From dense to sparse design: Optimal rates under the supremum norm for estimating the mean function in functional data analysis
arxiv(2023)
摘要
We derive optimal rates of convergence in the supremum norm for estimating
the Hölder-smooth mean function of a stochastic process which is repeatedly
and discretely observed with additional errors at fixed, multivariate,
synchronous design points, the typical scenario for machine recorded functional
data. Similarly to the optimal rates in L_2 obtained in
, for sparse design a discretization term dominates,
while in the dense case the parametric √(n) rate can be achieved as if the
n processes were continuously observed without errors. The supremum norm is
of practical interest since it corresponds to the visualization of the
estimation error, and forms the basis for the construction uniform confidence
bands. We show that in contrast to the analysis in L_2, there is an
intermediate regime between the sparse and dense cases dominated by the
contribution of the observation errors. Furthermore, under the supremum norm
interpolation estimators which suffice in L_2 turn out to be sub-optimal in
the dense setting, which helps to explain their poor empirical performance. In
contrast to previous contributions involving the supremum norm, we discuss
optimality even in the multivariate setting, and for dense design obtain the
√(n) rate of convergence without additional logarithmic factors. We also
obtain a central limit theorem in the supremum norm, and provide simulations
and real data applications to illustrate our results.
更多查看译文
AI 理解论文
溯源树
样例
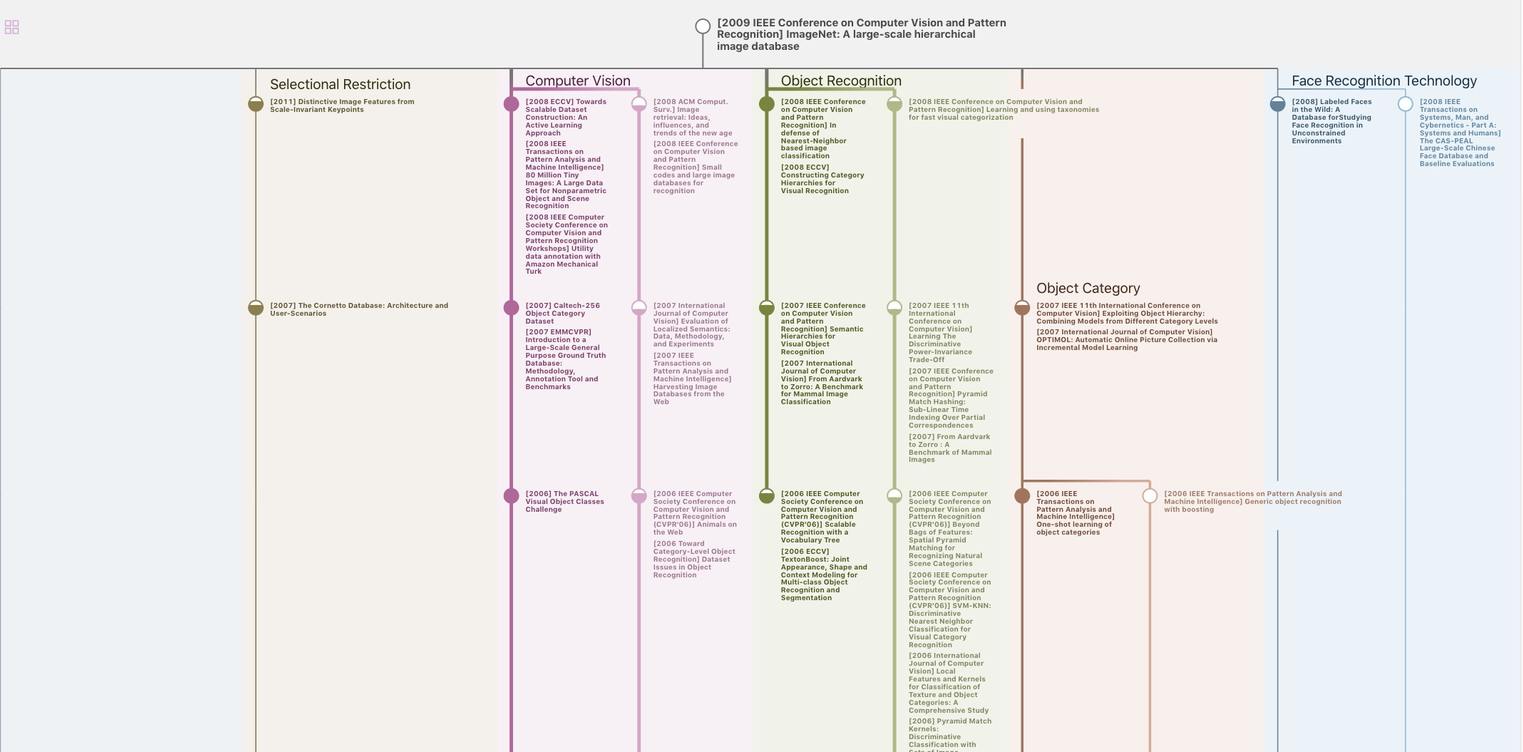
生成溯源树,研究论文发展脉络
Chat Paper
正在生成论文摘要