PILLAR: How to make semi-private learning more effective
CoRR(2023)
摘要
In Semi-Supervised Semi-Private (SP) learning, the learner has access to both public unlabelled and private labelled data. We propose a computationally efficient algorithm that, under mild assumptions on the data, provably achieves significantly lower private labelled sample complexity and can be efficiently run on real-world datasets. For this purpose, we leverage the features extracted by networks pre-trained on public (labelled or unlabelled) data, whose distribution can significantly differ from the one on which SP learning is performed. To validate its empirical effectiveness, we propose a wide variety of experiments under tight privacy constraints (\(\epsilon=0.1\)) and with a focus on low-data regimes. In all of these settings, our algorithm exhibits significantly improved performance over available baselines that use similar amounts of public data.
更多查看译文
关键词
learning,semi-private
AI 理解论文
溯源树
样例
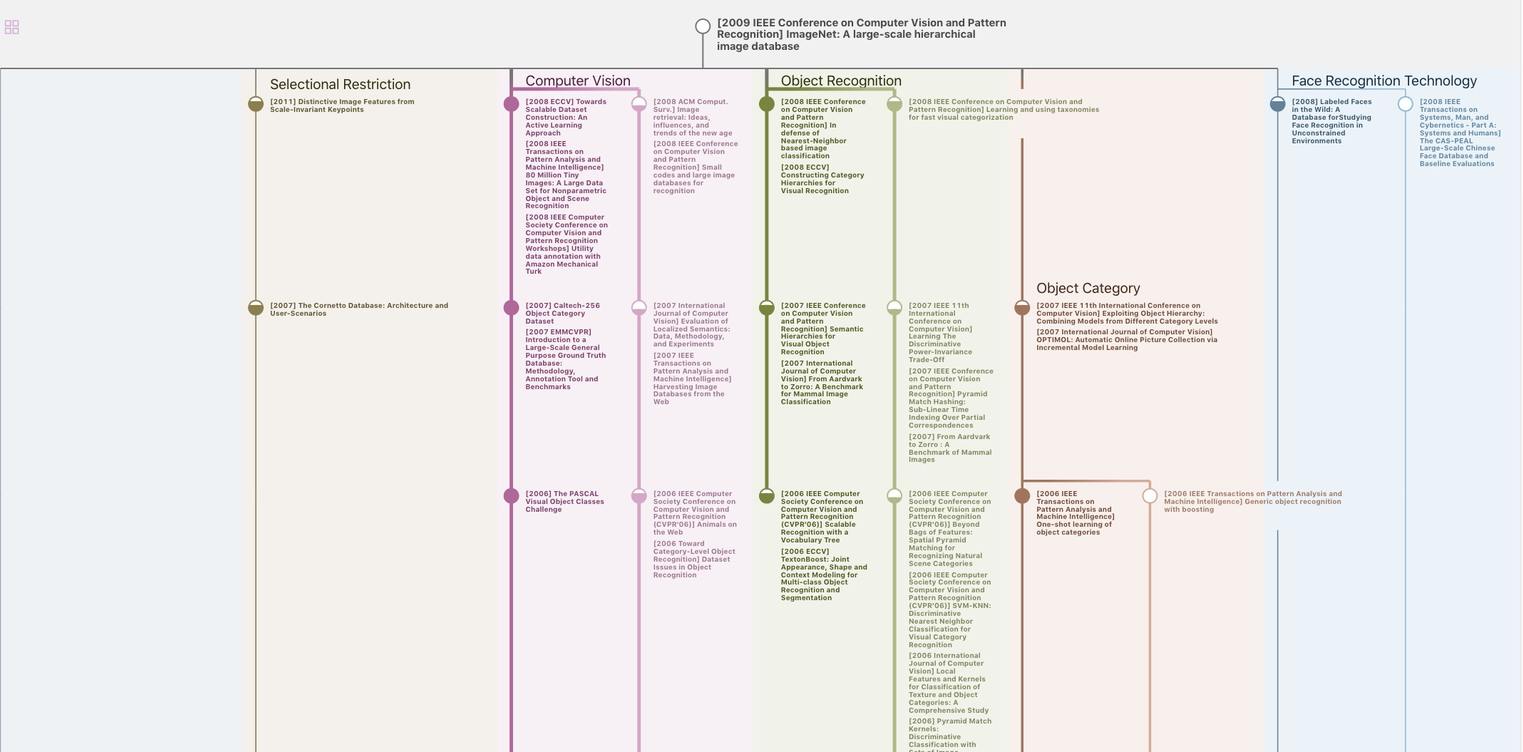
生成溯源树,研究论文发展脉络
Chat Paper
正在生成论文摘要