基于PCA-CNN-BiLSTM的锂离子电池剩余使用寿命预测
Journal of Jiamusi University(Natural Science Edition)(2023)
Abstract
准确预测锂电池剩余使用寿命(Remaining Useful Life,RUL)对电池状态和管理的估计具有重要的意义.针对传统方法的预测误差大、训练时间长及易受环境影响等不足,提出一种混合神经网络,即卷积神经网络(Convolutional Neural Networks,CNN)和双向长短期记忆(Bi-directional Long Short-Term Memory,BiLSTM),旨在寻找影响电池退化几个变量之间的层次特征,挖掘数据中深层次的信息.为去除冗余信息和提取特征变量引入主成分分析方法(Principal Component Analysis,PCA),以便训练混合神经网络.通过在NASA公开锂电池的数据集上测试,并与现有的LSTM,BiLSTM和CNN-LSTM模型比较.结果表明,相比LSTM,BILSTM和CNN-LSTM,PCA-CNN-BiLSTM模型预测准确率更高,达到99.07%,可以实现对容量轨迹和RUL的准确预测.
MoreTranslated text
Key words
li-ion batteries,bidirectional long short-term memory,convolutional neural network,principal component analysis,remaining useful life prediction
AI Read Science
Must-Reading Tree
Example
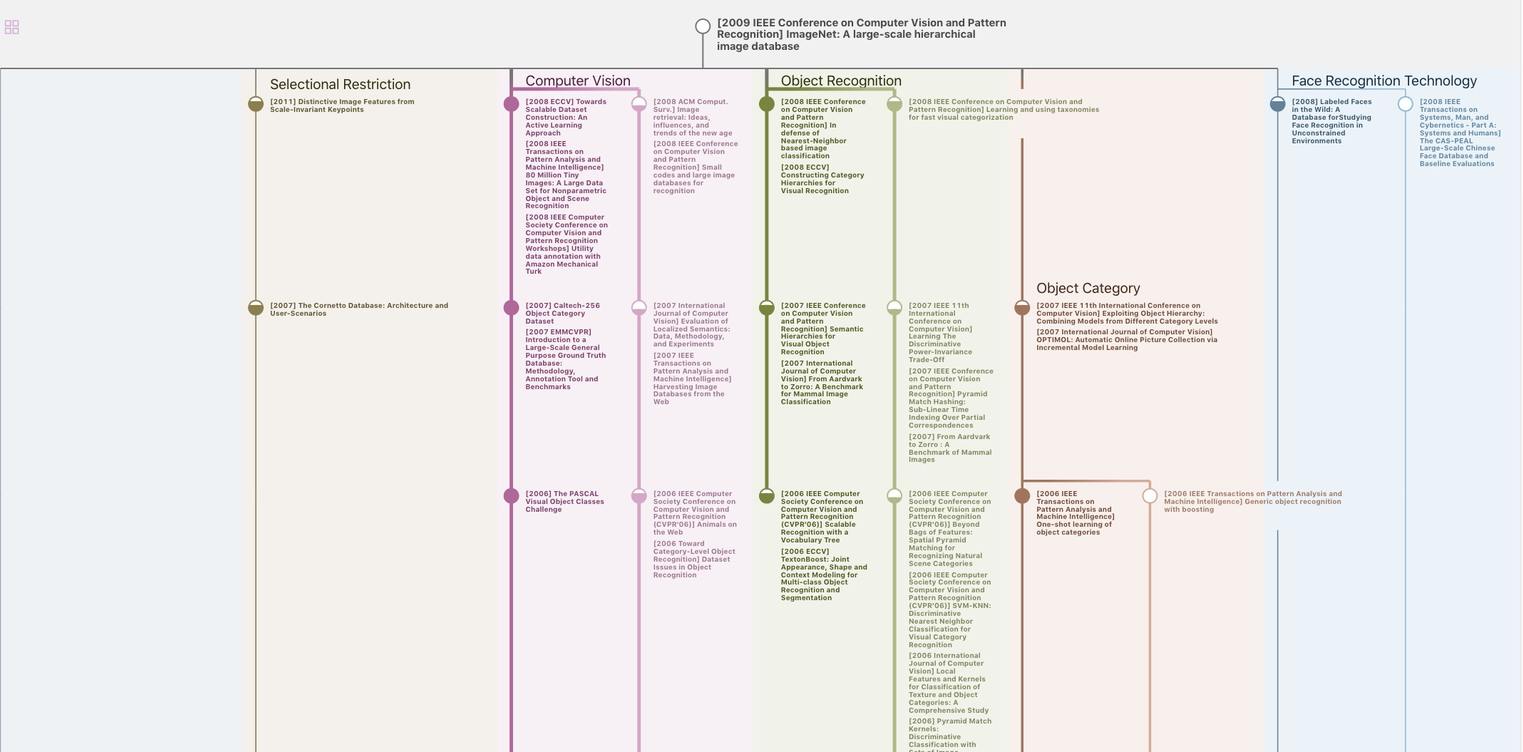
Generate MRT to find the research sequence of this paper
Chat Paper
Summary is being generated by the instructions you defined