Data-driven augmentation of a RANS turbulence model for transonic flow prediction
INTERNATIONAL JOURNAL OF NUMERICAL METHODS FOR HEAT & FLUID FLOW(2023)
摘要
Purpose This paper aims to improve Reynolds-averaged Navier Stokes (RANS) turbulence models using a data-driven approach based on machine learning (ML). A special focus is put on determining the optimal input features used for the ML model.Design/methodology/approach The field inversion and machine learning (FIML) approach is applied to the negative Spalart-Allmaras turbulence model for transonic flows over an airfoil where shock-induced separation occurs.Findings Optimal input features and an ML model are developed, which improve the existing negative Spalart-Allmaras turbulence model with respect to shock-induced flow separation.Originality/value A comprehensive workflow is demonstrated that yields insights on which input features and which ML model should be used in the context of the FIML approach
更多查看译文
关键词
rans turbulence model,flow,augmentation,data-driven
AI 理解论文
溯源树
样例
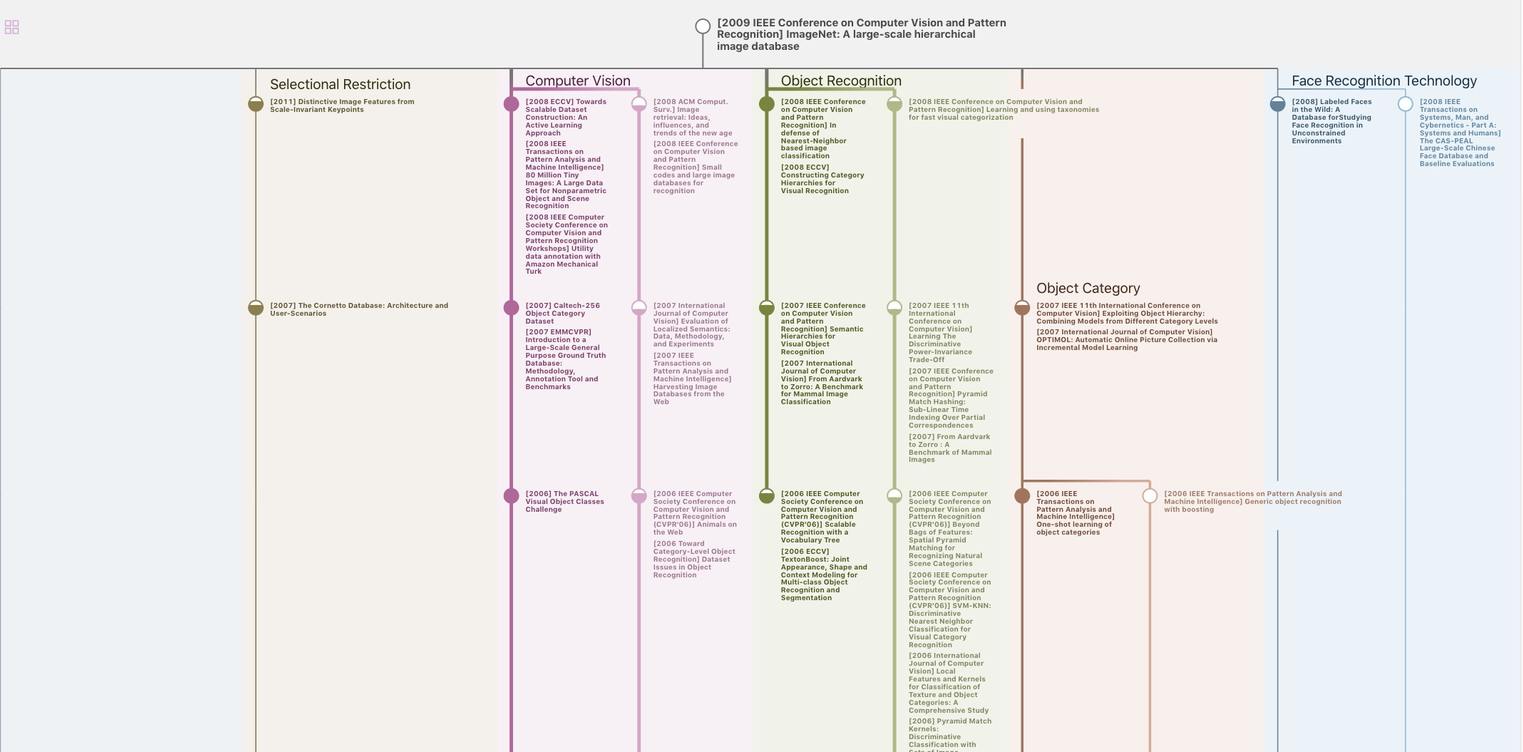
生成溯源树,研究论文发展脉络
Chat Paper
正在生成论文摘要