A Comparative Study of Forecasting Problems on Electrical Load Timeseries Data using Deep Learning Techniques
2023 IEEE/IAS 59th Industrial and Commercial Power Systems Technical Conference (I&CPS)(2023)
Abstract
This work presents a comparative study of forecasting problems on the Austria country electrical timeseries load demand data using deep learning models ANN-MLP, RNN-LSTM, and ID-CNN for short-term and medium-term electrical load forecast using different timestamps data and found that single timestamp ahead prediction (STAP) using MLP and multiple timestamps ahead prediction (MTAP) using ID-CNN show better results with reduced MAPE. ID-CNN is selected as the best model for both short-term 1-day ahead load forecast using 1-hour timestamp data with MTAP methodology resulting in MAPE 4.62% and for mid-term 1-year ahead load forecast using 1-month timestamp data with STAF methodology resulting in MAPE 1.45%.
MoreTranslated text
Key words
Artificial neural networks,convolutional neural networks,deep learning,long short-term memory,load forecasting,time series forecasting
AI Read Science
Must-Reading Tree
Example
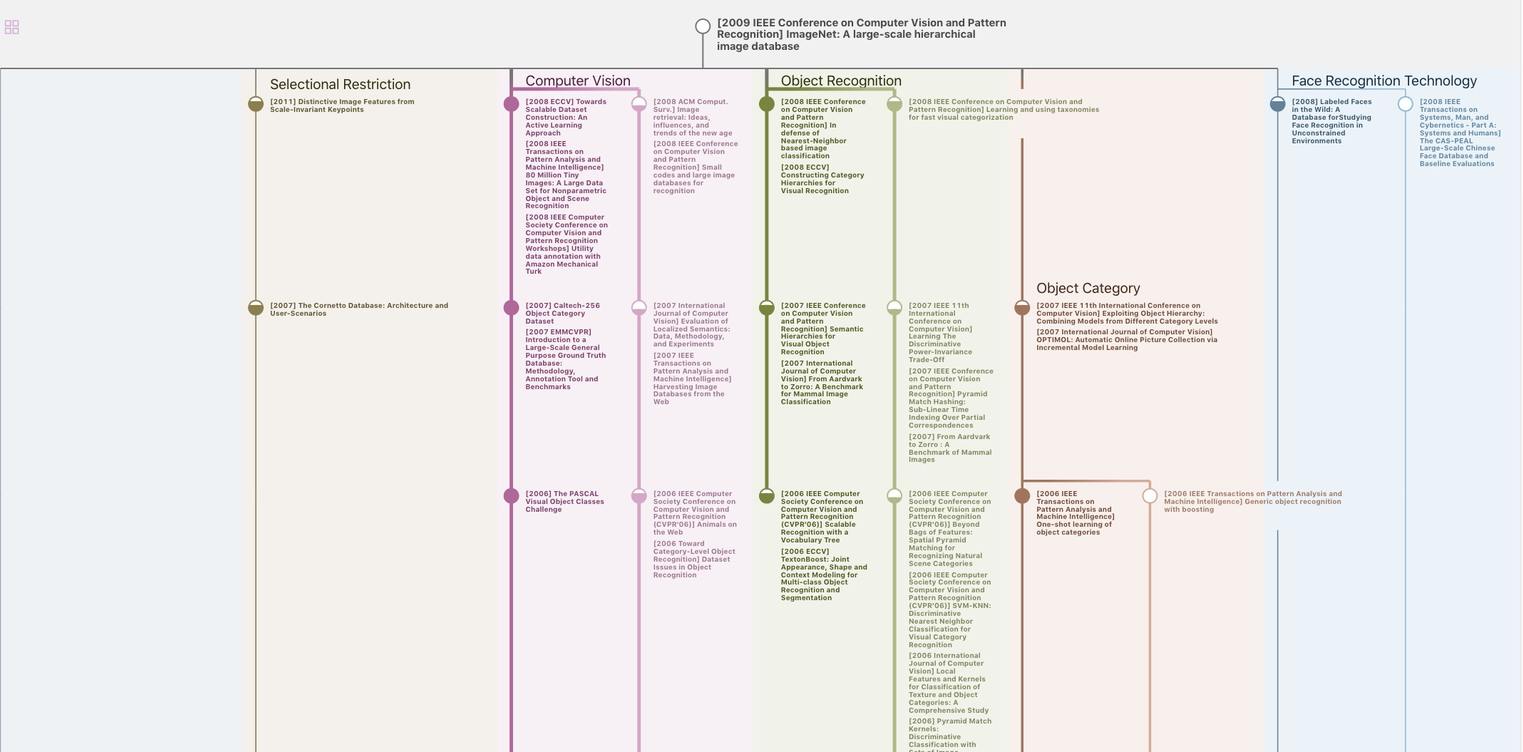
Generate MRT to find the research sequence of this paper
Chat Paper
Summary is being generated by the instructions you defined