Accounting For Informative Sampling When Learning to Forecast Treatment Outcomes Over Time
CoRR(2023)
摘要
Machine learning (ML) holds great potential for accurately forecasting treatment outcomes over time, which could ultimately enable the adoption of more individualized treatment strategies in many practical applications. However, a significant challenge that has been largely overlooked by the ML literature on this topic is the presence of informative sampling in observational data. When instances are observed irregularly over time, sampling times are typically not random, but rather informative -- depending on the instance's characteristics, past outcomes, and administered treatments. In this work, we formalize informative sampling as a covariate shift problem and show that it can prohibit accurate estimation of treatment outcomes if not properly accounted for. To overcome this challenge, we present a general framework for learning treatment outcomes in the presence of informative sampling using inverse intensity-weighting, and propose a novel method, TESAR-CDE, that instantiates this framework using Neural CDEs. Using a simulation environment based on a clinical use case, we demonstrate the effectiveness of our approach in learning under informative sampling.
更多查看译文
关键词
forecast treatment
AI 理解论文
溯源树
样例
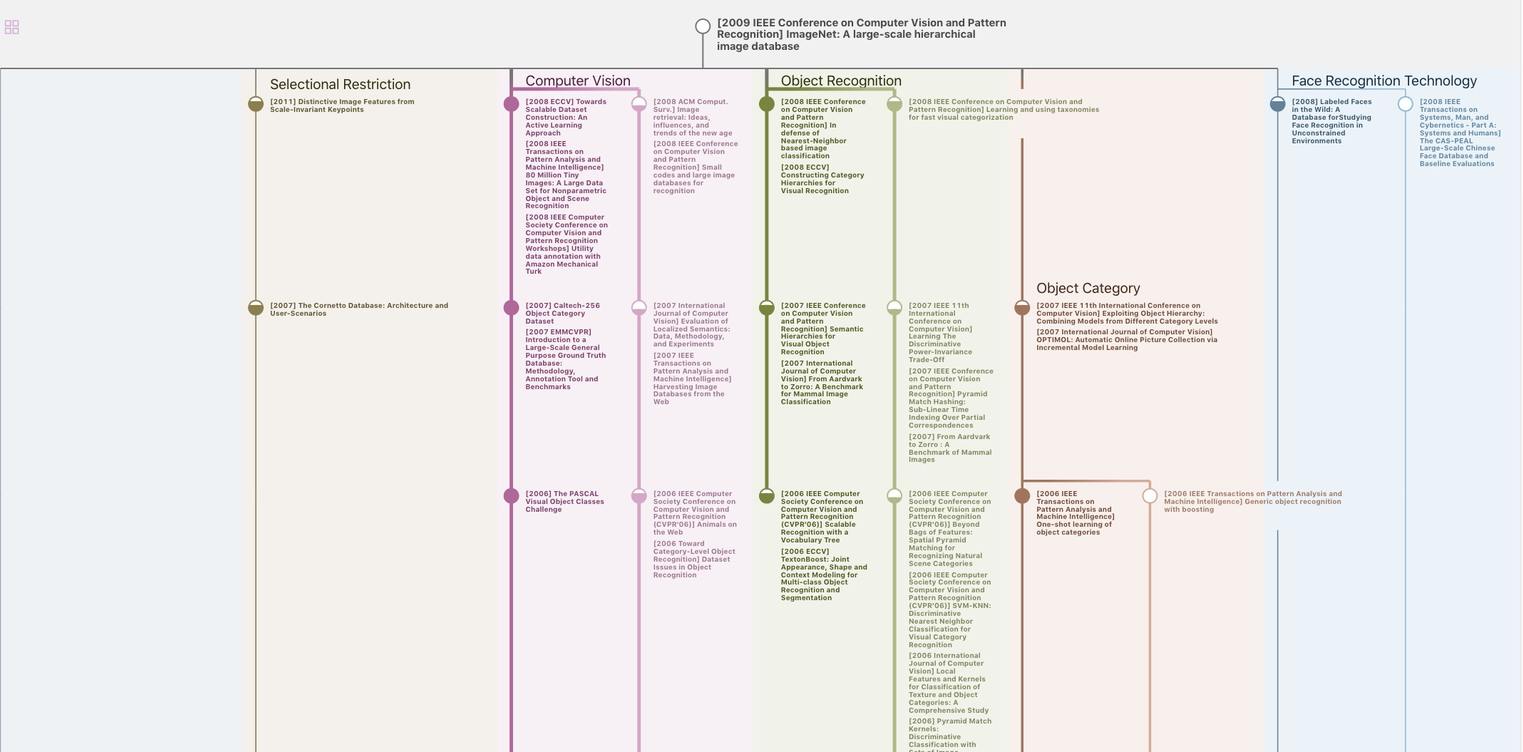
生成溯源树,研究论文发展脉络
Chat Paper
正在生成论文摘要