Matched Pair Calibration for Ranking Fairness
CoRR(2023)
Abstract
We propose a test of fairness in score-based ranking systems called matched pair calibration. Our approach constructs a set of matched item pairs with minimal confounding differences between subgroups before computing an appropriate measure of ranking error over the set. The matching step ensures that we compare subgroup outcomes between identically scored items so that measured performance differences directly imply unfairness in subgroup-level exposures. We show how our approach generalizes the fairness intuitions of calibration from a binary classification setting to ranking and connect our approach to other proposals for ranking fairness measures. Moreover, our strategy shows how the logic of marginal outcome tests extends to cases where the analyst has access to model scores. Lastly, we provide an example of applying matched pair calibration to a real-word ranking data set to demonstrate its efficacy in detecting ranking bias.
MoreTranslated text
Key words
ranking fairness,pair,calibration
AI Read Science
Must-Reading Tree
Example
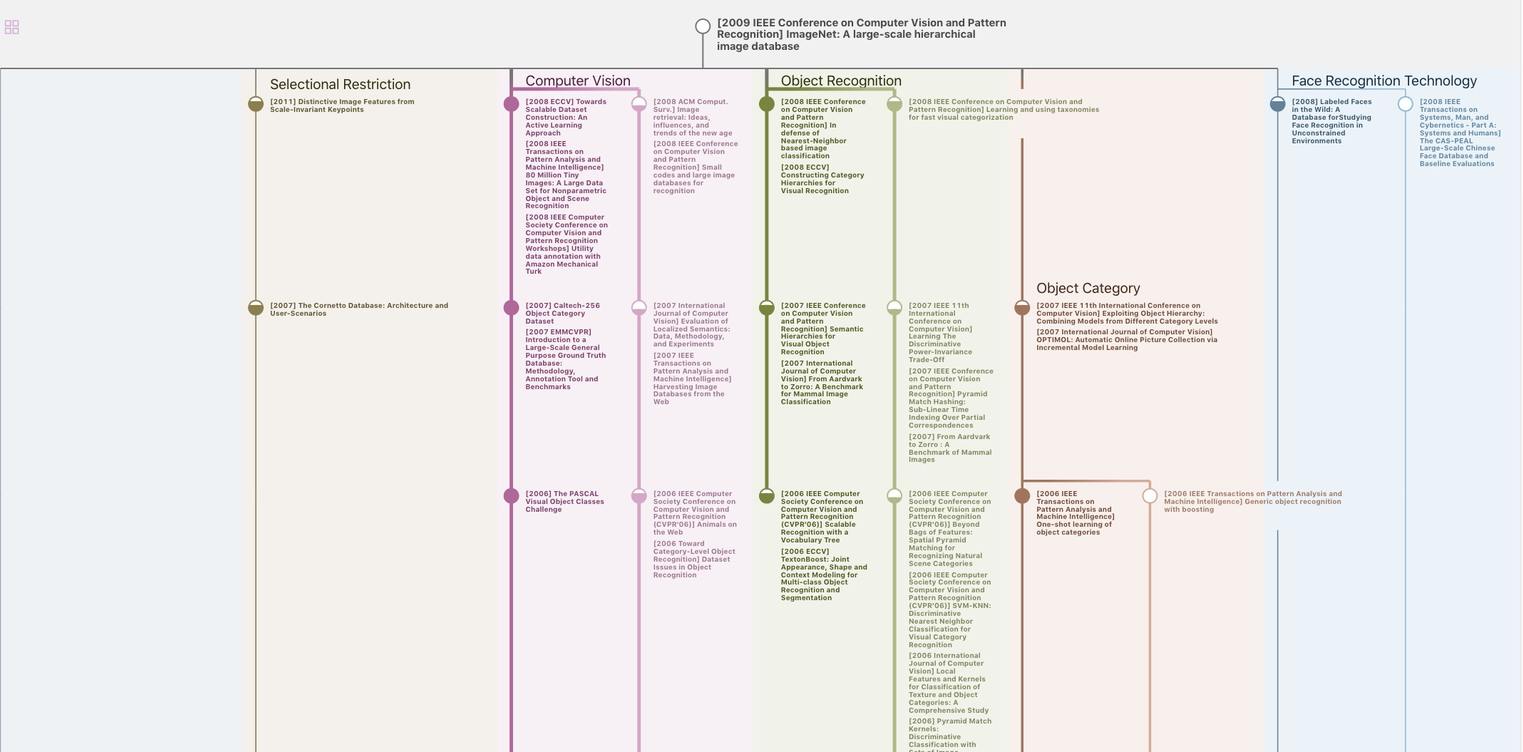
Generate MRT to find the research sequence of this paper
Chat Paper
Summary is being generated by the instructions you defined