High-dimensional and permutation invariant anomaly detection
SCIPOST PHYSICS(2024)
摘要
Methods for anomaly detection of new physics processes are often limited to lowdimensional spaces due to the difficulty of learning high -dimensional probability densities. Particularly at the constituent level, incorporating desirable properties such as permutation invariance and variable -length inputs becomes difficult within popular density estimation methods. In this work, we introduce a permutation -invariant density estimator for particle physics data based on diffusion models, specifically designed to handle variable -length inputs. We demonstrate the efficacy of our methodology by utilizing the learned density as a permutation -invariant anomaly detection score, effectively identifying jets with low likelihood under the background -only hypothesis. To validate our density estimation method, we investigate the ratio of learned densities and compare to those obtained by a supervised classification algorithm.
更多查看译文
AI 理解论文
溯源树
样例
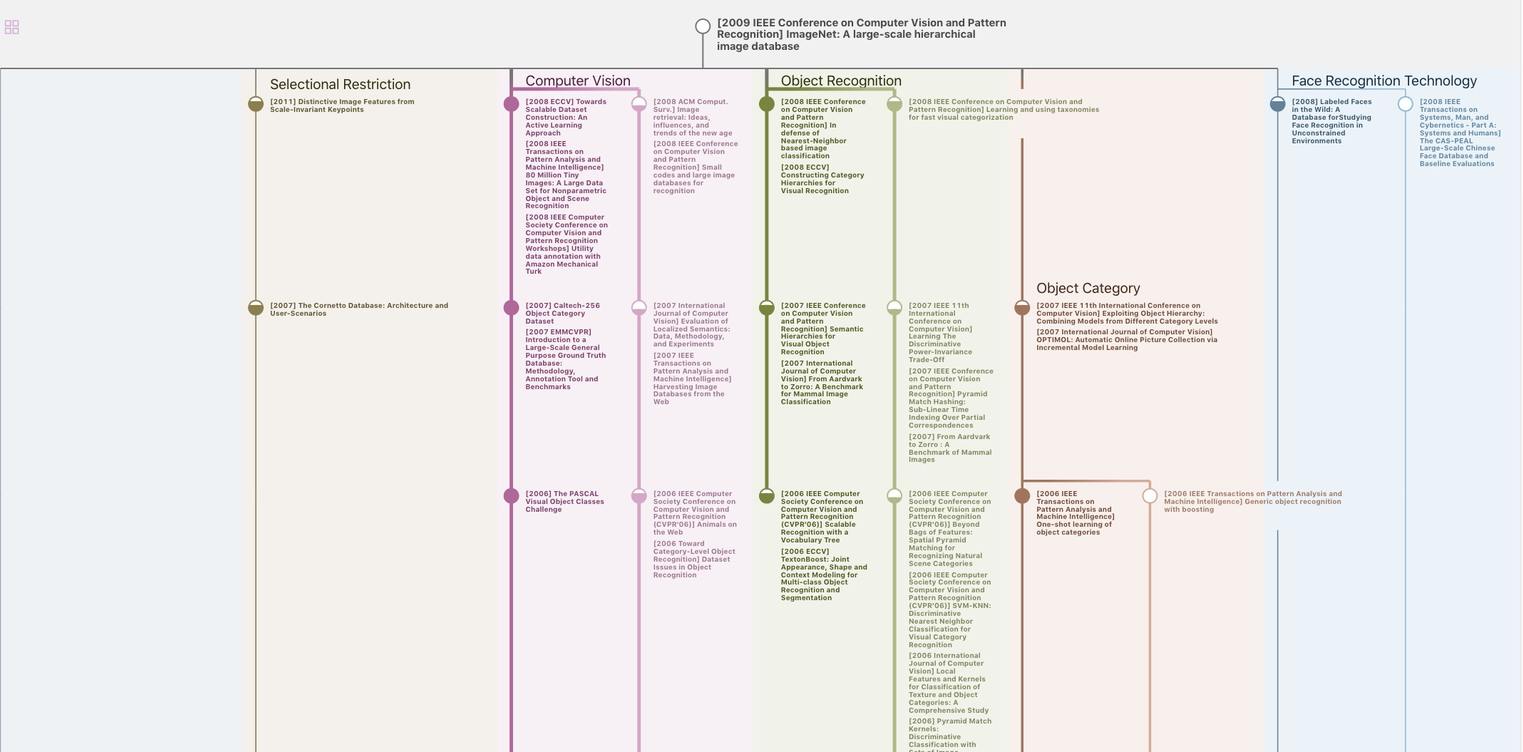
生成溯源树,研究论文发展脉络
Chat Paper
正在生成论文摘要