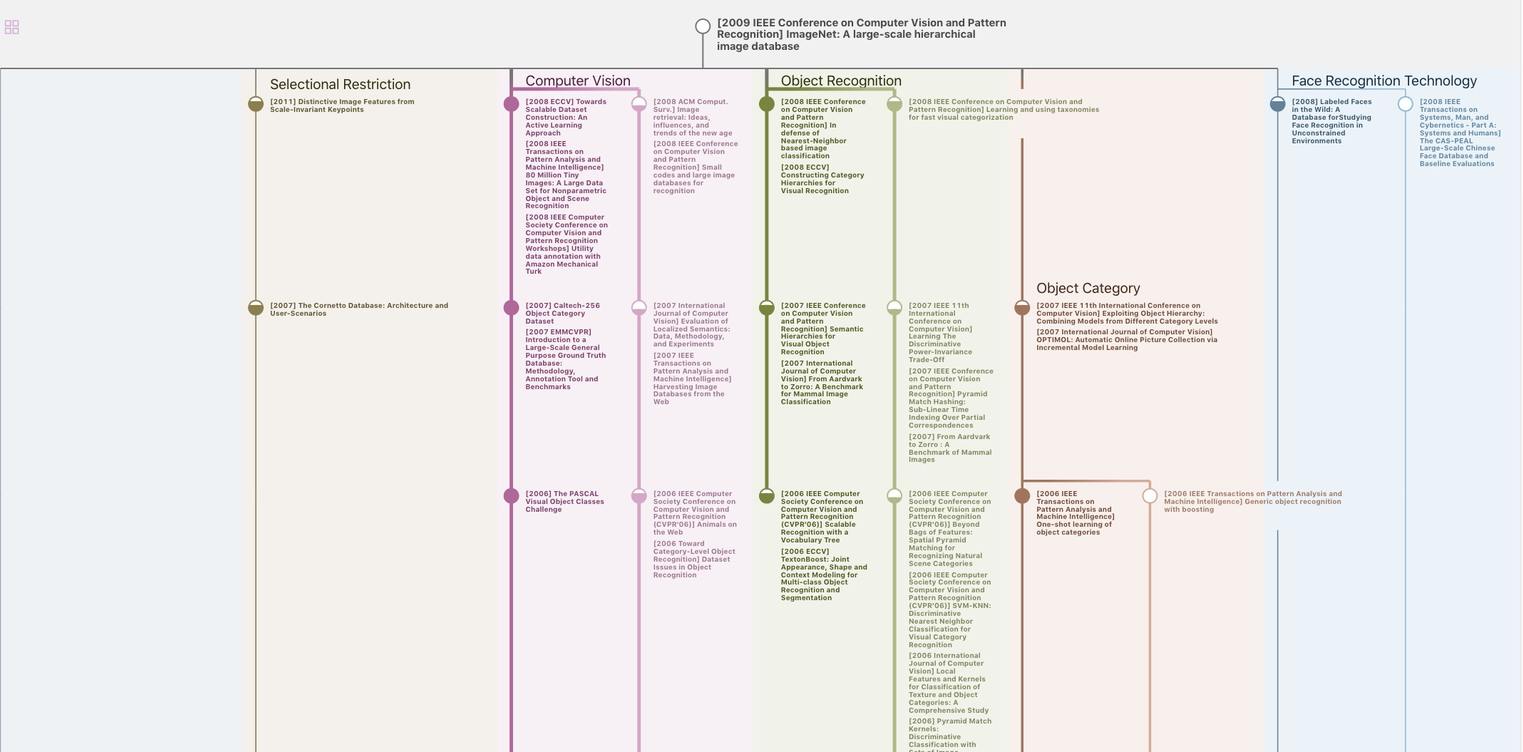
生成溯源树,研究论文发展脉络
Chat Paper
正在生成论文摘要