Invariant Causal Set Covering Machines
CoRR(2023)
摘要
Rule-based models, such as decision trees, appeal to practitioners due to their interpretable nature. However, the learning algorithms that produce such models are often vulnerable to spurious associations and thus, they are not guaranteed to extract causally-relevant insights. In this work, we build on ideas from the invariant causal prediction literature to propose Invariant Causal Set Covering Machines, an extension of the classical Set Covering Machine algorithm for conjunctions/disjunctions of binary-valued rules that provably avoids spurious associations. We demonstrate both theoretically and empirically that our method can identify the causal parents of a variable of interest in polynomial time.
更多查看译文
关键词
set
AI 理解论文
溯源树
样例
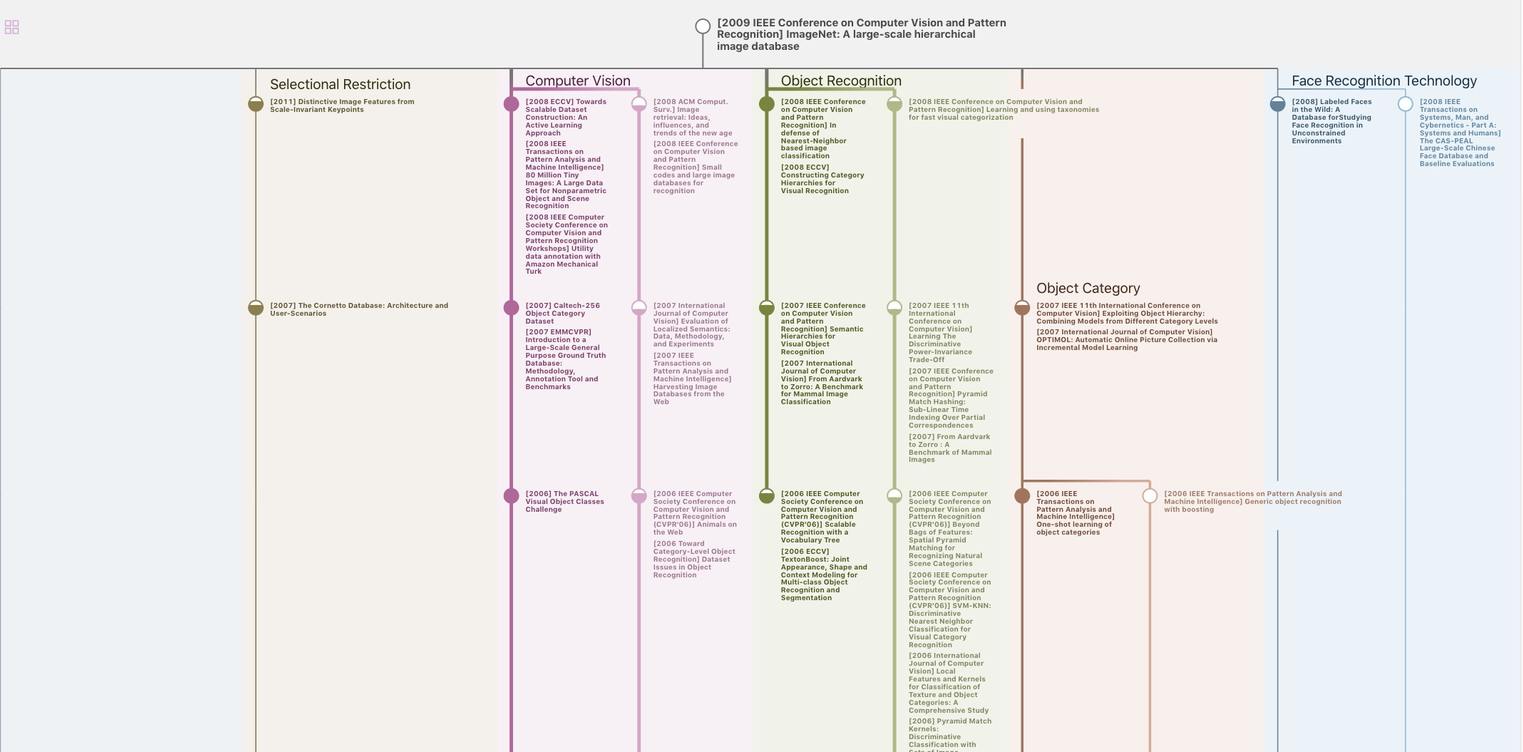
生成溯源树,研究论文发展脉络
Chat Paper
正在生成论文摘要