Long Sequence Hopfield Memory
arXiv (Cornell University)(2023)
摘要
Sequence memory is an essential attribute of natural and artificial intelligence that enables agents to encode, store, and retrieve complex sequences of stimuli and actions. Computational models of sequence memory have been proposed where recurrent Hopfield-like neural networks are trained with temporally asymmetric Hebbian rules. However, these networks suffer from limited sequence capacity (maximal length of the stored sequence) due to interference between the memories. Inspired by recent work on Dense Associative Memories, we expand the sequence capacity of these models by introducing a nonlinear interaction term, enhancing separation between the patterns. We derive novel scaling laws for sequence capacity with respect to network size, significantly outperforming existing scaling laws for models based on traditional Hopfield networks, and verify these theoretical results with numerical simulation. Moreover, we introduce a generalized pseudoinverse rule to recall sequences of highly correlated patterns. Finally, we extend this model to store sequences with variable timing between states' transitions and describe a biologically-plausible implementation, with connections to motor neuroscience.
更多查看译文
关键词
memory,sequence
AI 理解论文
溯源树
样例
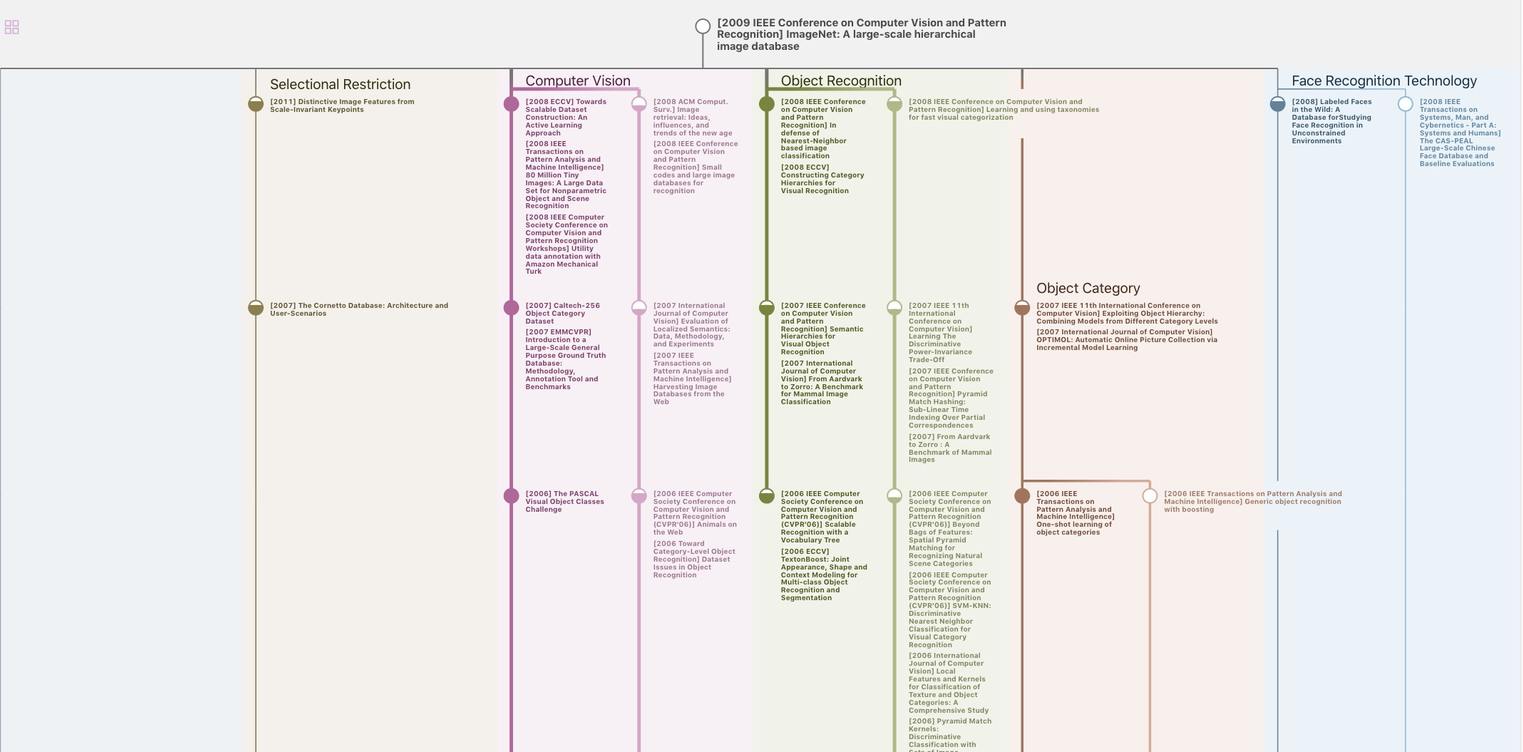
生成溯源树,研究论文发展脉络
Chat Paper
正在生成论文摘要