ICON$^2$: Reliably Benchmarking Predictive Inequity in Object Detection
CoRR(2023)
摘要
As computer vision systems are being increasingly deployed at scale in high-stakes applications like autonomous driving, concerns about social bias in these systems are rising. Analysis of fairness in real-world vision systems, such as object detection in driving scenes, has been limited to observing predictive inequity across attributes such as pedestrian skin tone, and lacks a consistent methodology to disentangle the role of confounding variables e.g. does my model perform worse for a certain skin tone, or are such scenes in my dataset more challenging due to occlusion and crowds? In this work, we introduce ICON$^2$, a framework for robustly answering this question. ICON$^2$ leverages prior knowledge on the deficiencies of object detection systems to identify performance discrepancies across sub-populations, compute correlations between these potential confounders and a given sensitive attribute, and control for the most likely confounders to obtain a more reliable estimate of model bias. Using our approach, we conduct an in-depth study on the performance of object detection with respect to income from the BDD100K driving dataset, revealing useful insights.
更多查看译文
关键词
detection,predictive inequity,object,reliably
AI 理解论文
溯源树
样例
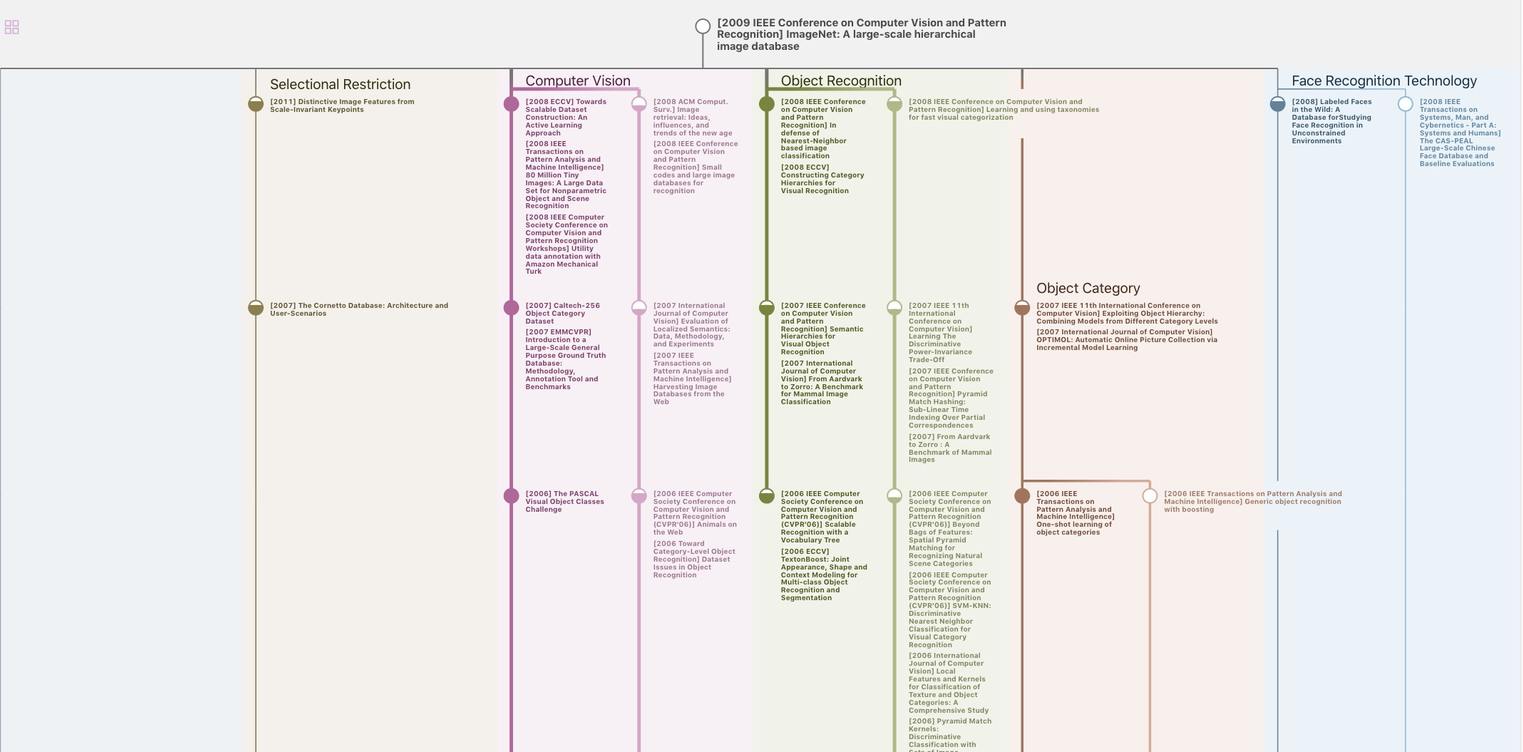
生成溯源树,研究论文发展脉络
Chat Paper
正在生成论文摘要